Articles
All articles
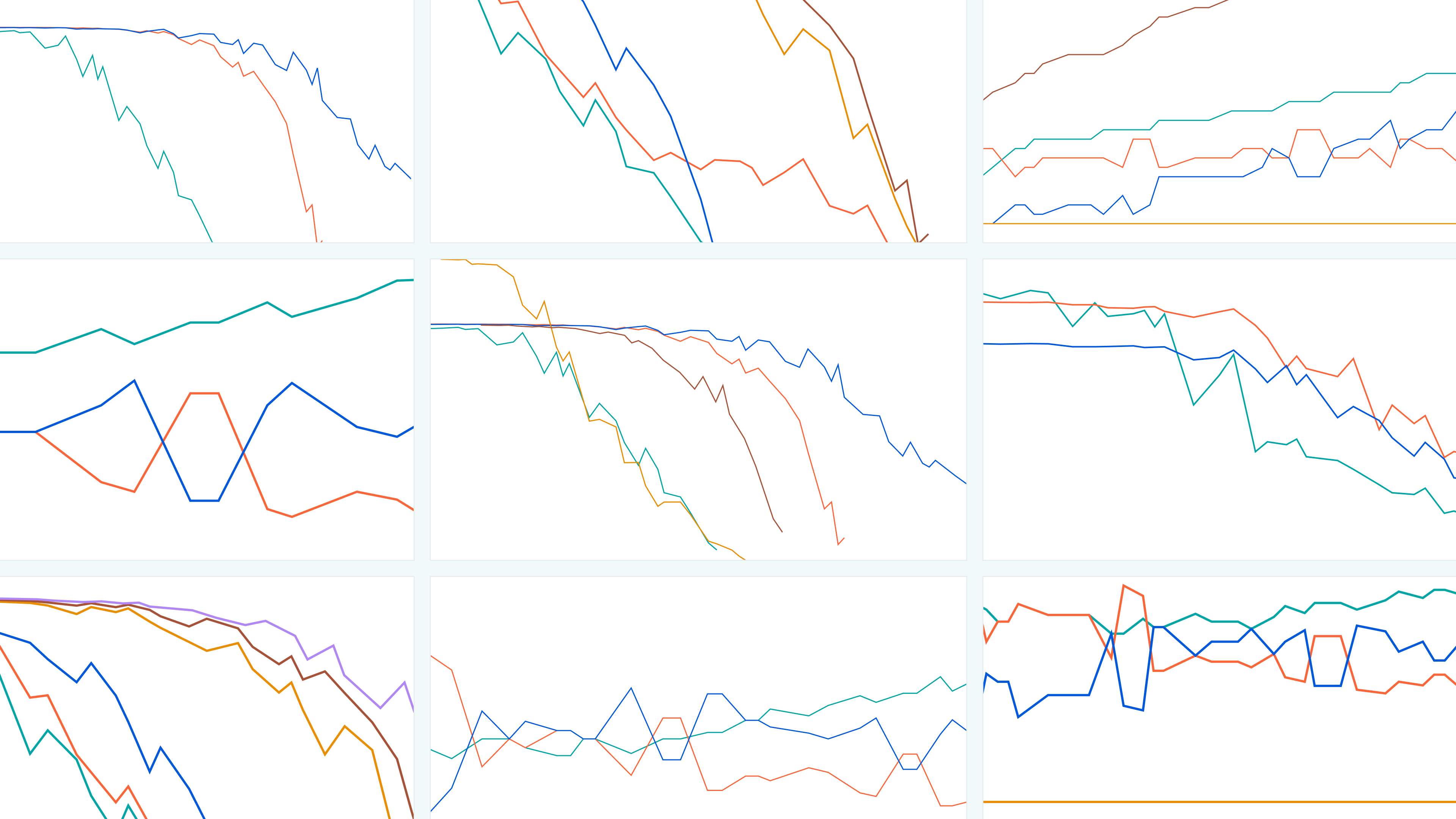
announcement
· 6 min read
Introducing the Distributed Training Interactive Simulator
We introduce and walk you through an interactive tool that simulates distributed training runs of large language models under ideal conditions.
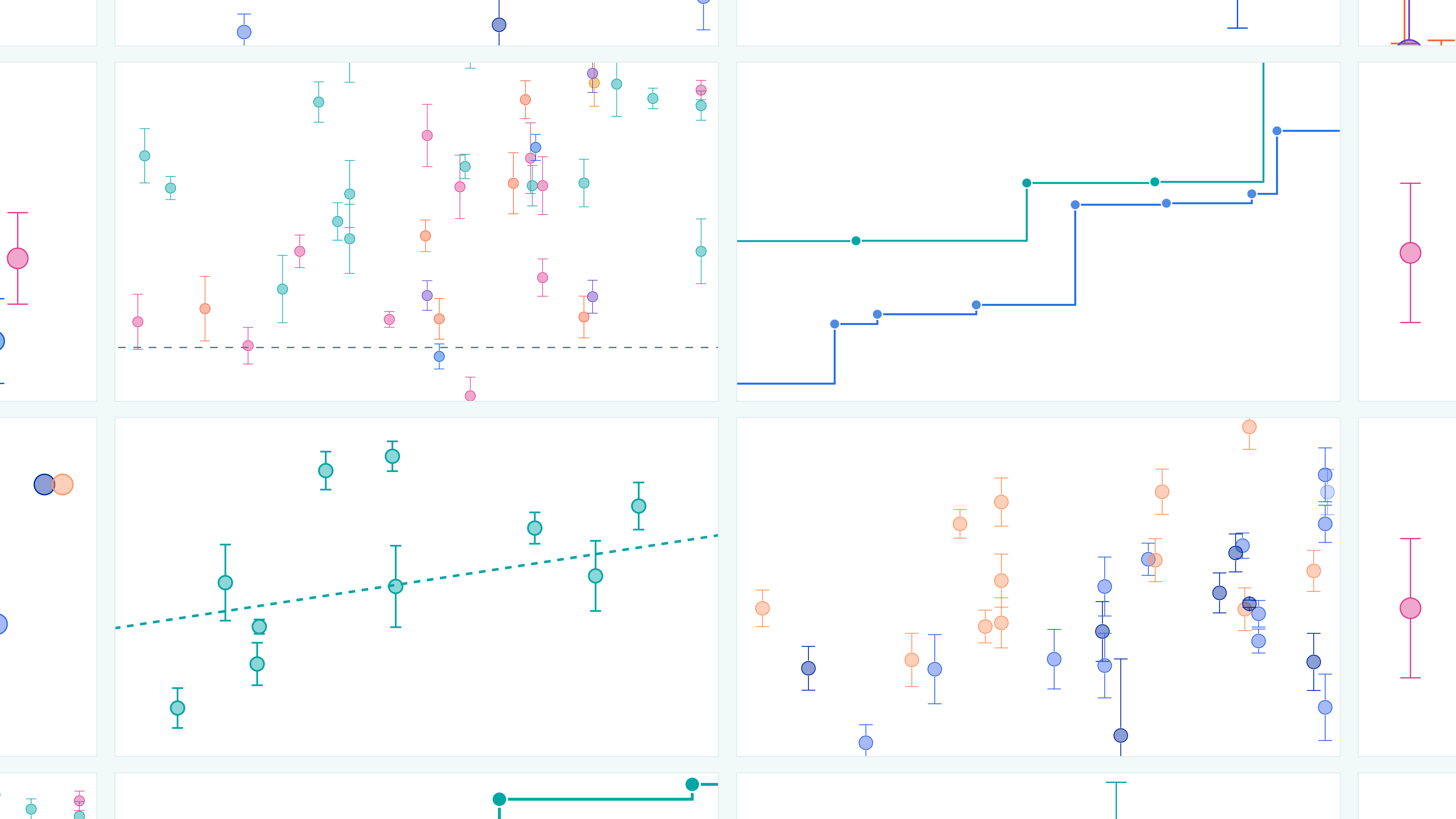
announcement
· 2 min read
Introducing Epoch AI's AI Benchmarking Hub
We are launching the AI Benchmarking Hub: a platform presenting our evaluations of leading models on challenging benchmarks, with analysis of trends in AI capabilities.
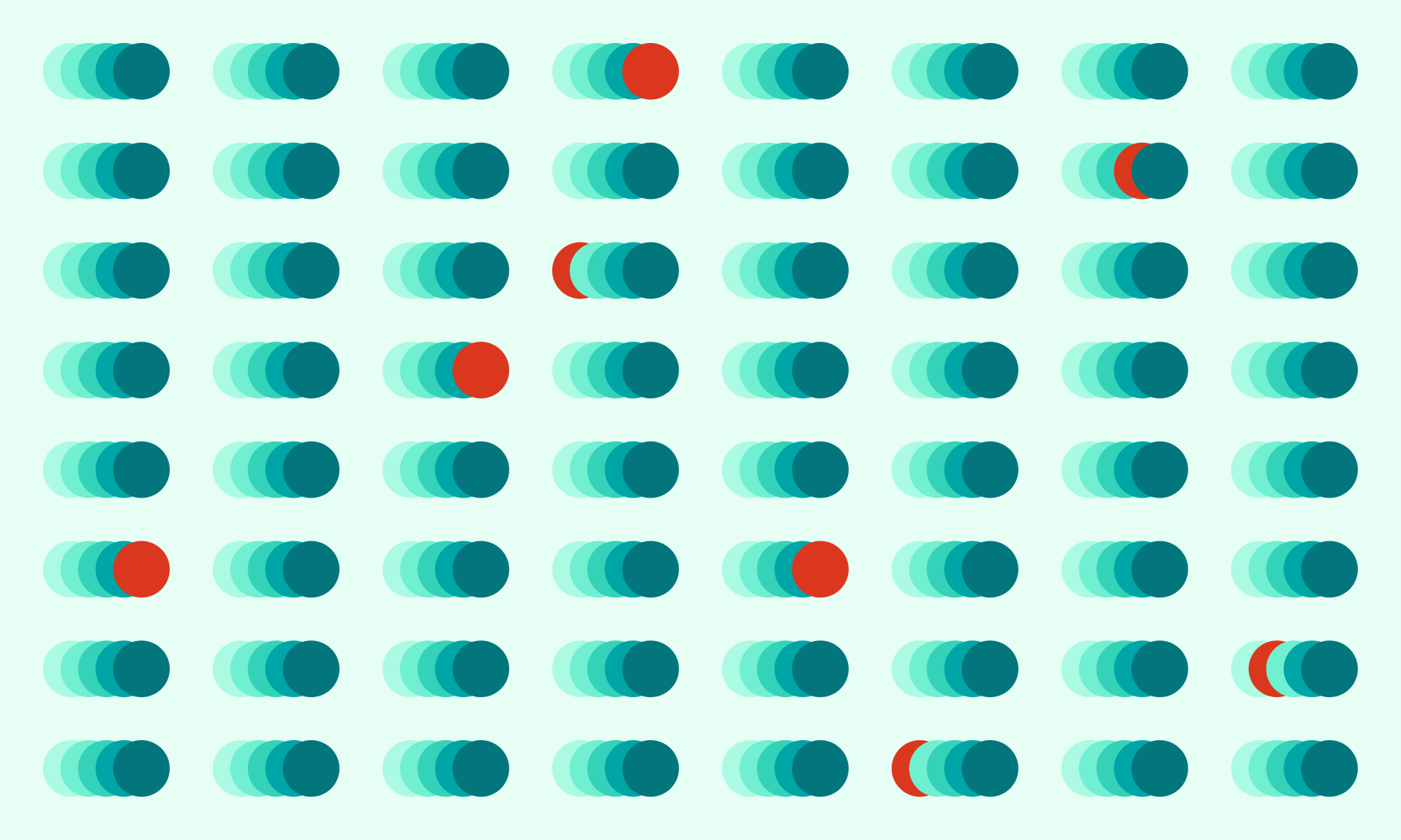
report
· 15 min read
Hardware Failures Won’t Limit AI Scaling
Hardware failures won't limit AI training scale - GPU memory checkpointing enables training with millions of GPUs despite failures.
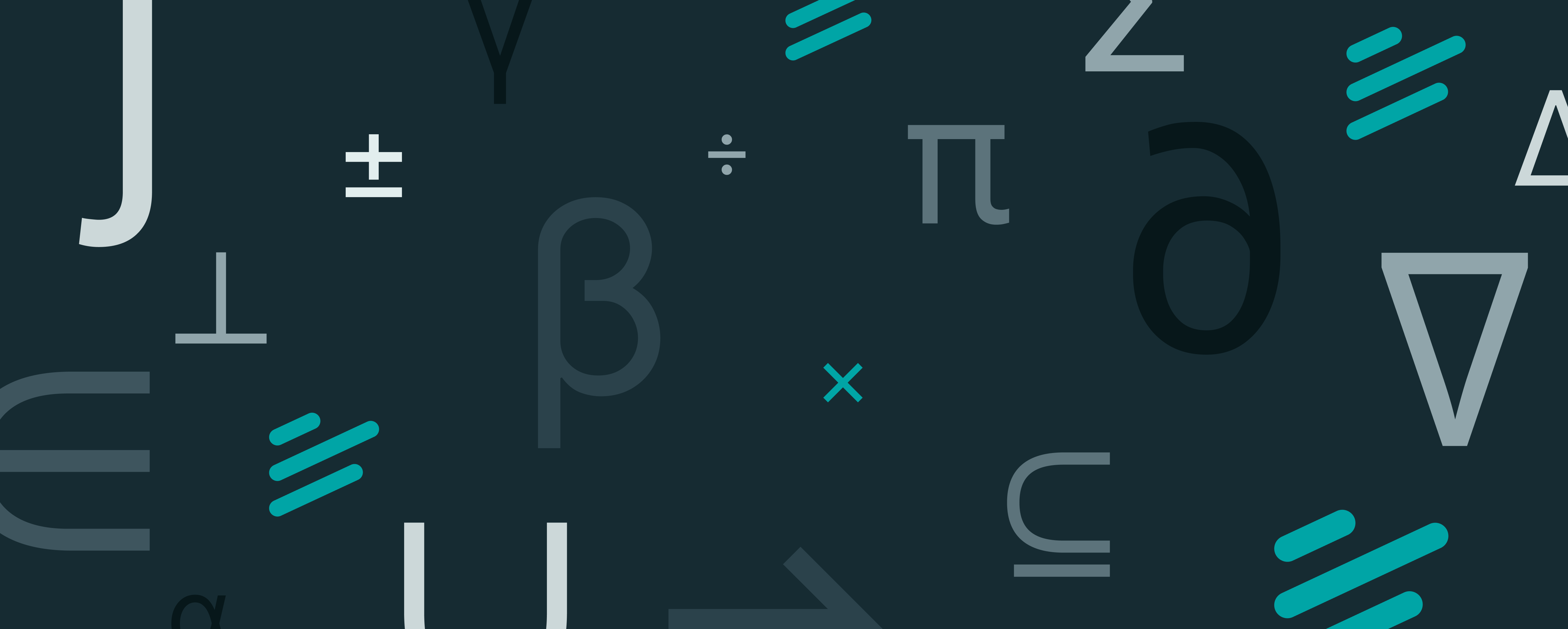
announcement
· 6 min read
FrontierMath: A Benchmark for Evaluating Advanced Mathematical Reasoning in AI
FrontierMath: a new benchmark of expert-level math problems designed to measure AI's mathematical abilities. See how leading AI models perform against the collective mathematics community.
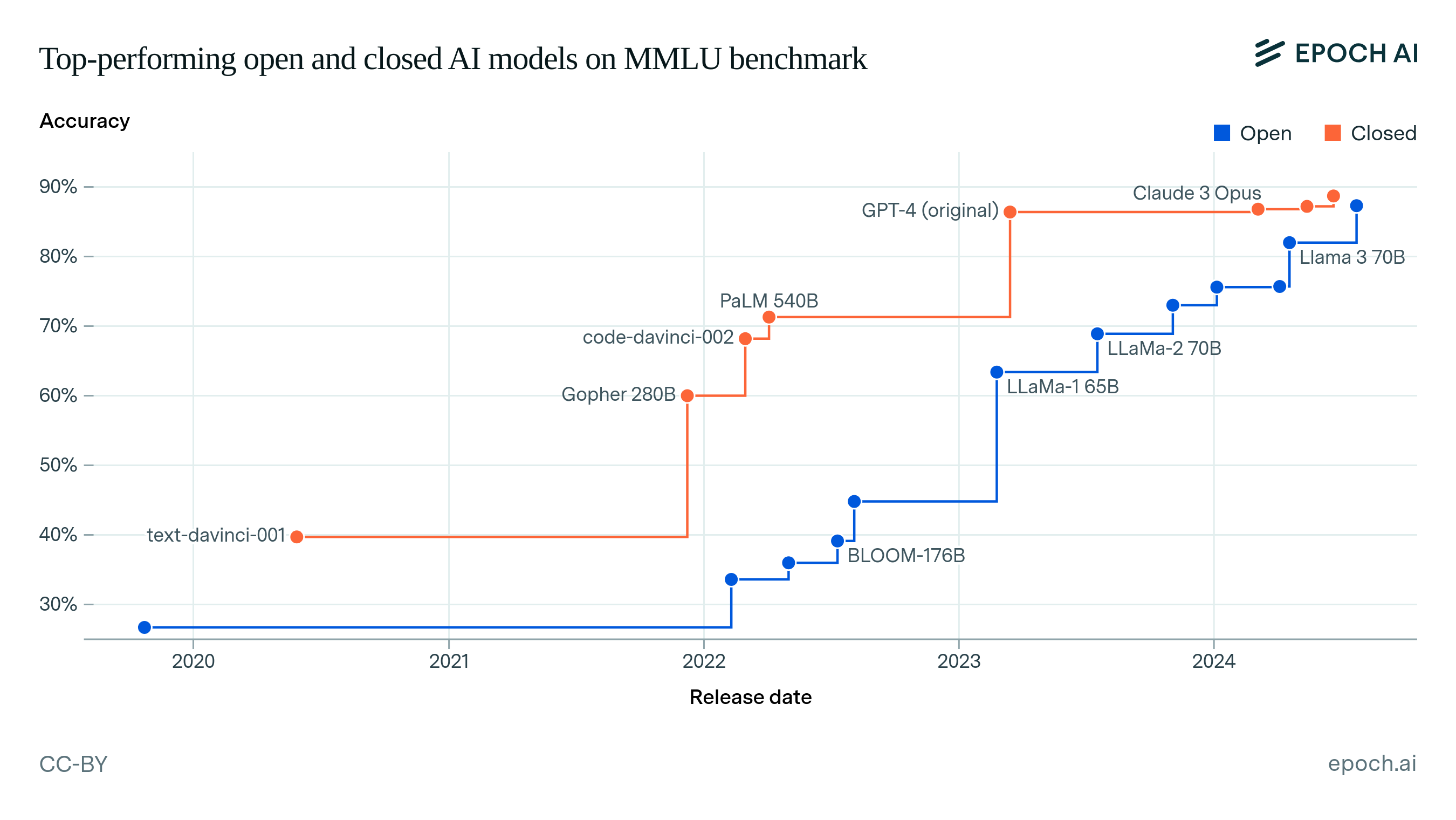
report
· 37 min read
How Far Behind Are Open Models?
Analysis of open vs. closed AI models reveals the best open model today matches closed models in performance and training compute, but with a one-year lag.
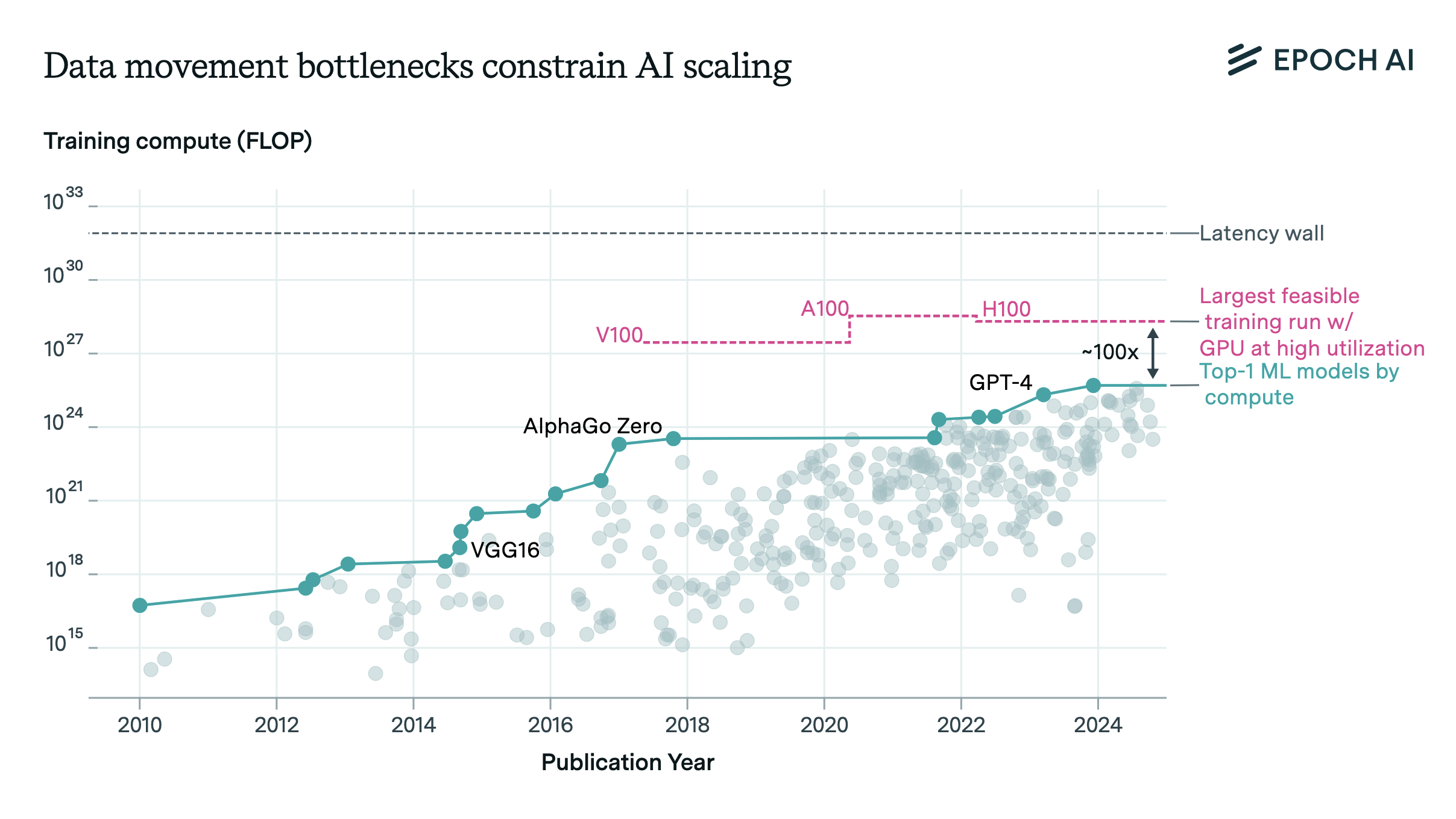
paper
· 14 min read
Data Movement Bottlenecks to Large-Scale Model Training: Scaling Past 1e28 FLOP
Data movement bottlenecks limit LLM scaling beyond 2e28 FLOP, with a "latency wall" at 2e31 FLOP. We may hit these in ~3 years. Aggressive batch size scaling could potentially overcome these limits.
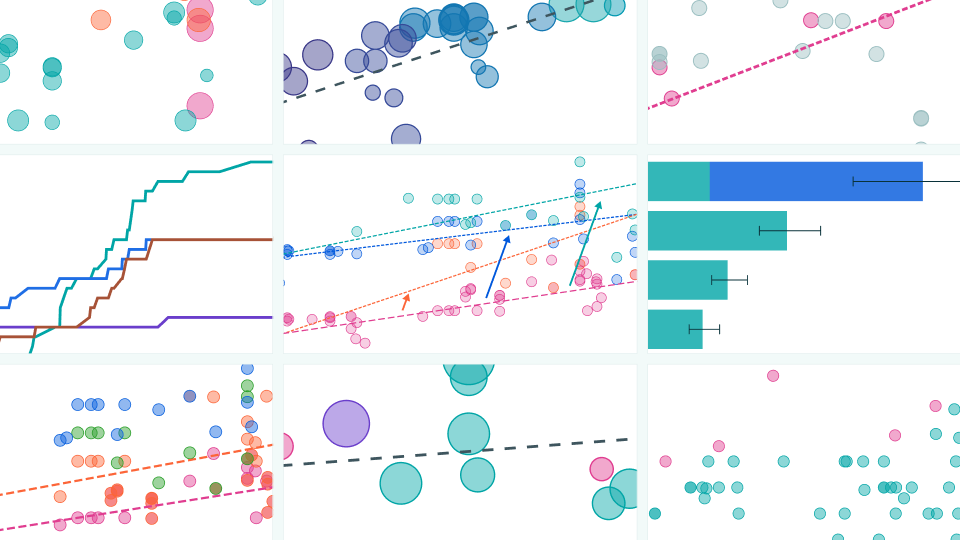
announcement
· 1 min read
Introducing Epoch AI’s Machine Learning Hardware Database
Our new database covers hardware used to train AI models, featuring over 100 accelerators (GPUs and TPUs) across the deep learning era.
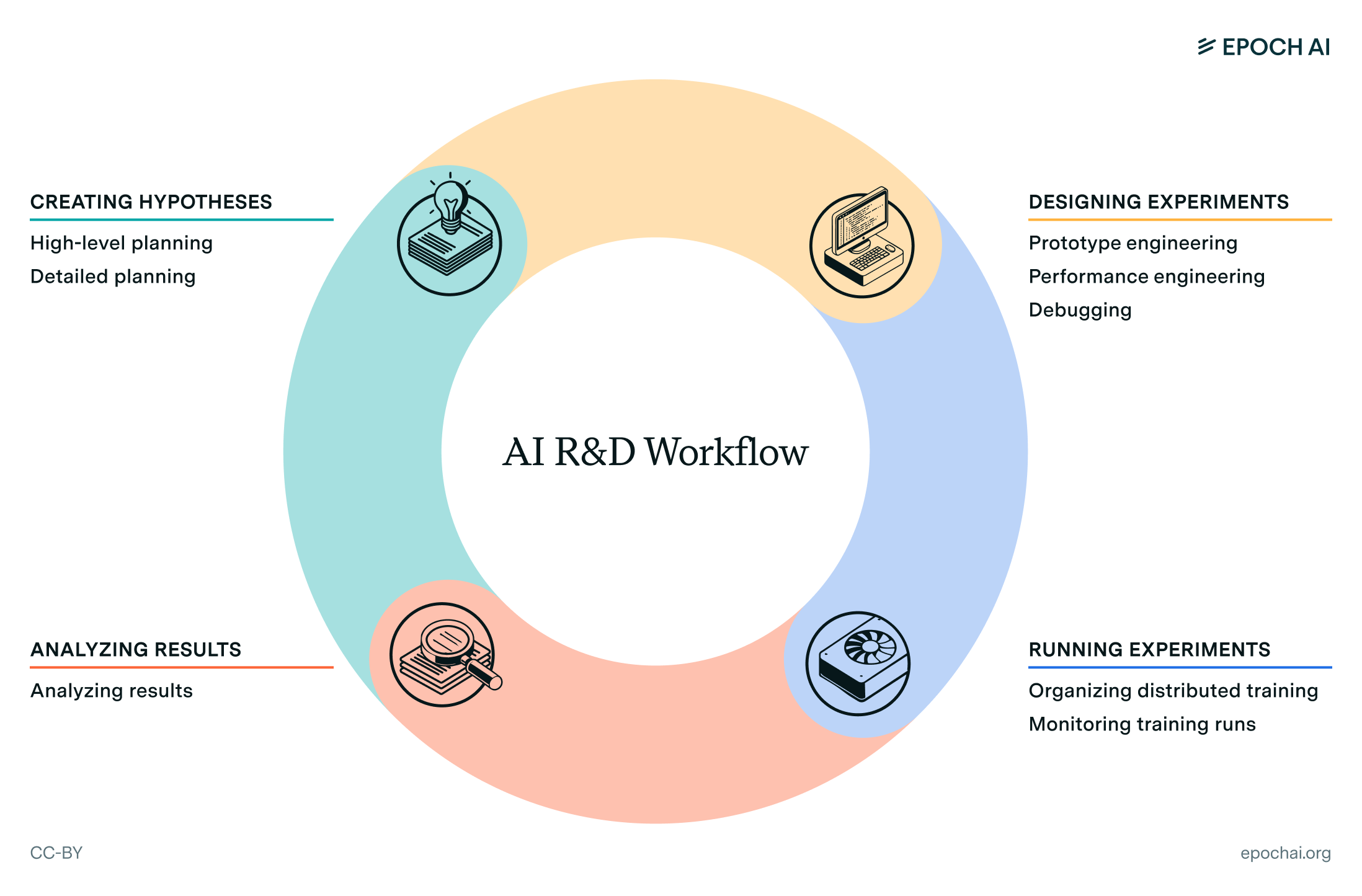
report
· 10 min read
Interviewing AI researchers on automation of AI R&D
AI could speed up AI R&D, especially in coding and debugging. We explore predictions on automation and researchers' suggestions for AI R&D evaluations.
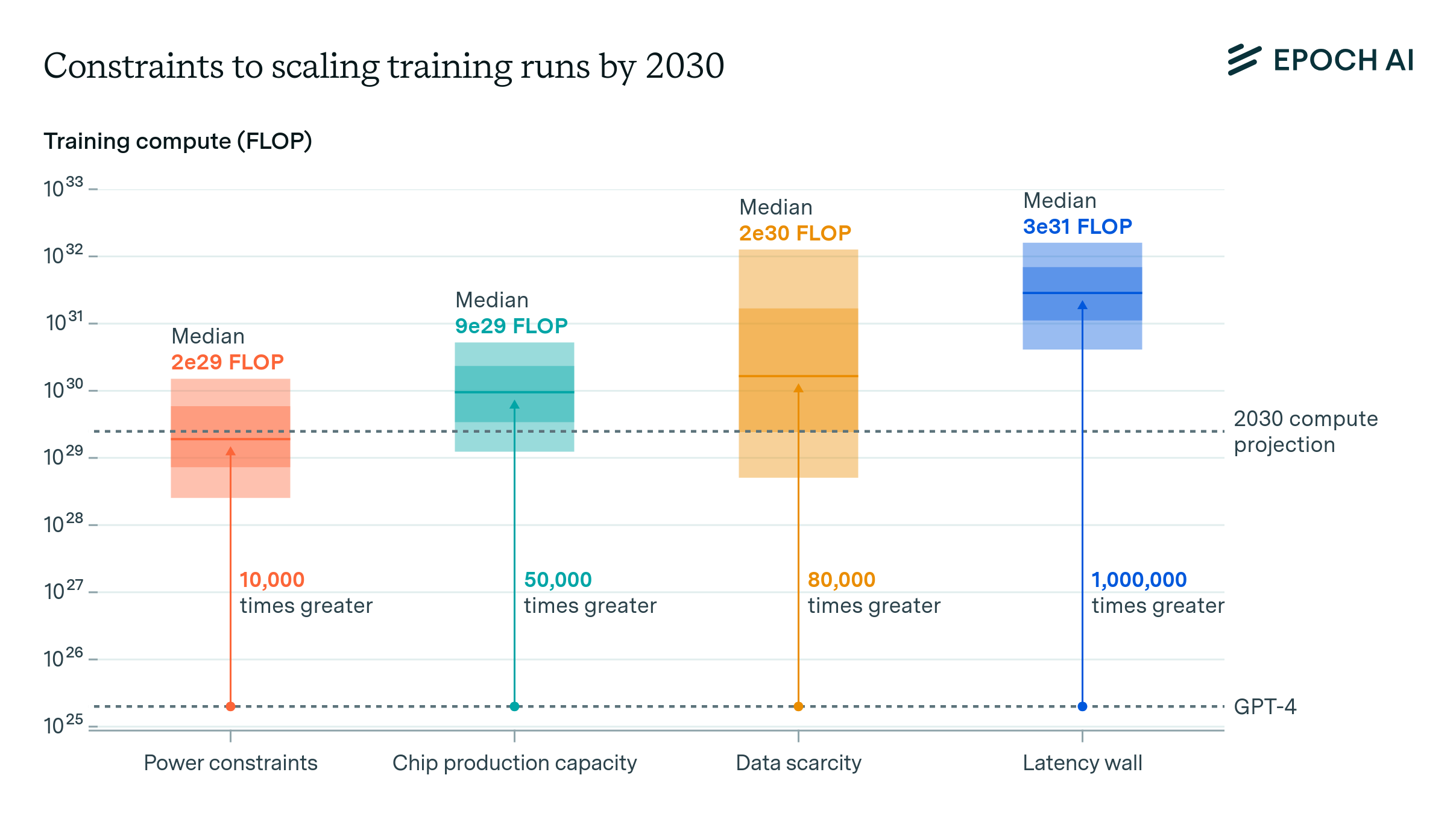
report
· 83 min read
Can AI Scaling Continue Through 2030?
We investigate four constraints to scaling AI training: power, chip manufacturing, data, and latency. We predict 2e29 FLOP runs will be feasible by 2030.
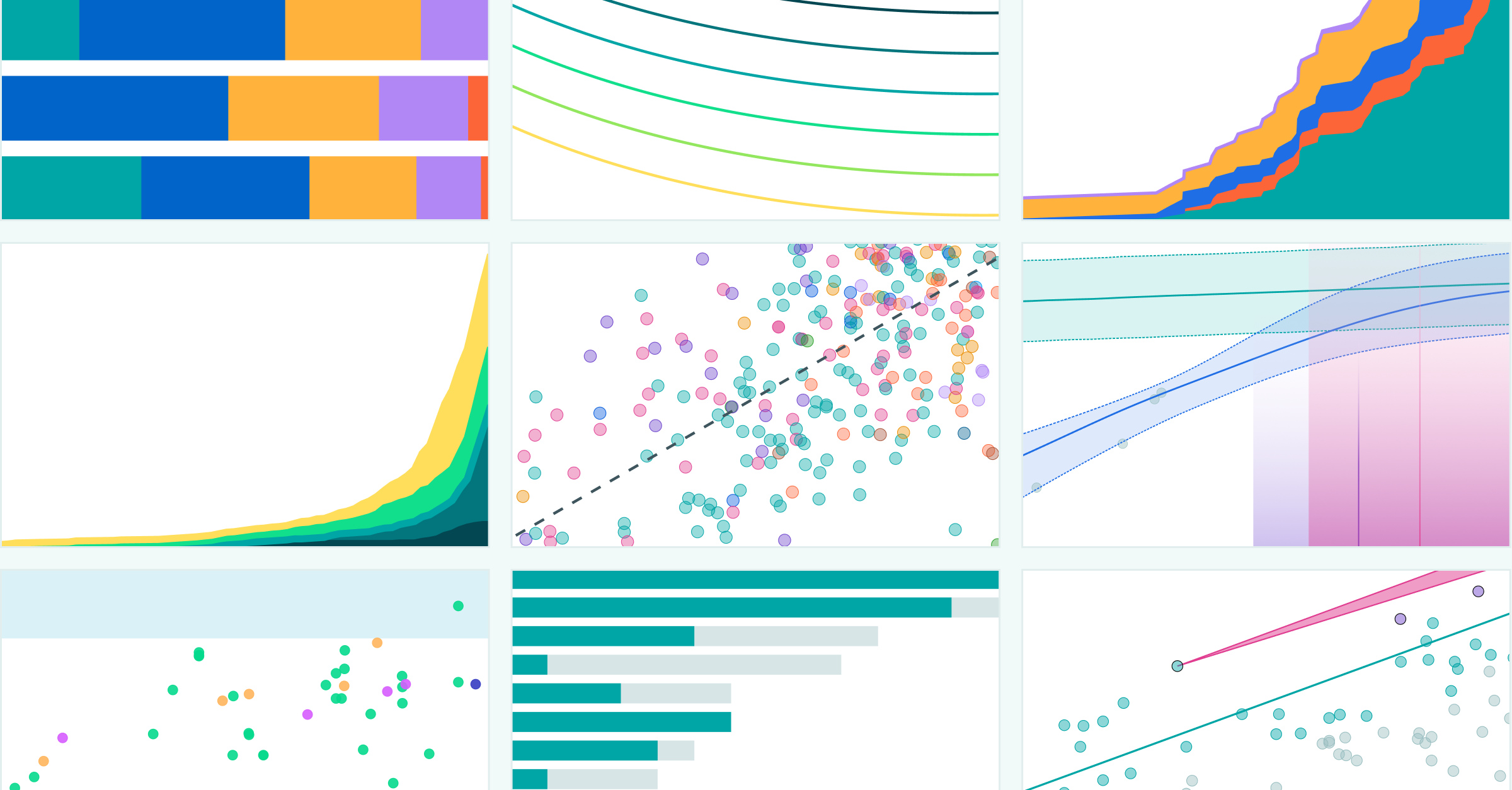
announcement
· 1 min read
Announcing Epoch AI’s Data Hub
We're launching a hub for data and visualizations, featuring our databases on notable and large-scale AI models for users and researchers.
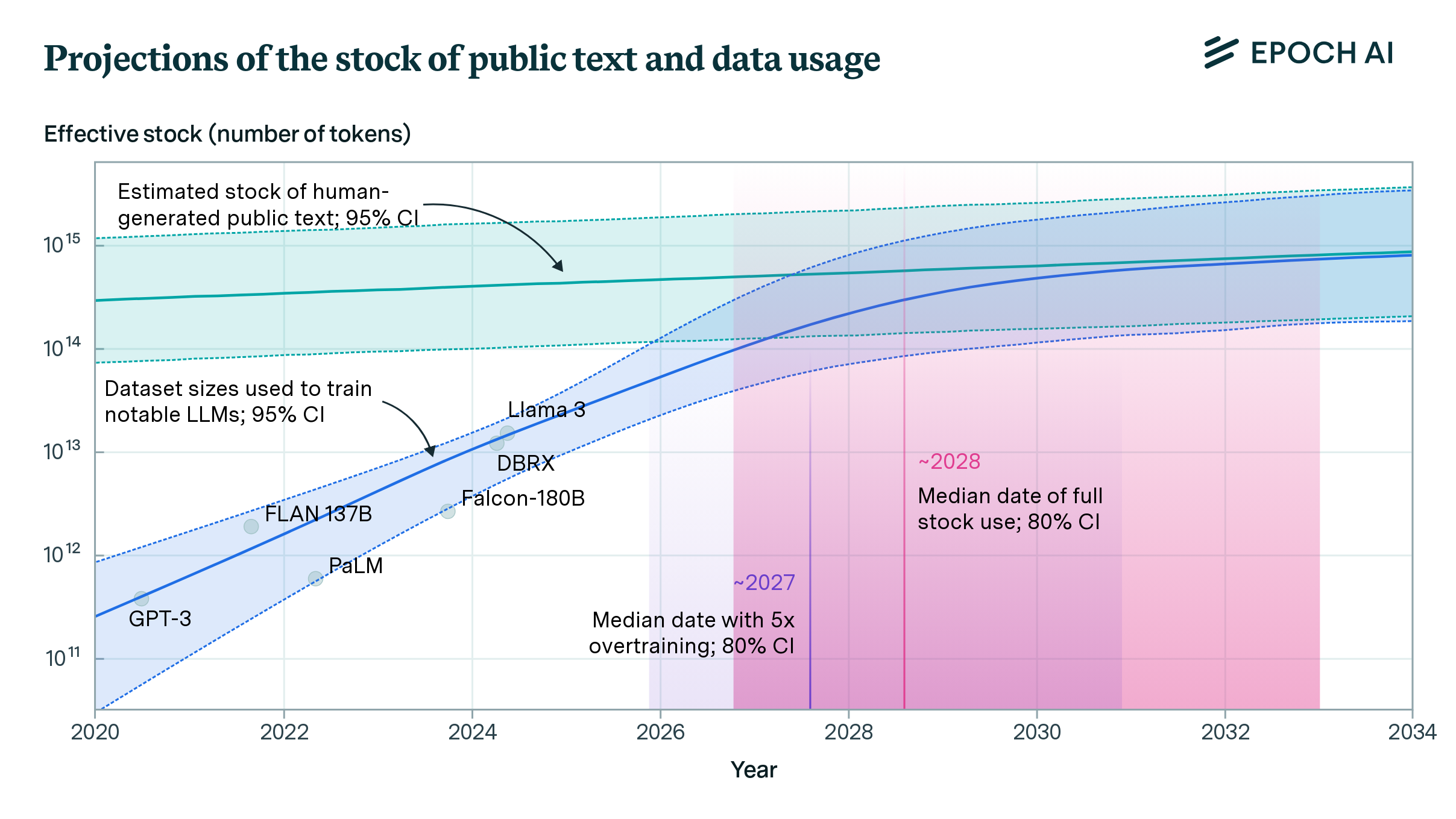
paper
· 6 min read
Will We Run Out of Data? Limits of LLM Scaling Based on Human-Generated Data
If trends continue, language models will fully utilize the stock of human-generated public text between 2026 and 2032.
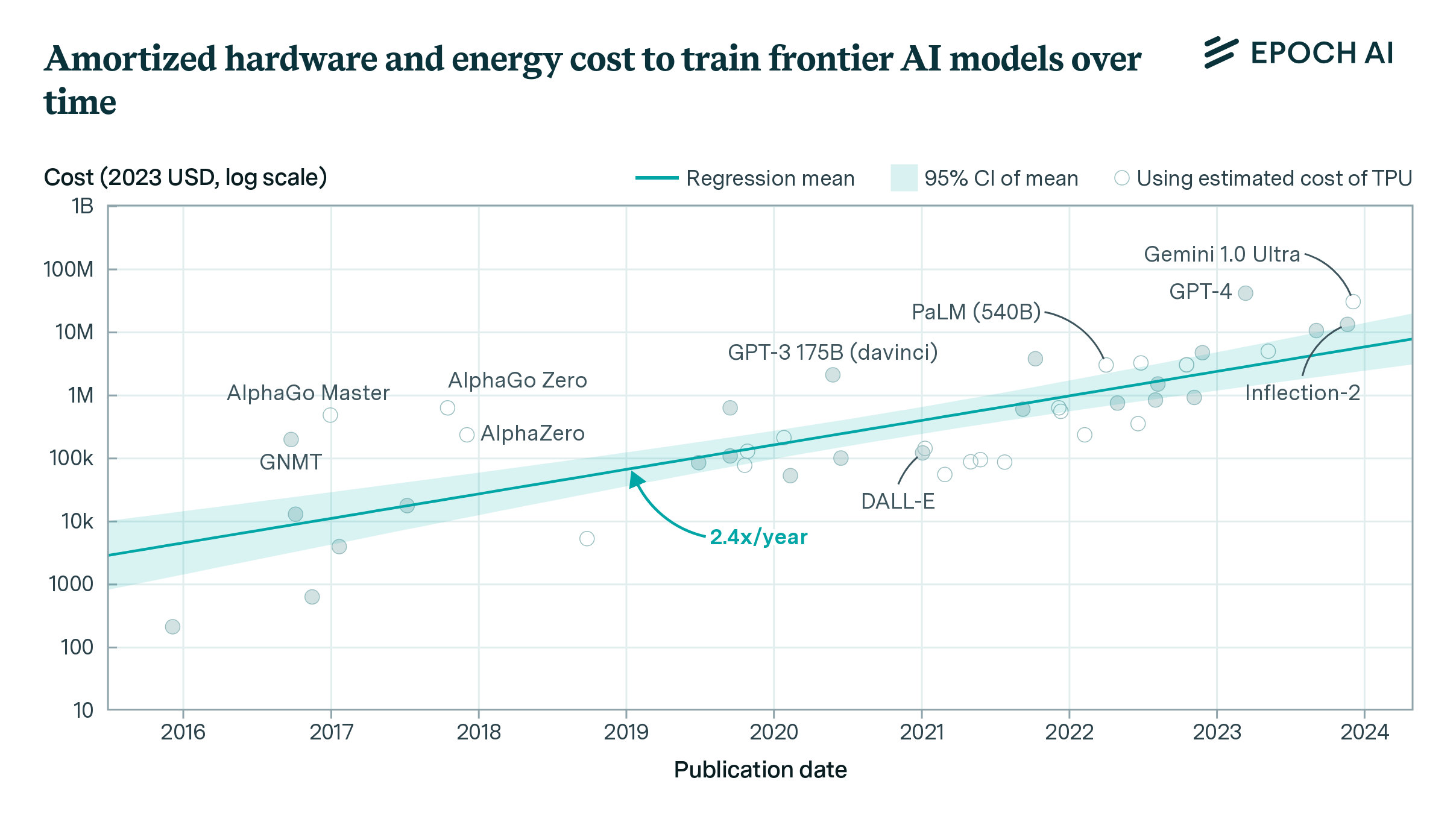
paper
· 4 min read
How Much Does It Cost to Train Frontier AI Models?
The cost of training top AI models has grown 2-3x annually for the past eight years. By 2027, the largest models could cost over a billion dollars.
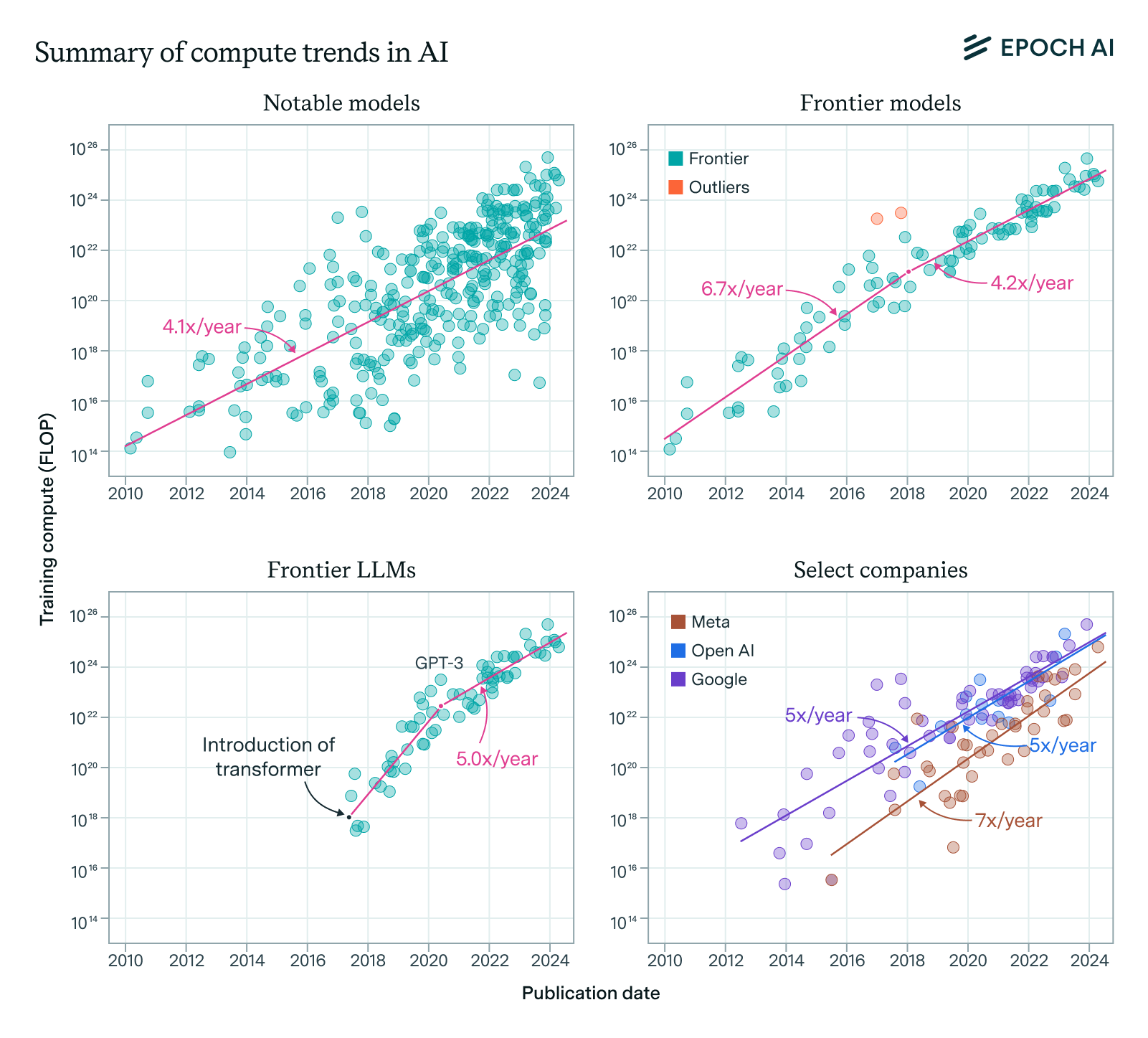
report
· 20 min read
Training Compute of Frontier AI Models Grows by 4-5x per Year
Our expanded AI model database shows that training compute grew 4-5x/year from 2010 to 2024, with similar trends in frontier and large language models.
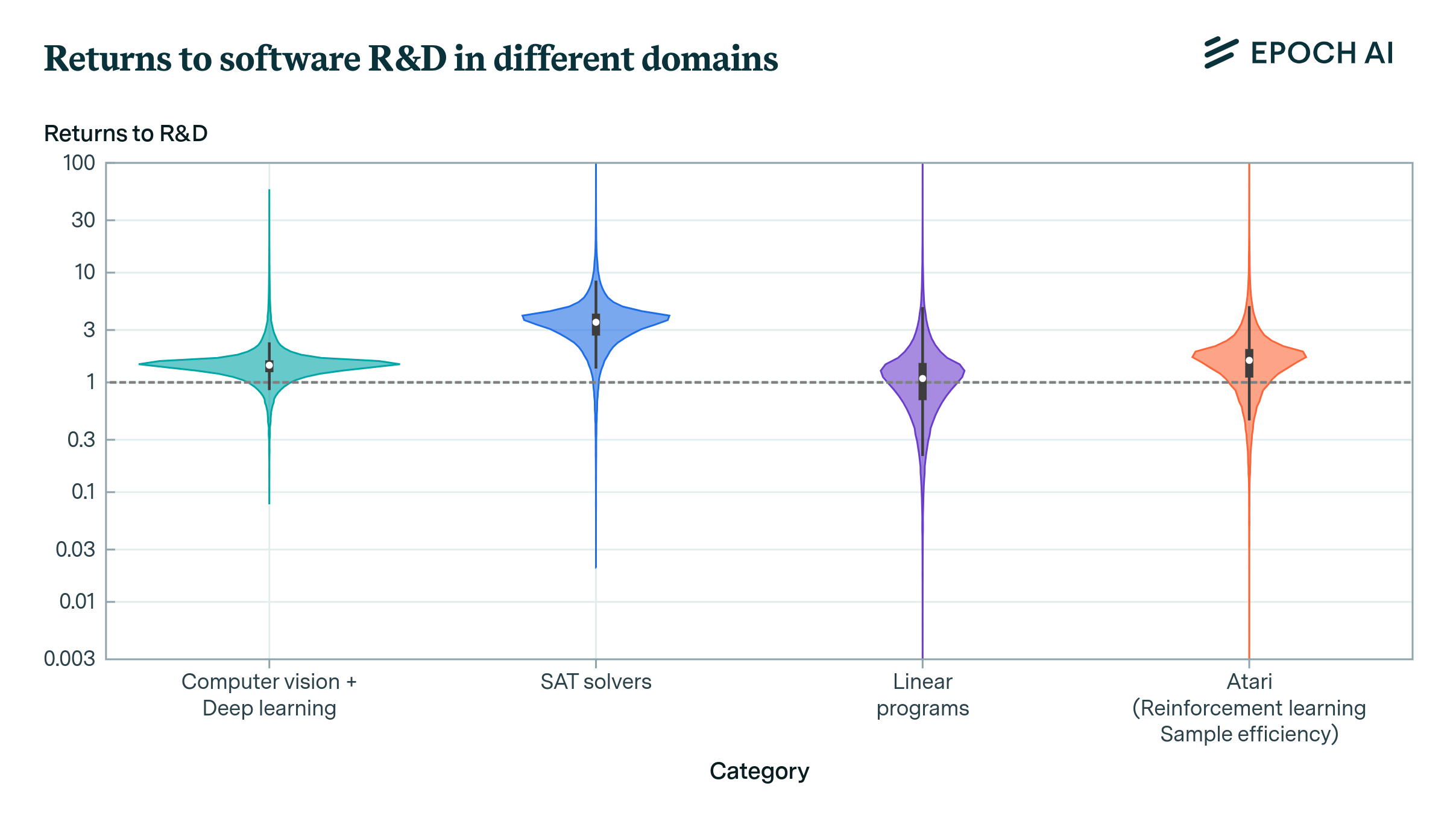
paper
· 10 min read
Do the Returns to Software R&D Point Towards a Singularity?
Returns to R&D are key in growth dynamics and AI development. Our paper introduces new empirical techniques to estimate this vital parameter.
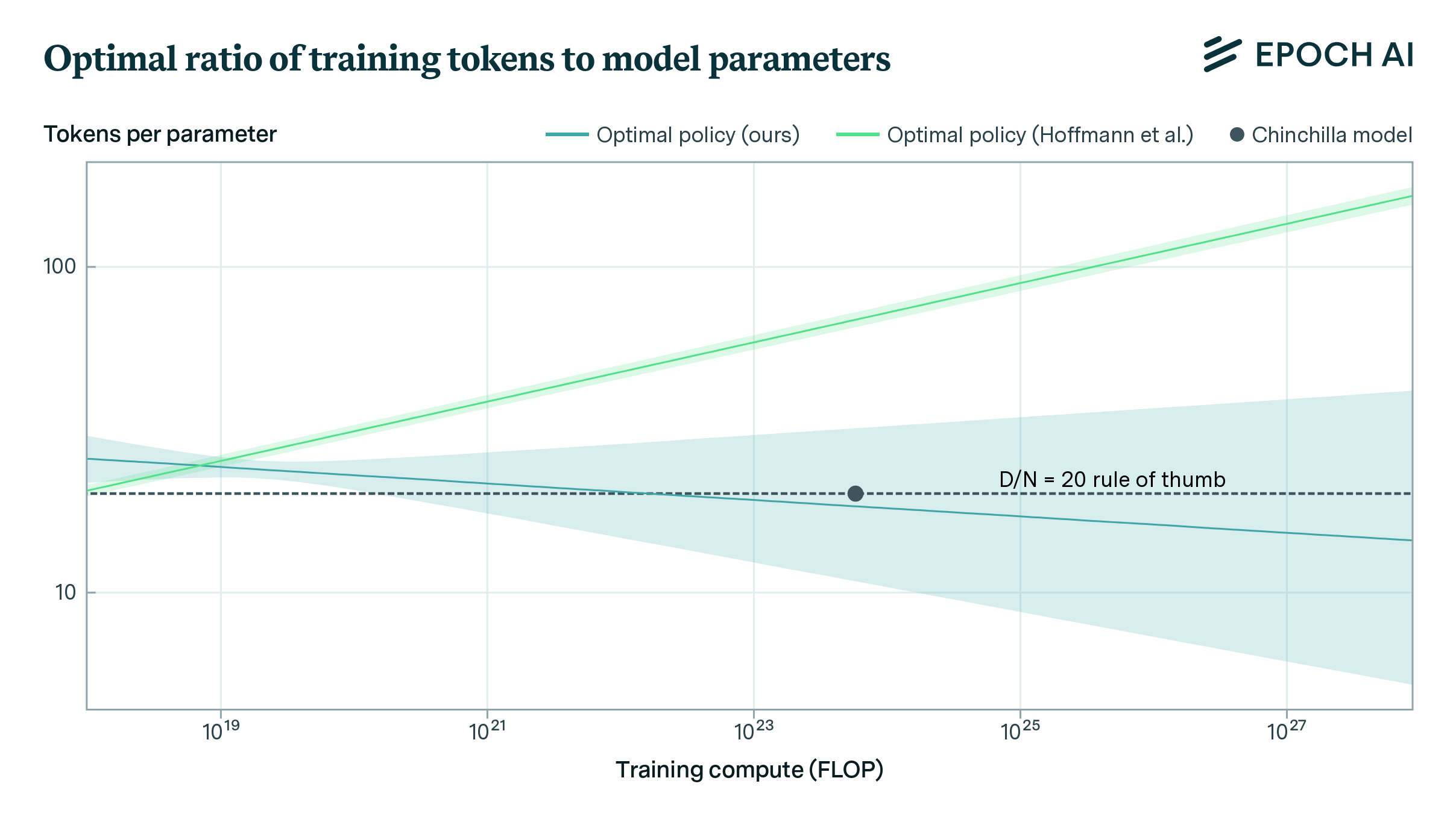
paper
· 4 min read
Chinchilla Scaling: A Replication Attempt
We replicate Hoffmann et al.’s parametric scaling law estimates, finding issues and providing better-fitting estimates that align with their other methods.
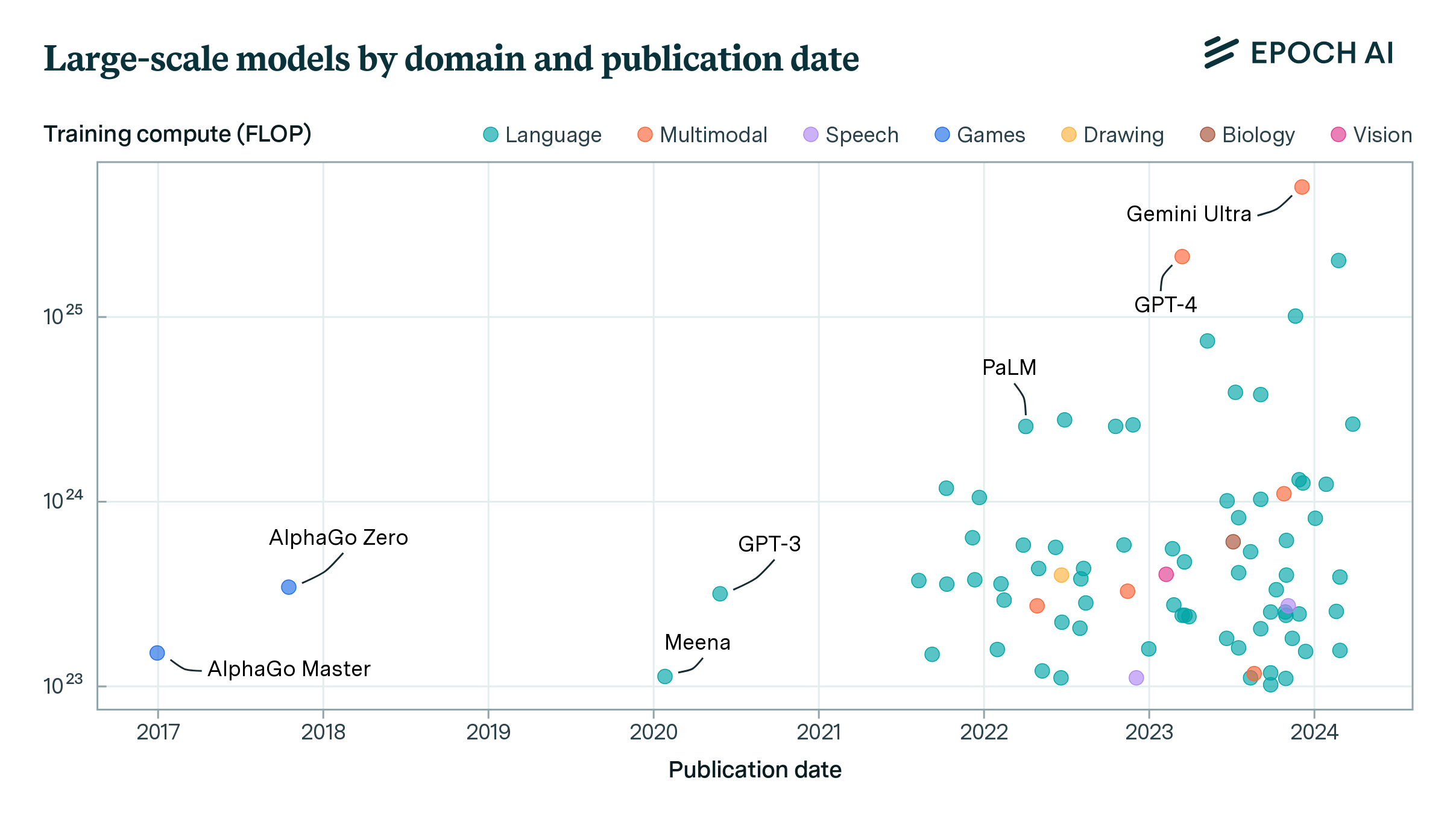
report
· 16 min read
Tracking Large-Scale AI Models
We present a dataset of 81 large-scale models, from AlphaGo to Gemini, developed across 18 countries, at the leading edge of scale and capabilities.
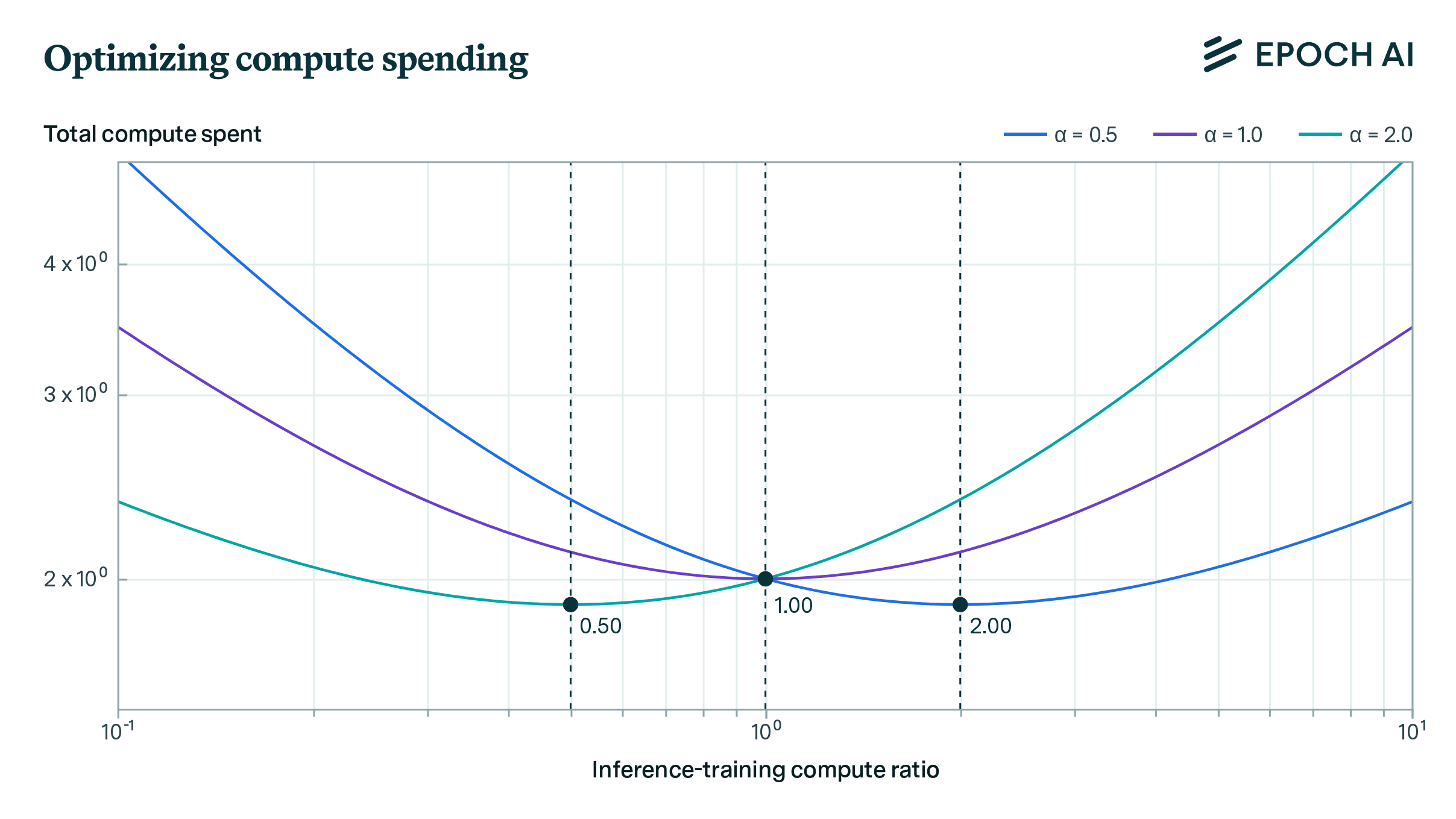
report
· 9 min read
Optimally Allocating Compute Between Inference and Training
AI labs should spend comparable resources on training and inference, assuming they can flexibly balance compute between the two to maintain performance.
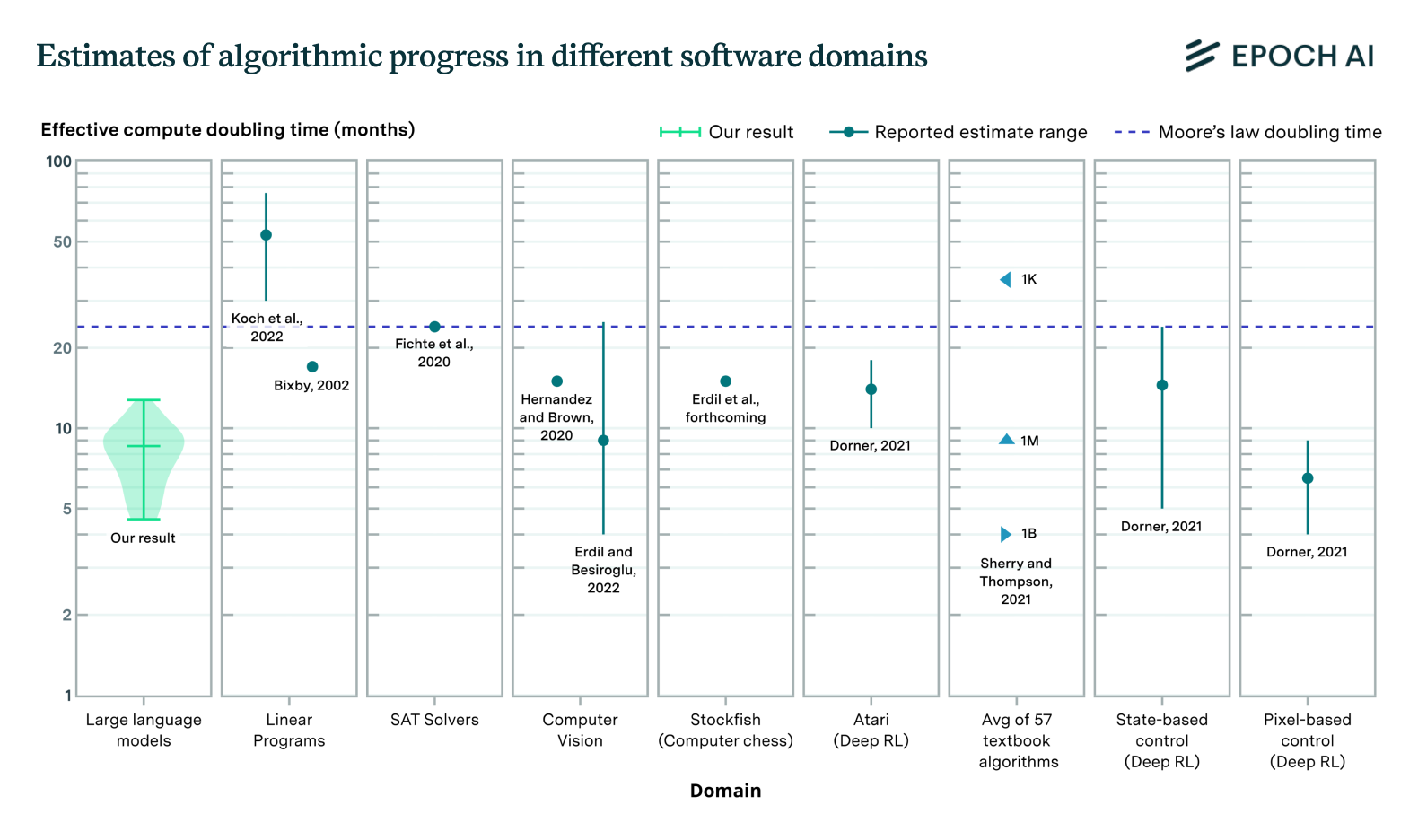
paper
· 3 min read
Algorithmic Progress in Language Models
Progress in language model performance outpaces expectations, occurring at a pace equivalent to doubling computational power every 5 to 14 months.
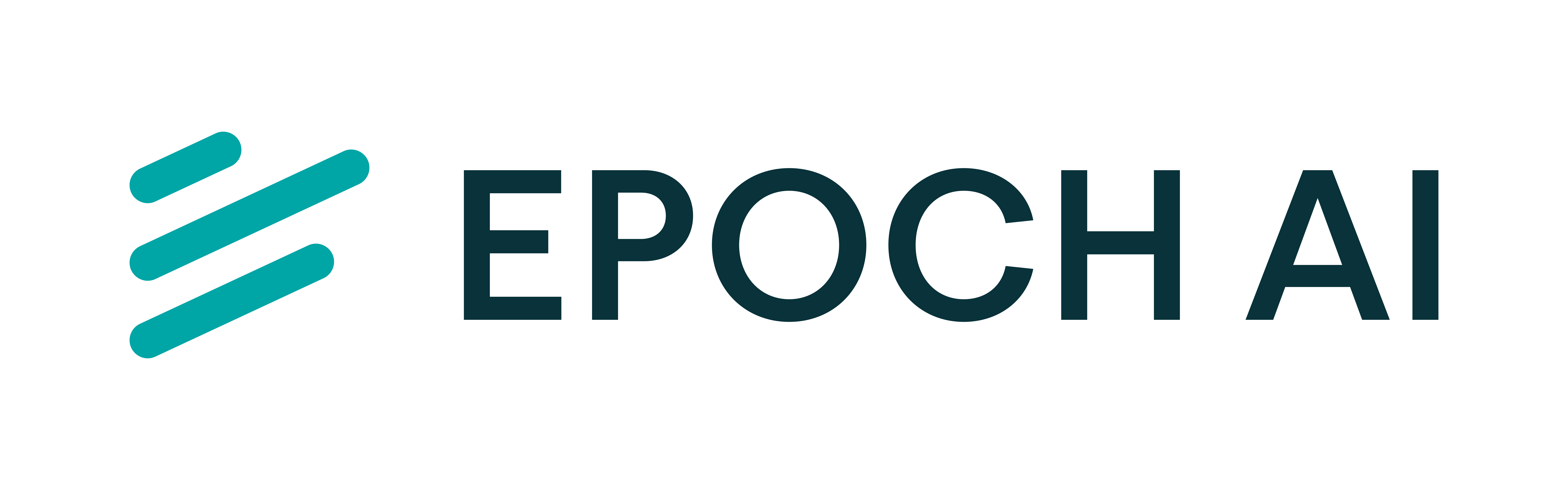
announcement
· 10 min read
Epoch AI Impact Assessment 2023
In 2023, Epoch published nearly 20 reports on AI, added hundreds of models to our database, helped with government policies, and raised over $7 million.
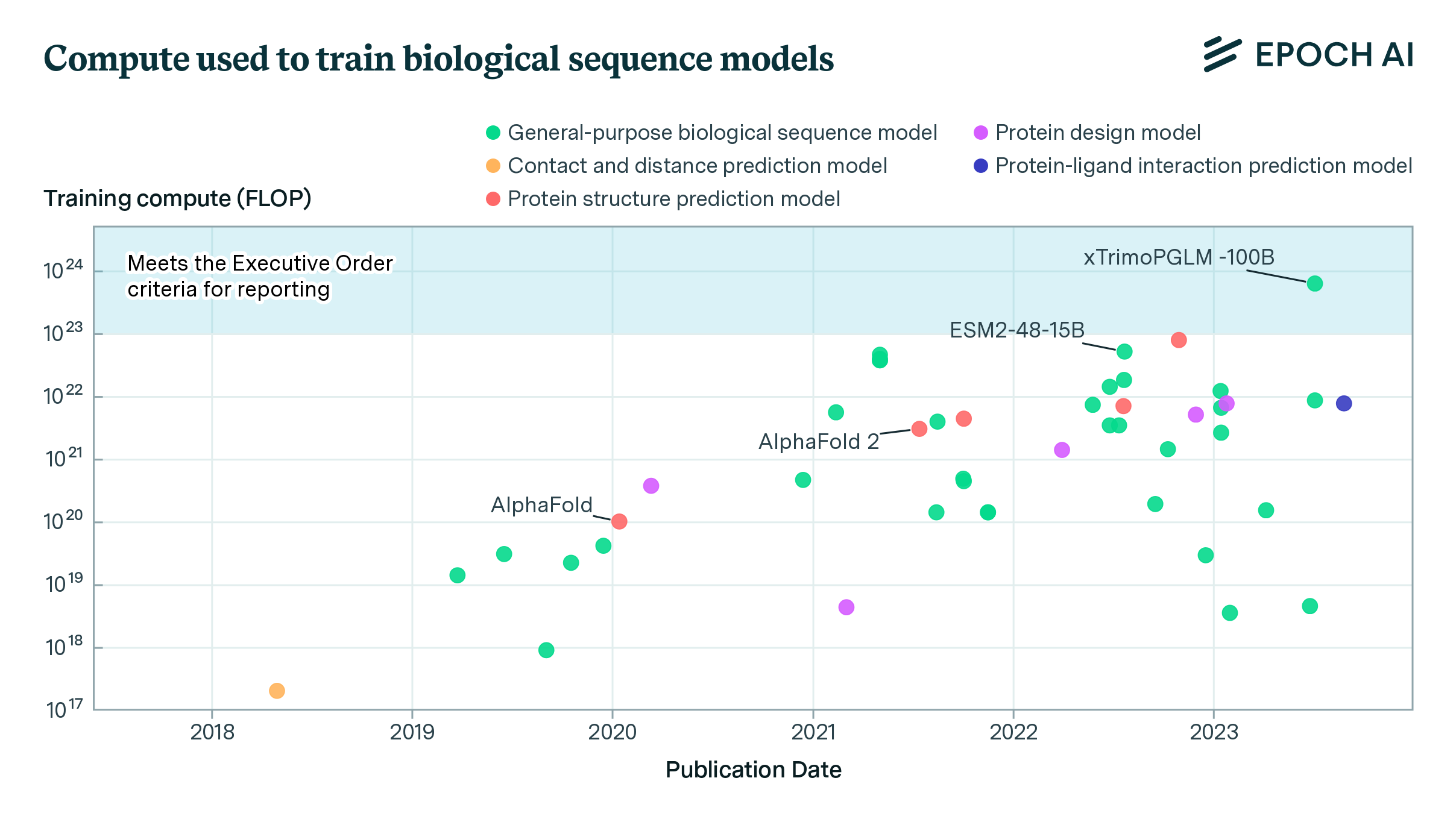
report
· 23 min read
Biological Sequence Models in the Context of the AI Directives
Our expanded database now includes biological sequence models, highlighting potential regulatory gaps and the growth of training compute in these models.
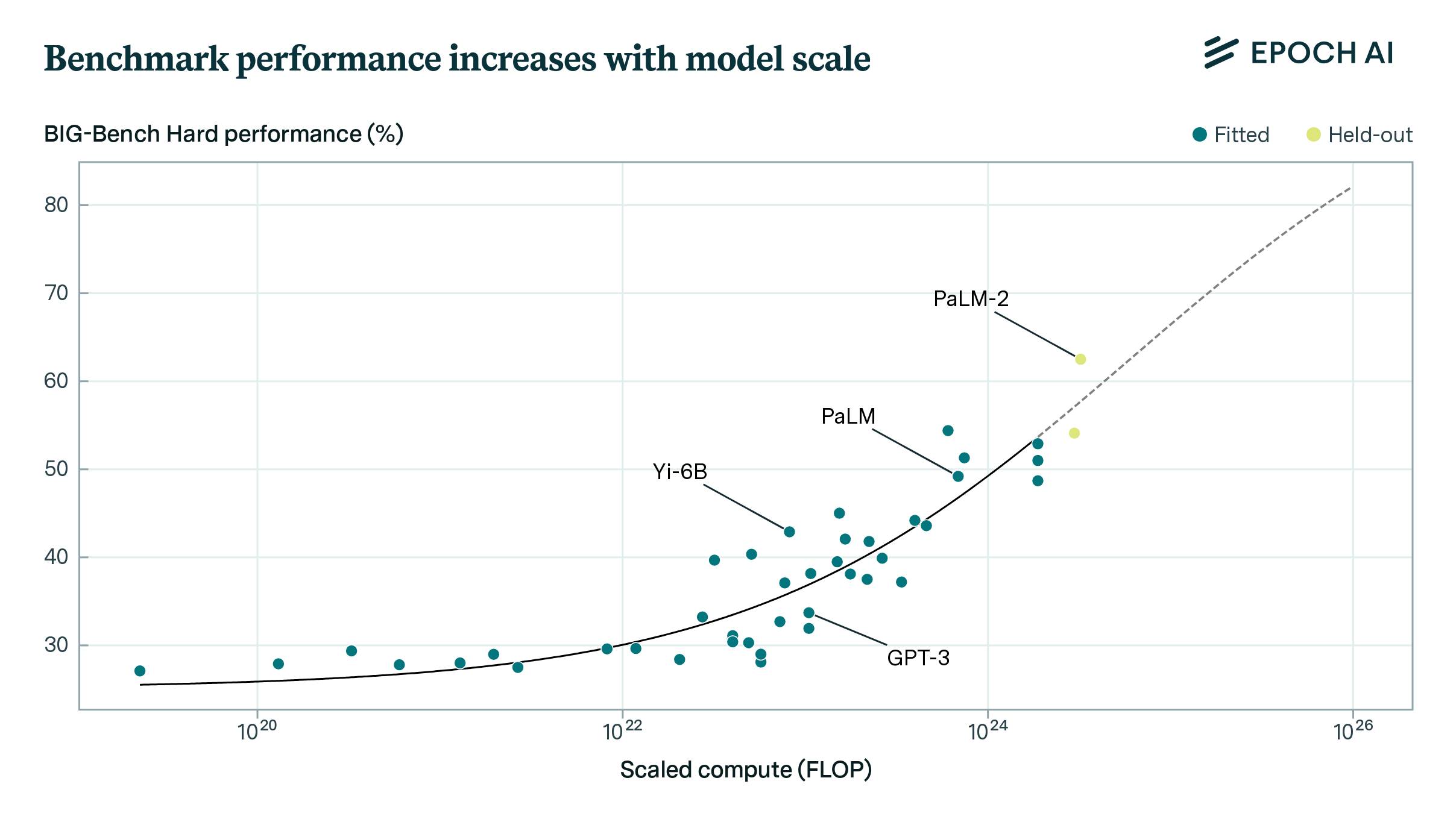
paper
· 3 min read
How Predictable Is Language Model Benchmark Performance?
We investigate large language model performance, finding that compute-focused extrapolations are a promising way to forecast AI capabilities.
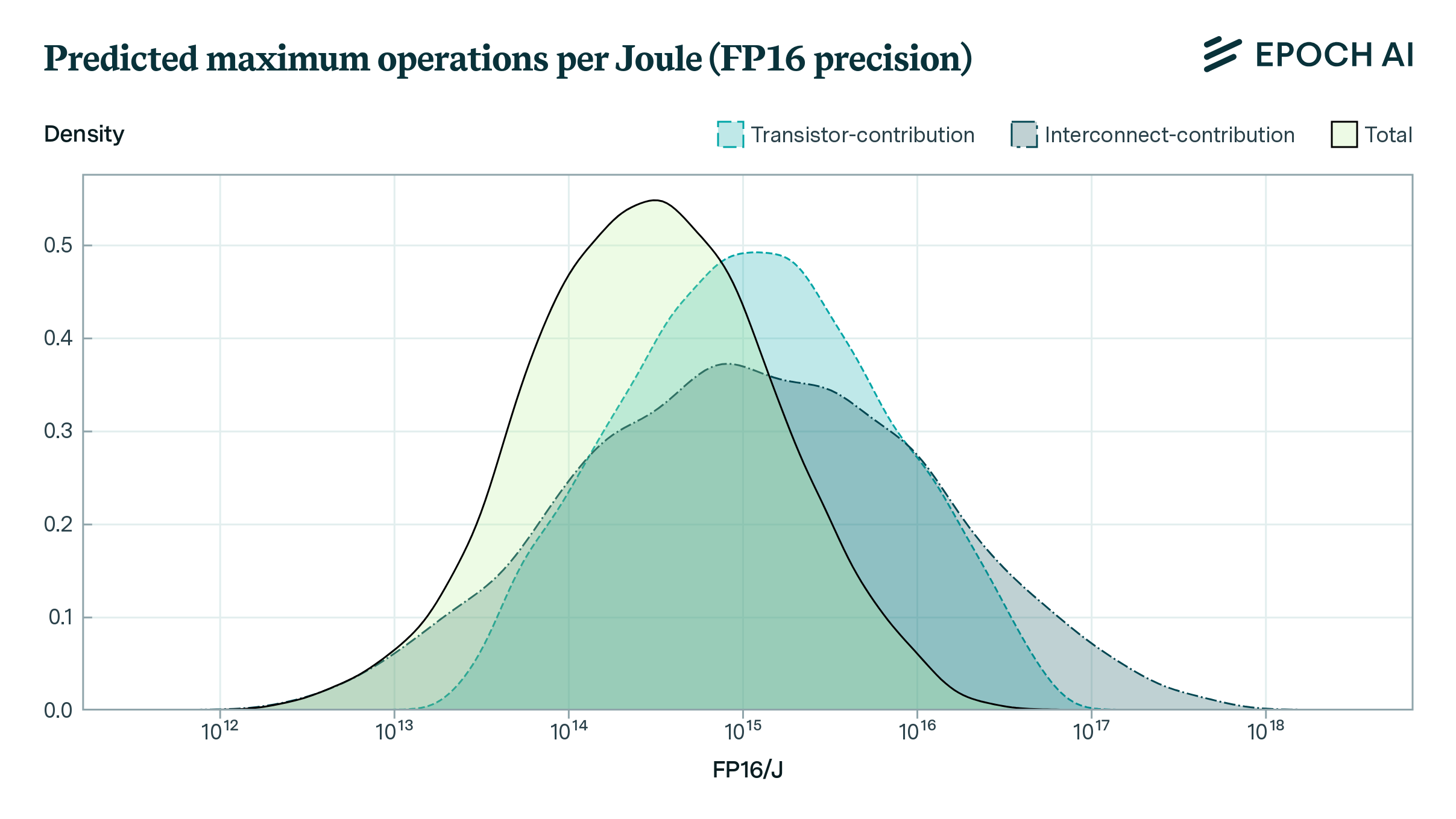
paper
· 4 min read
Limits to the Energy Efficiency of CMOS Microprocessors
How far can the energy efficiency of CMOS microprocessors be pushed before hitting physical limits? We find room for a further 50 to 1000x improvement.
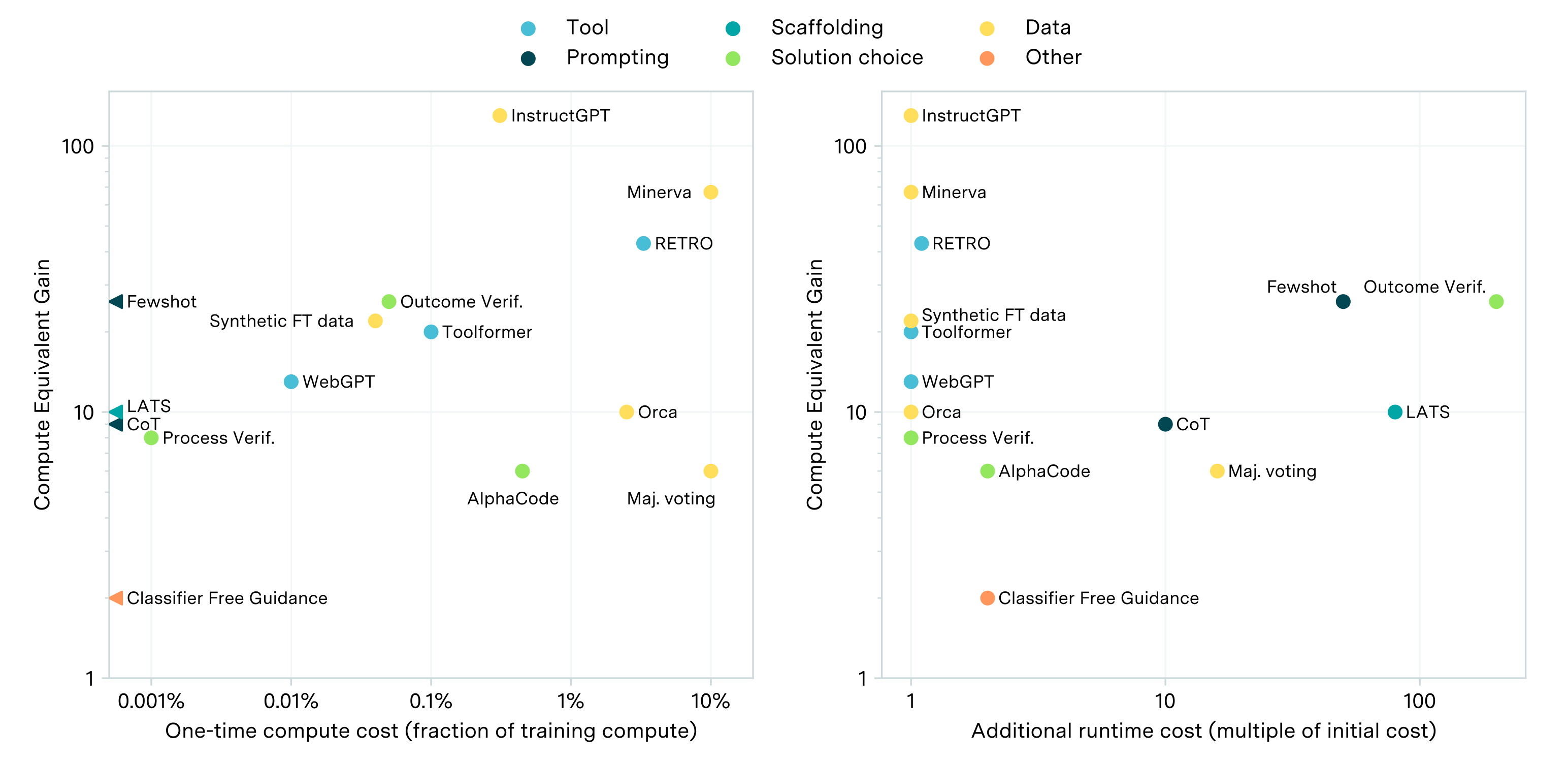
paper
· 2 min read
AI Capabilities Can Be Significantly Improved Without Expensive Retraining
While scaling compute is key to improving LLMs, post-training enhancements can offer gains equivalent to 5-20x more compute at less than 1% of the cost.
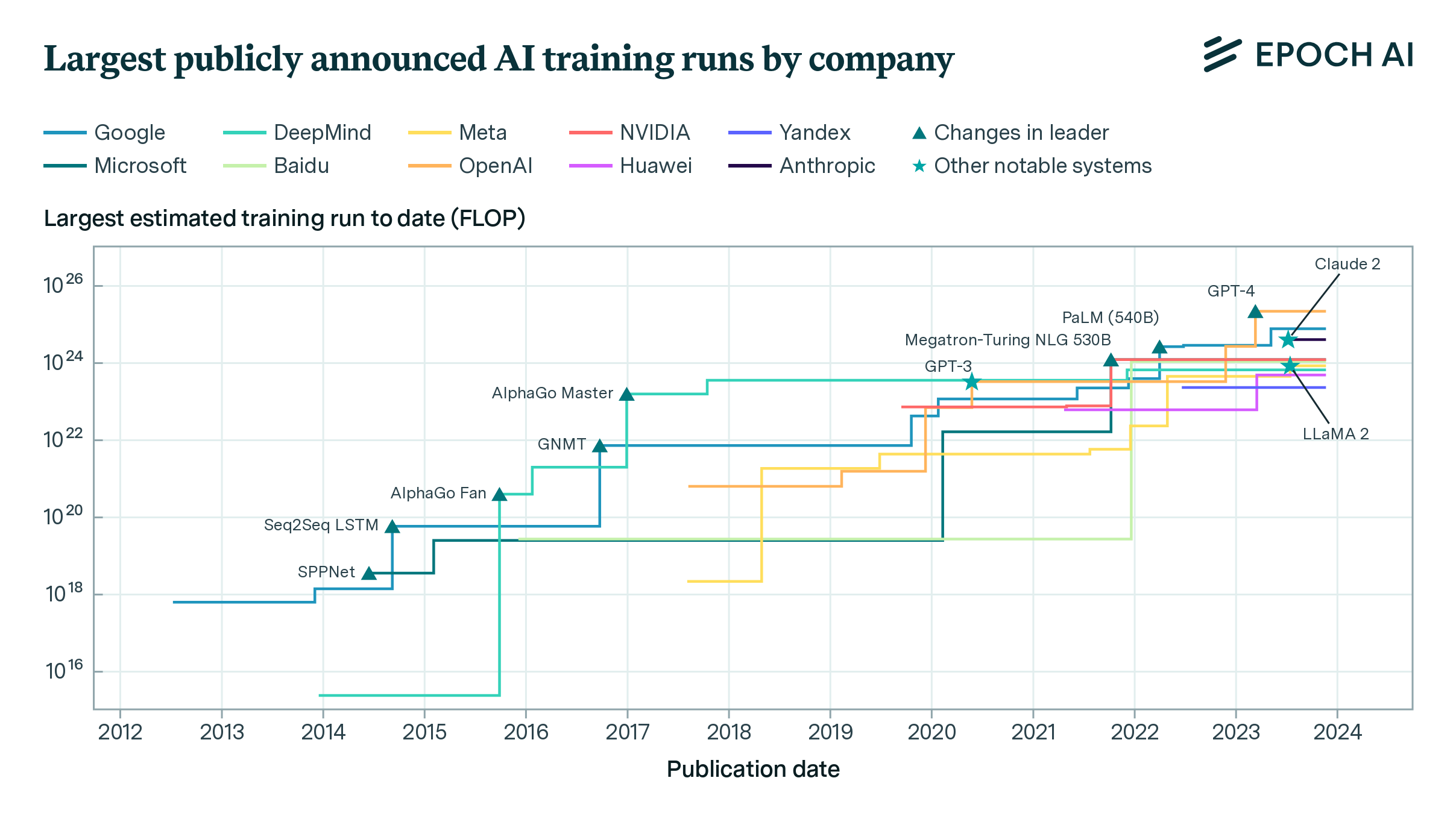
paper
· 3 min read
Who Is Leading in AI? An Analysis of Industry AI Research
Industry has emerged as a driving force in AI. We compare top companies on research impact, training runs, and contributions to algorithmic innovations.
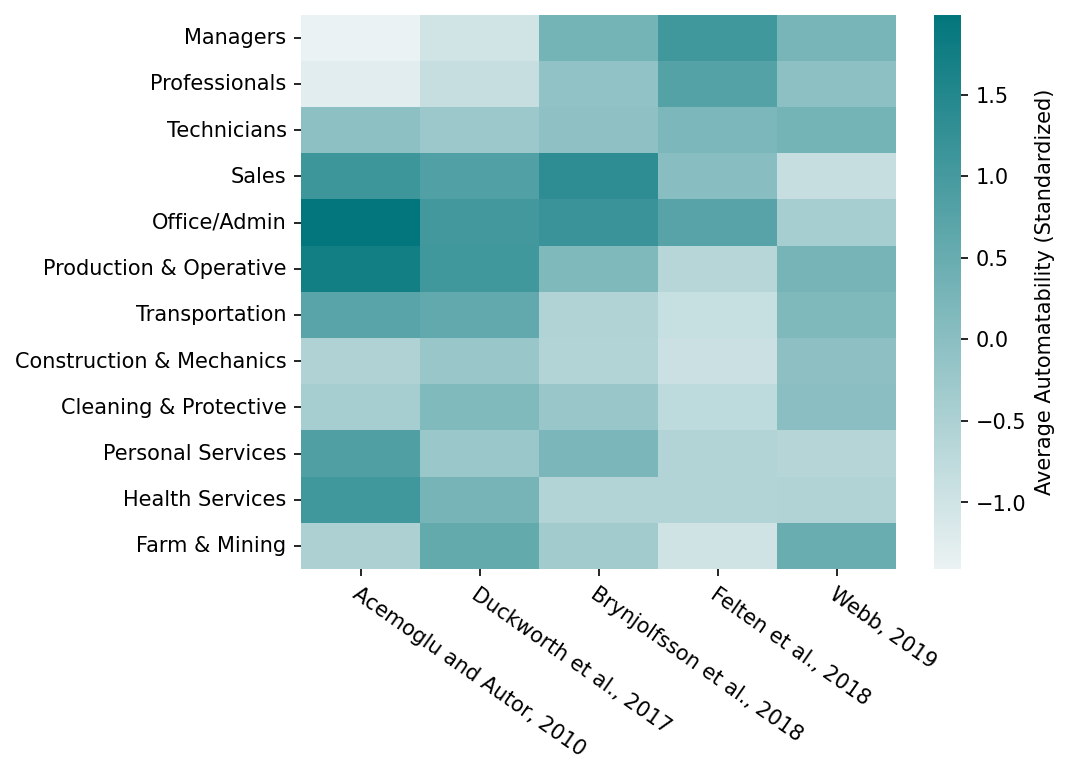
report
· 31 min read
Challenges in Predicting AI Automation
Economists propose various approaches to predicting AI's automation of valuable tasks, but disagreements persist, with no consensus on the best method.
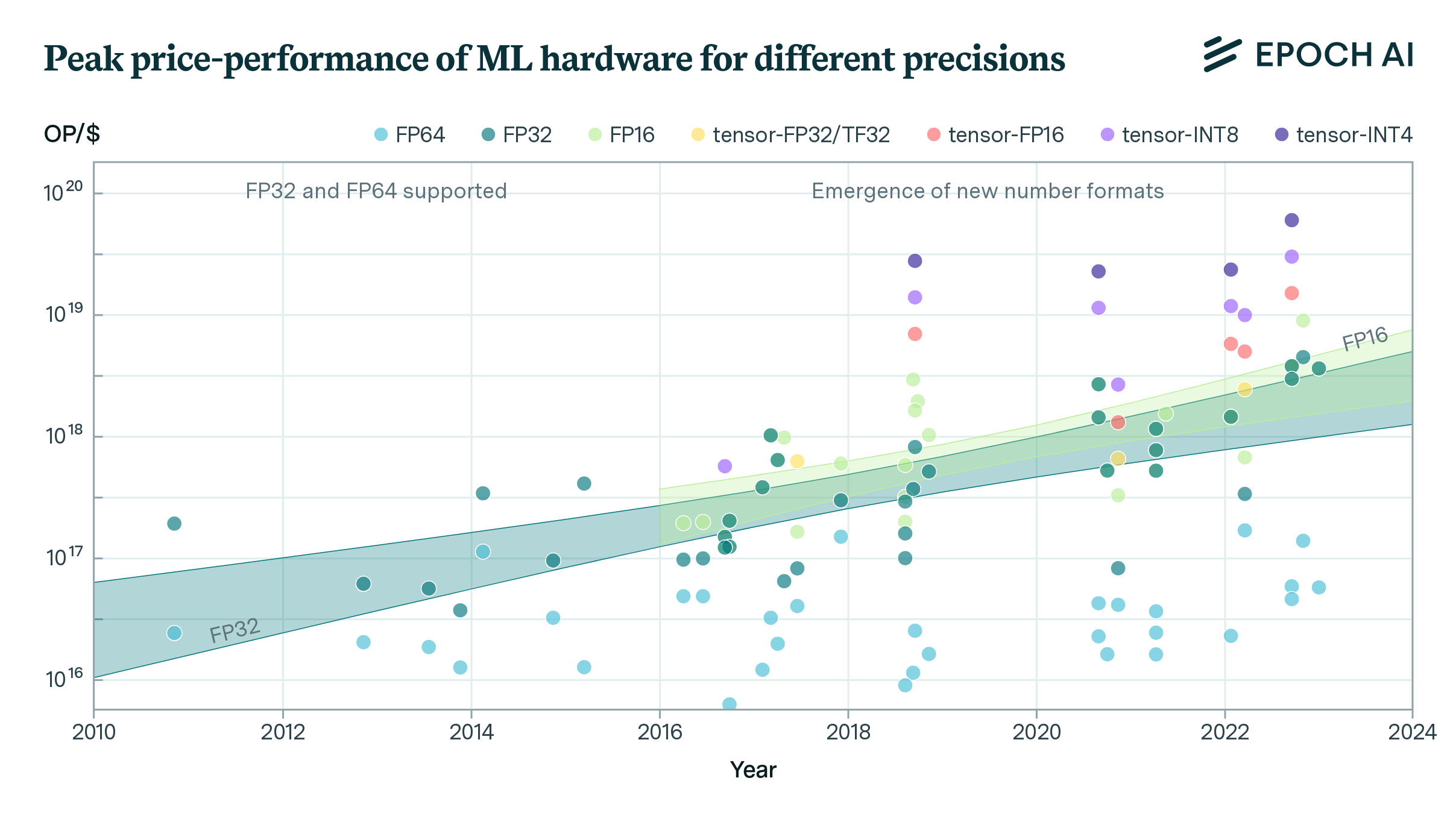
report
· 27 min read
Trends in Machine Learning Hardware
FLOP/s performance in 47 ML hardware accelerators doubled every 2.3 years. Switching from FP32 to tensor-FP16 led to a further 10x performance increase.
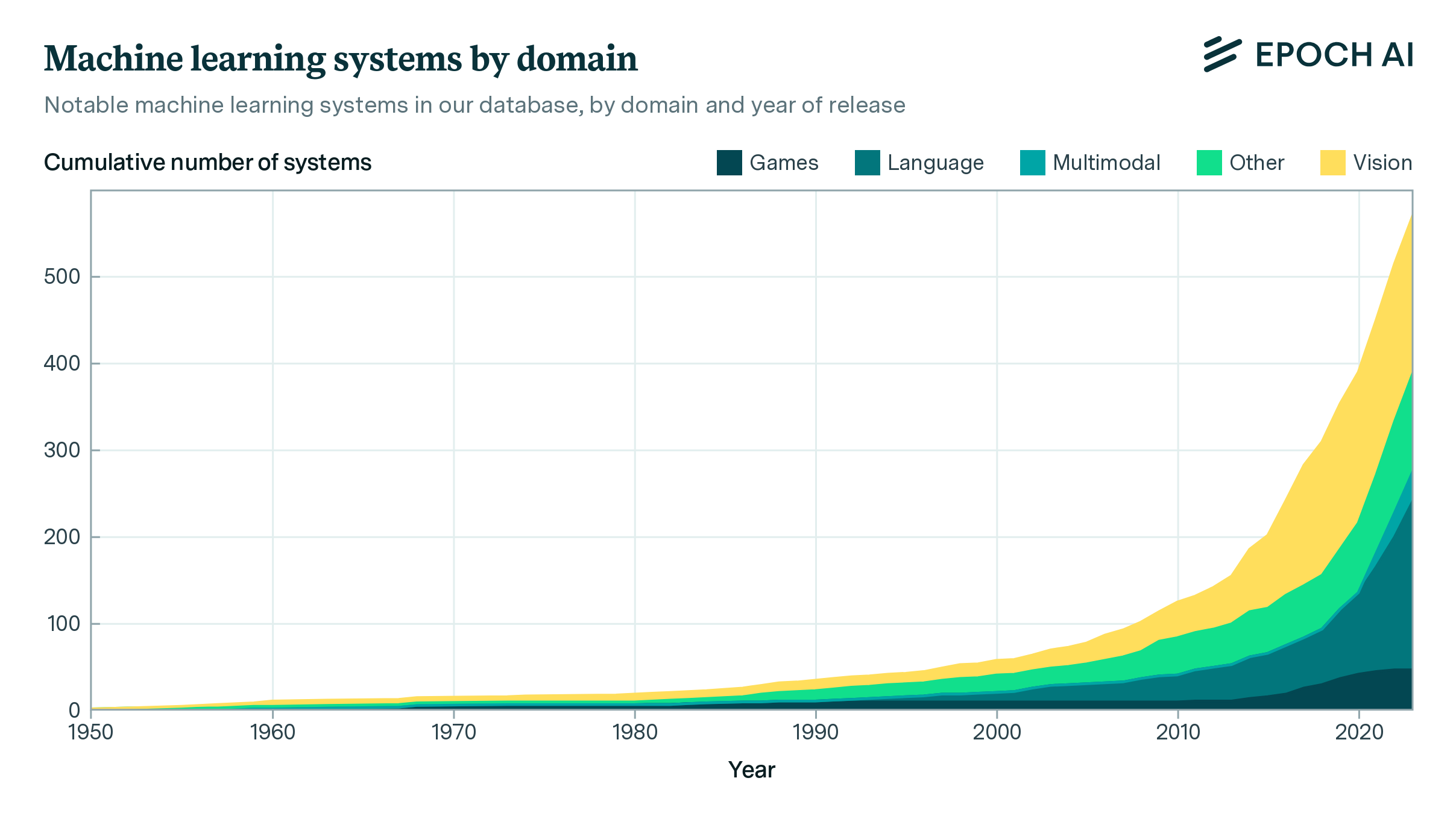
announcement
· 1 min read
Announcing Epoch AI’s Updated Parameter, Compute and Data Trends Database
Our database now spans over 700 ML systems, tracking parameters, datasets, and training compute details for notable machine learning models.
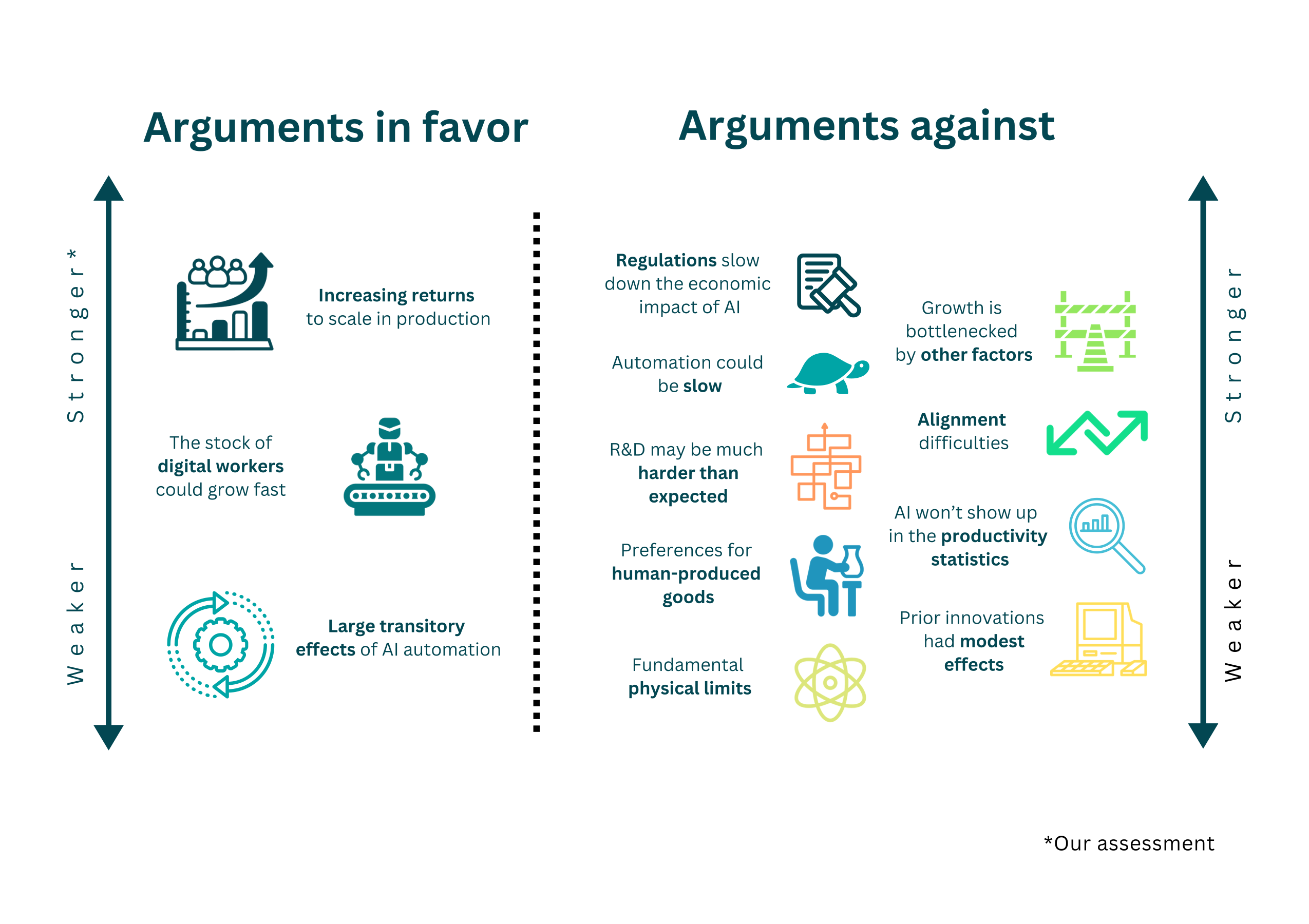
paper
· 11 min read
Explosive Growth from AI: A Review of the Arguments
Our new article explores whether deployment of advanced AI systems could lead to growth rates ten times higher than those of today’s frontier economies.
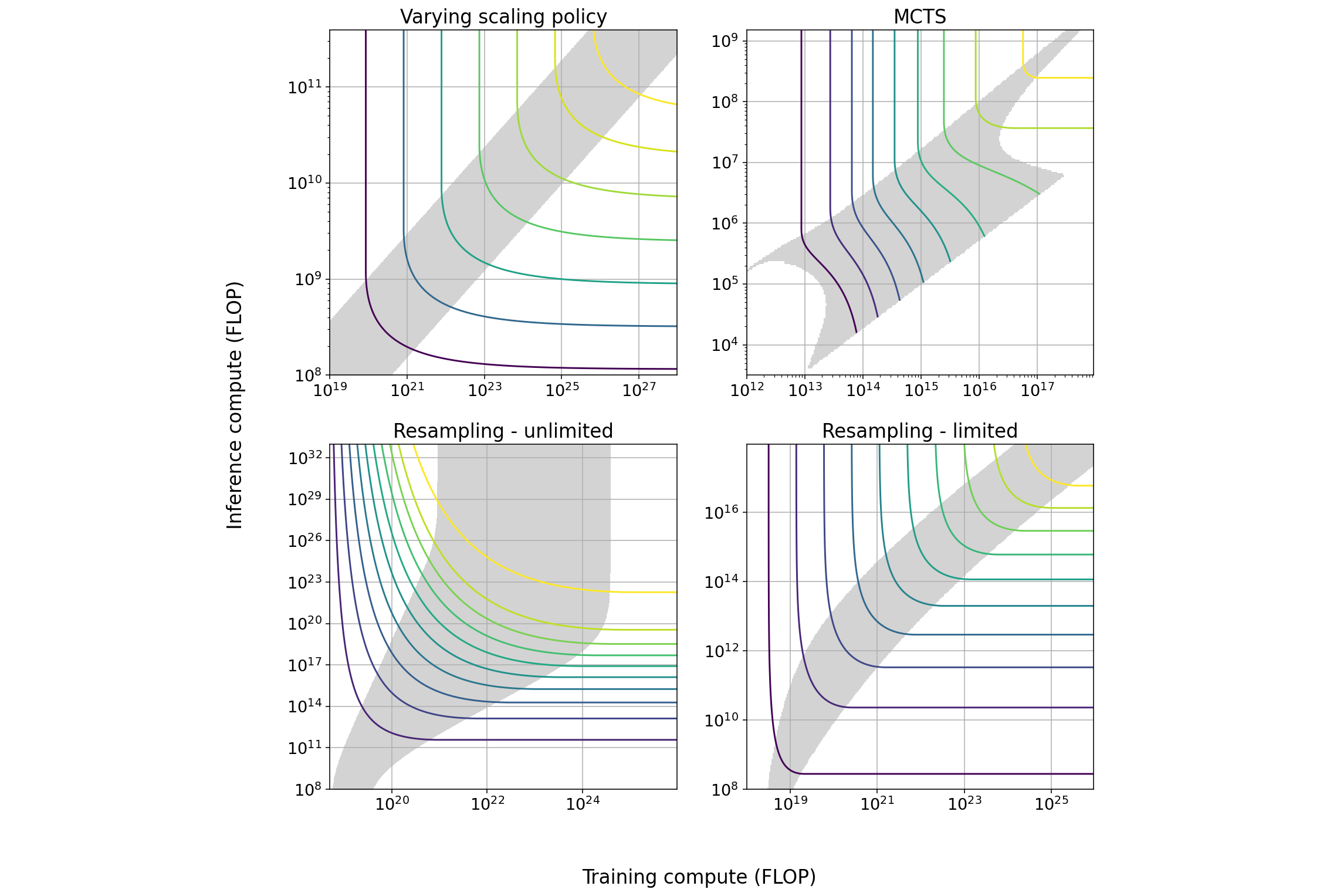
report
· 27 min read
Trading Off Compute in Training and Inference
We characterize techniques that induce a tradeoff between spending resources on training and inference, outlining their implications for AI governance.
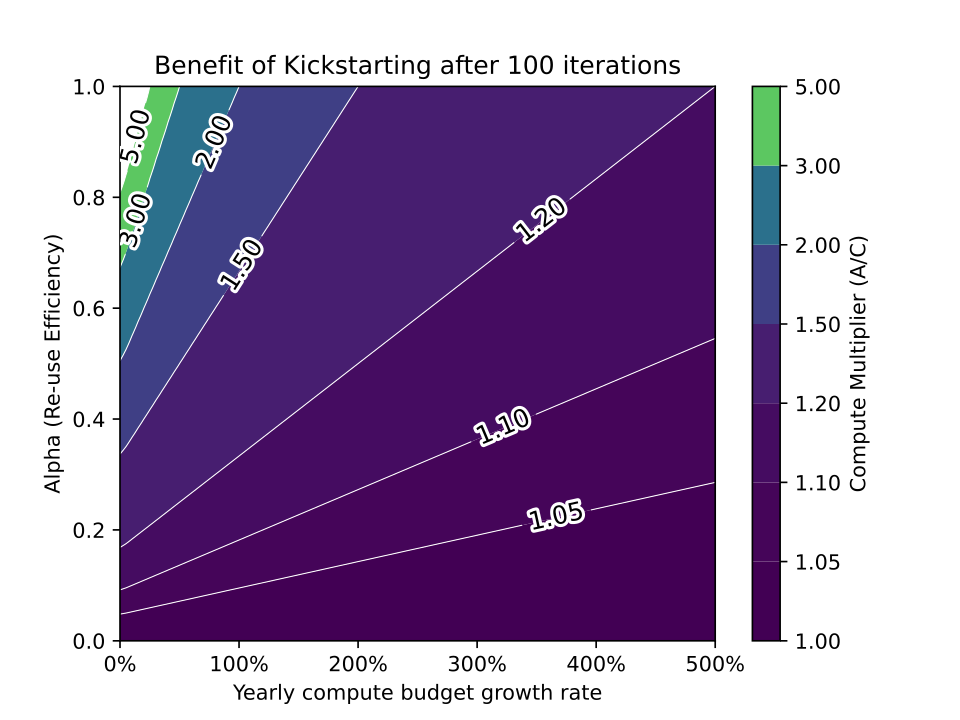
report
· 10 min read
The Limited Benefit of Recycling Foundation Models
Reusing pretrained models can save on training costs, but it's unlikely to significantly boost AI capabilities beyond modest improvements.
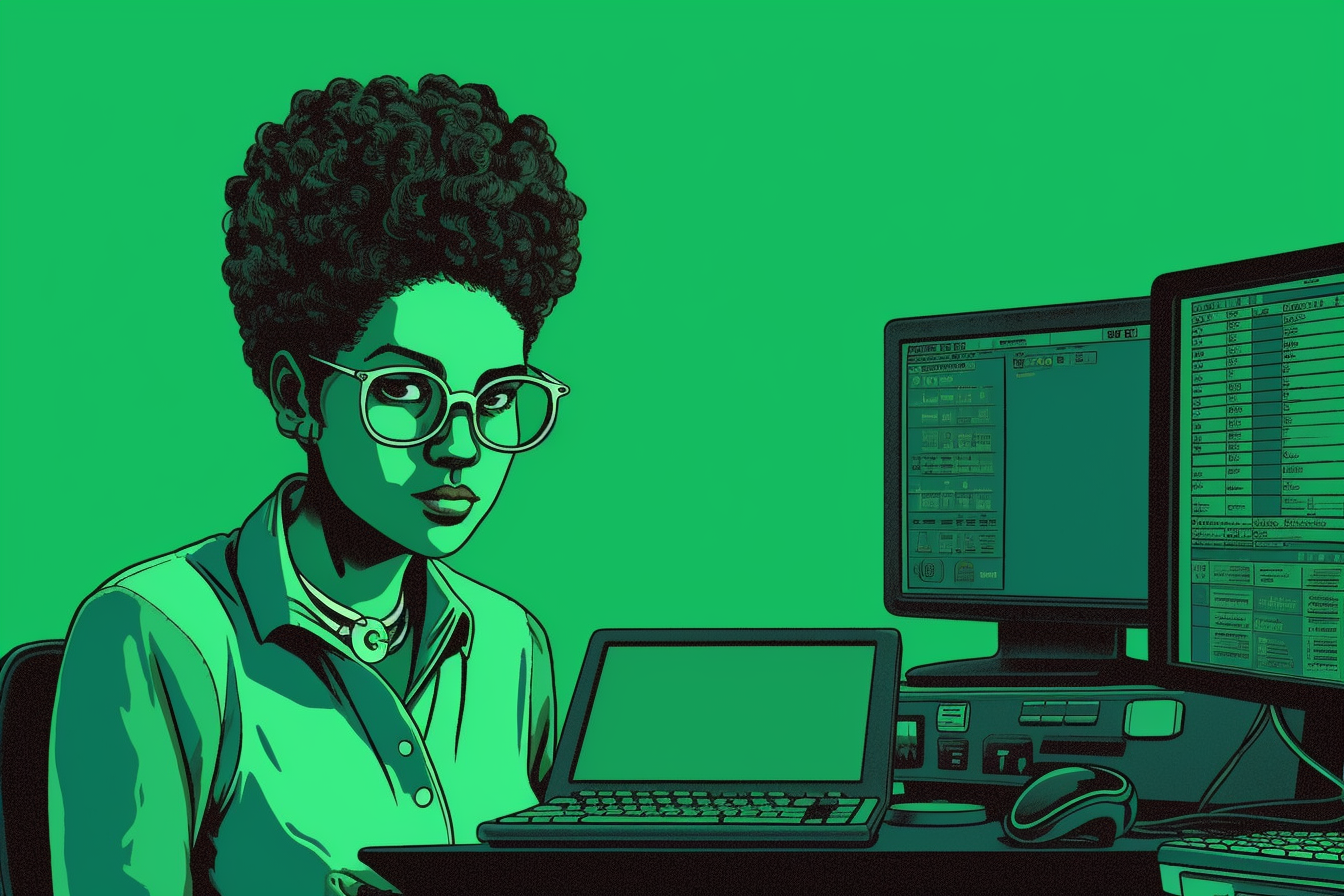
announcement
· 3 min read
Epoch AI and FRI Mentorship Program Summer 2023
We’re launching the Epoch and FRI mentorship program for women, non-binary, and transgender people interested in AI forecasting.
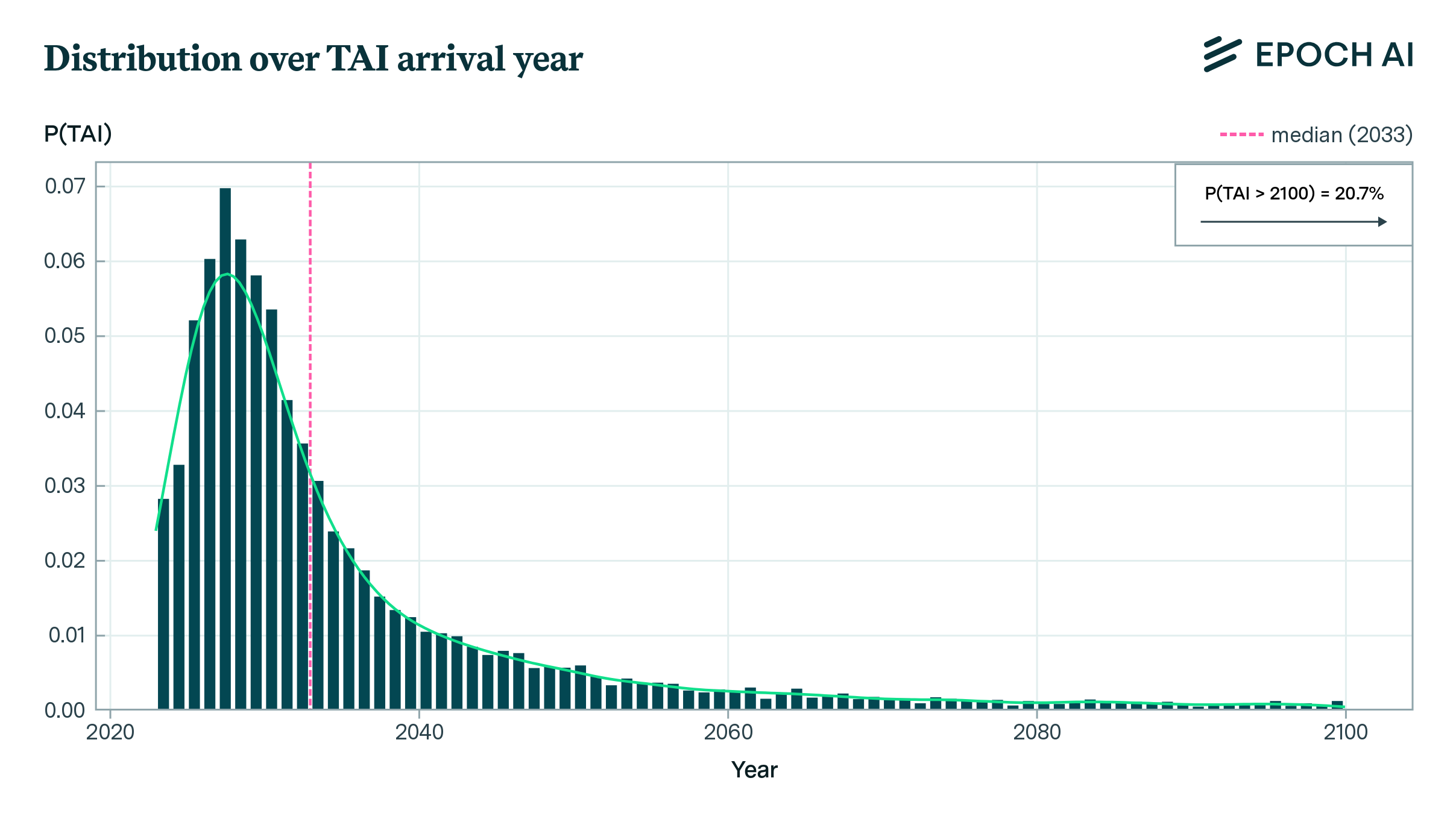
report
· 14 min read
Direct Approach Interactive Model
When could transformative AI be achieved? We present a simple, user-adjustable model of key inputs that forecasts the date TAI could be deployed.
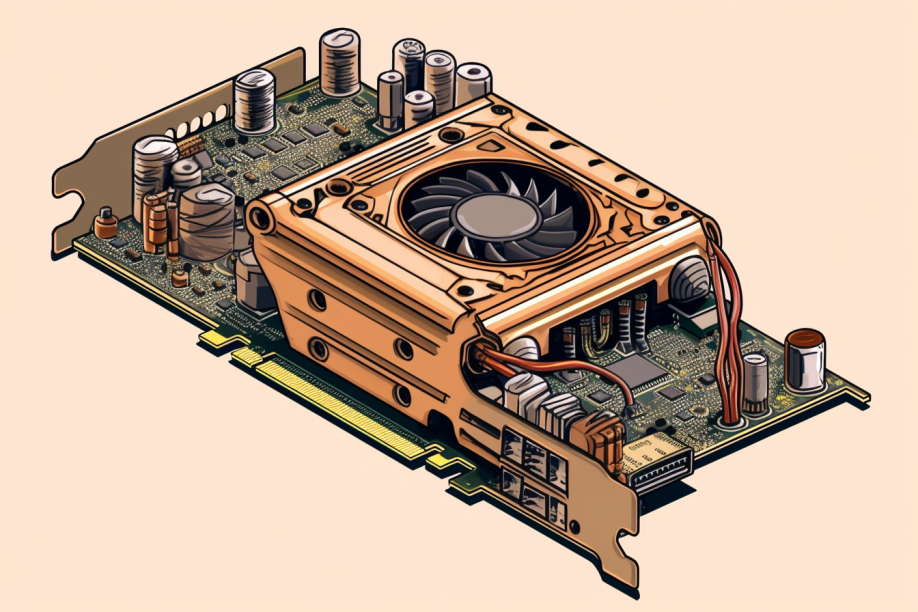
viewpoint
· 26 min read
A Compute-Based Framework for Thinking About the Future of AI
AI’s potential to automate labor could alter the course of human history. The availability of compute is the most important factor driving progress in AI.
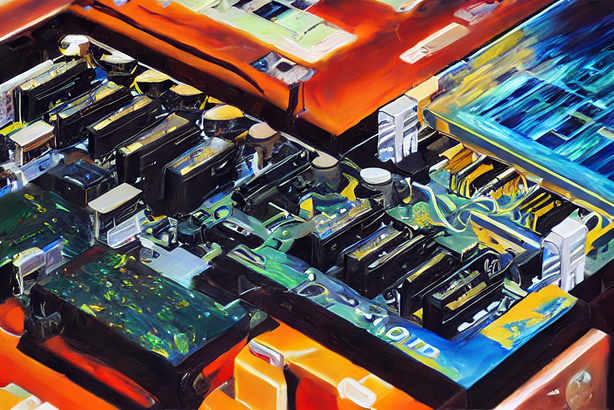
viewpoint
· 1 min read
Please Report Your Compute
Compute is essential for AI performance, yet often underreported. Adopting reporting norms would improve research, forecasts, and policy decisions.
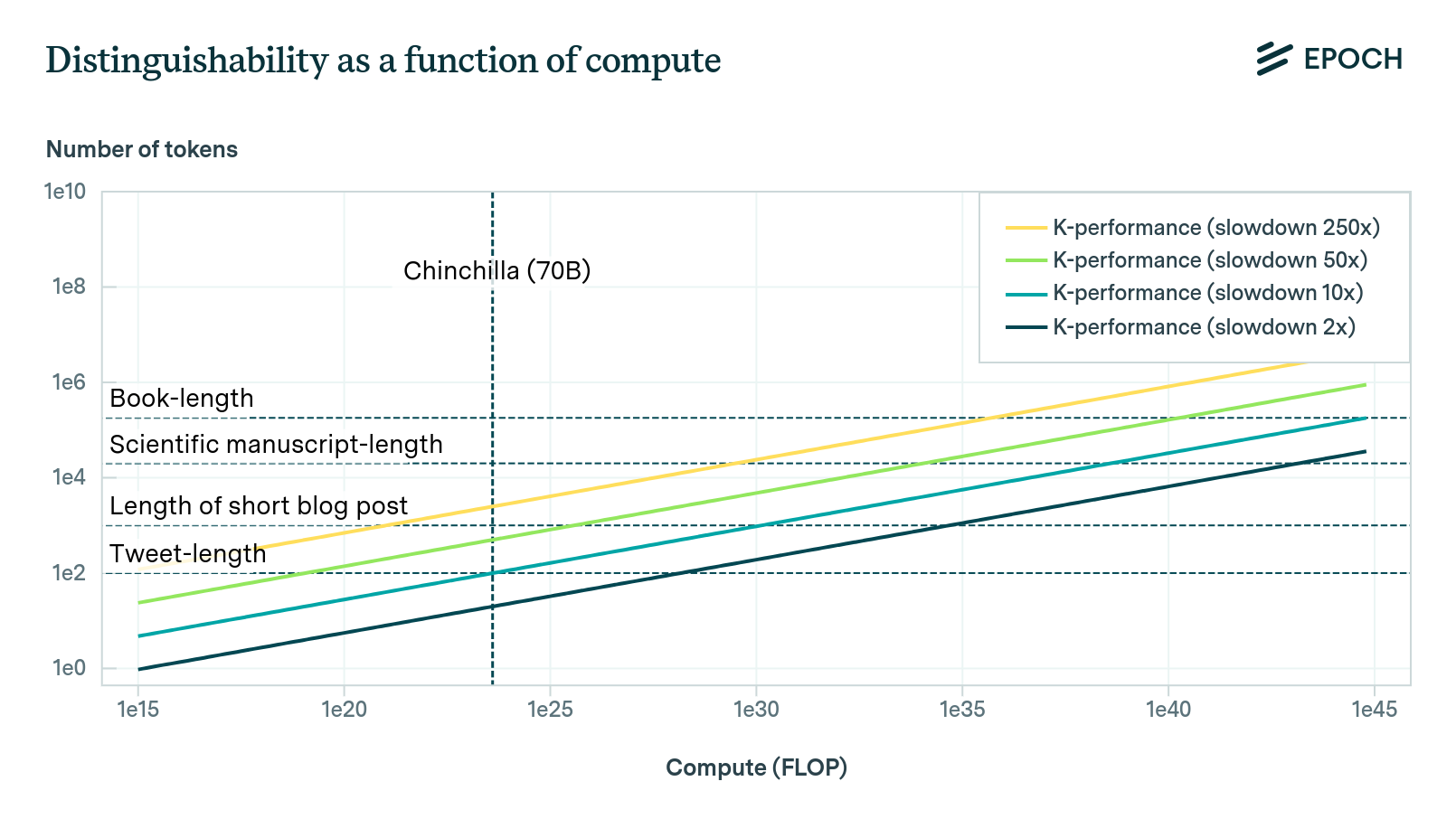
report
· 10 min read
The Direct Approach
We propose a method using neural scaling laws to estimate the compute needed to train AI models to reach human-level performance on various tasks.
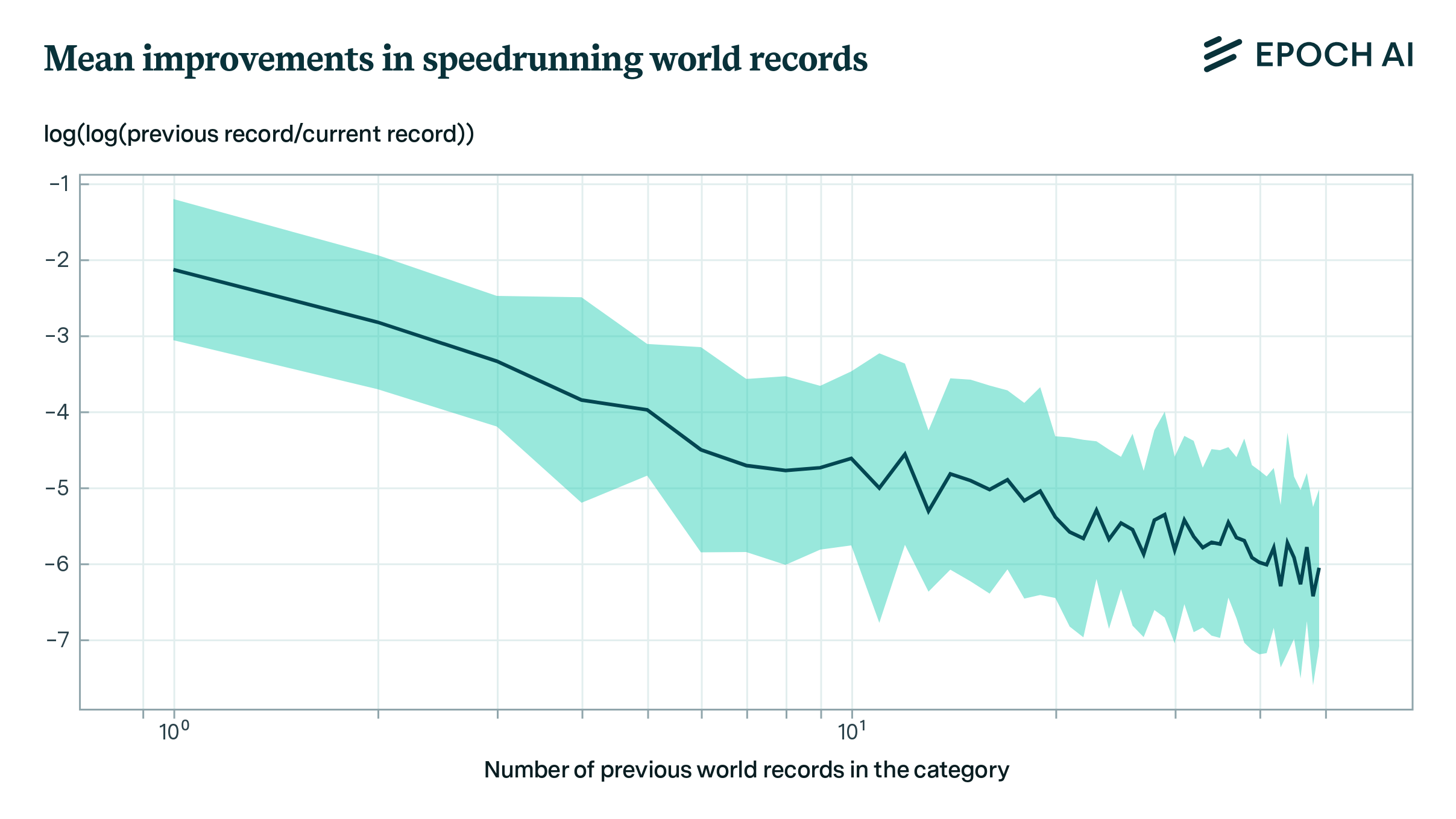
paper
· 2 min read
Power Laws in Speedrunning and Machine Learning
Our model suggests ML benchmarks aren’t near saturation. While large improvements are rare, we find 1OOM gains happen roughly once in every 50 instances.
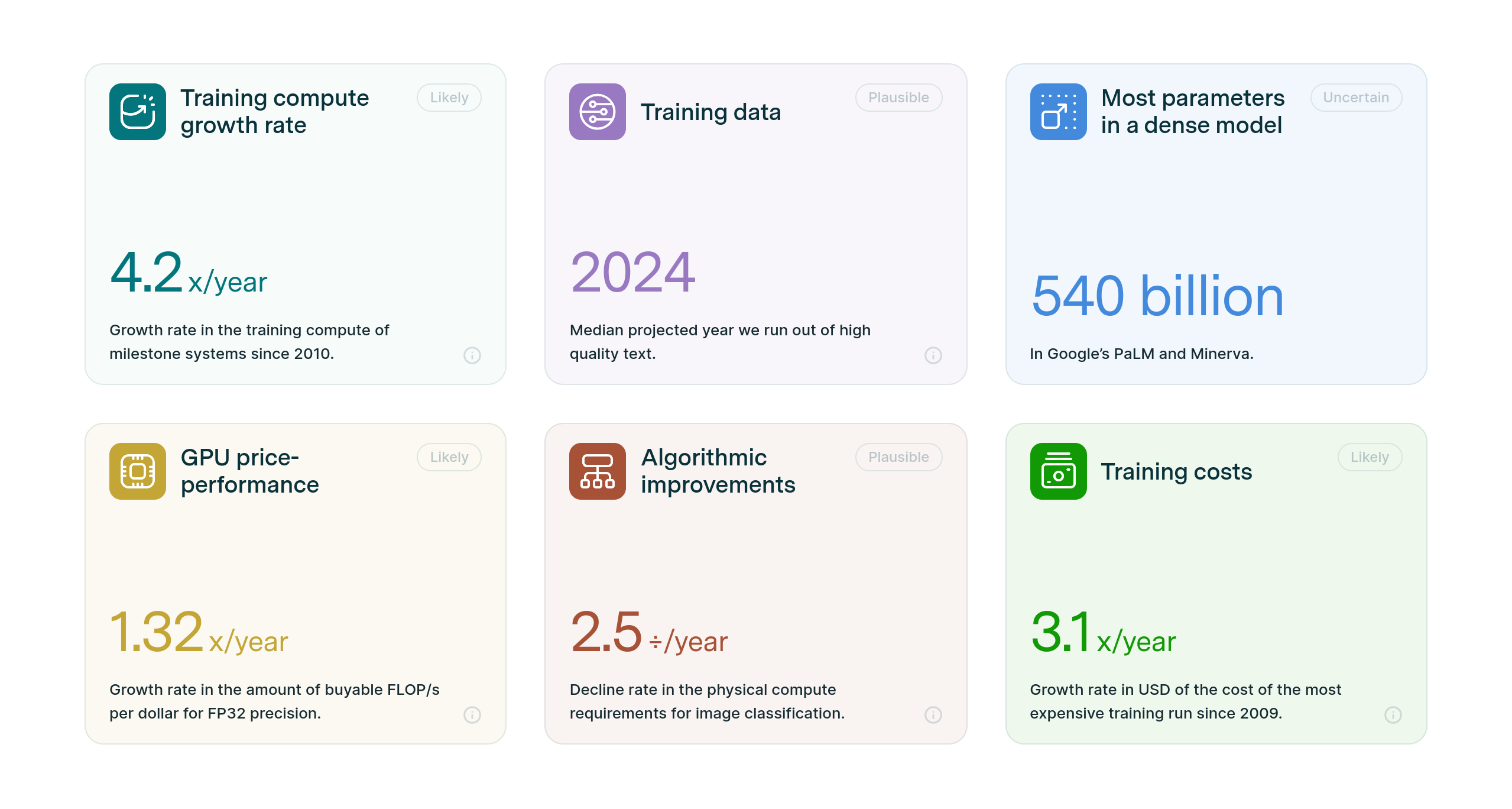
announcement
· 1 min read
Announcing Epoch AI’s Dashboard of Key Trends and Figures in Machine Learning
Our dashboard provides key data from our research on machine learning and is a valuable resource for understanding the present and future of the field.
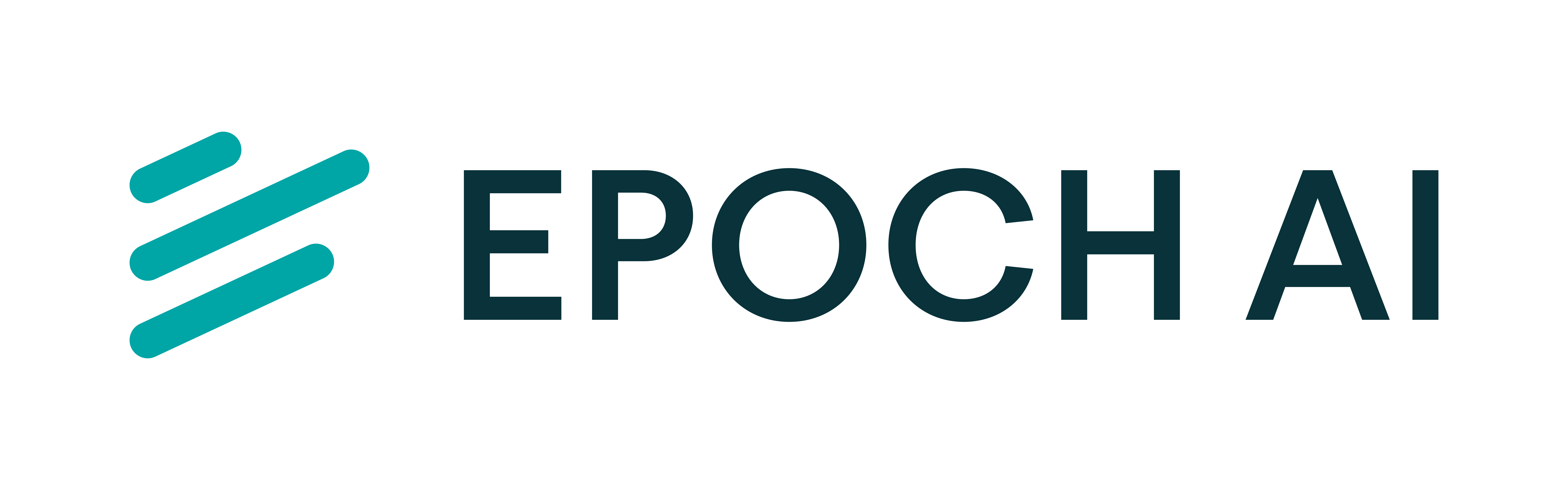
announcement
· 1 min read
Epoch AI Impact Assessment 2022
Our impact report for 2022.
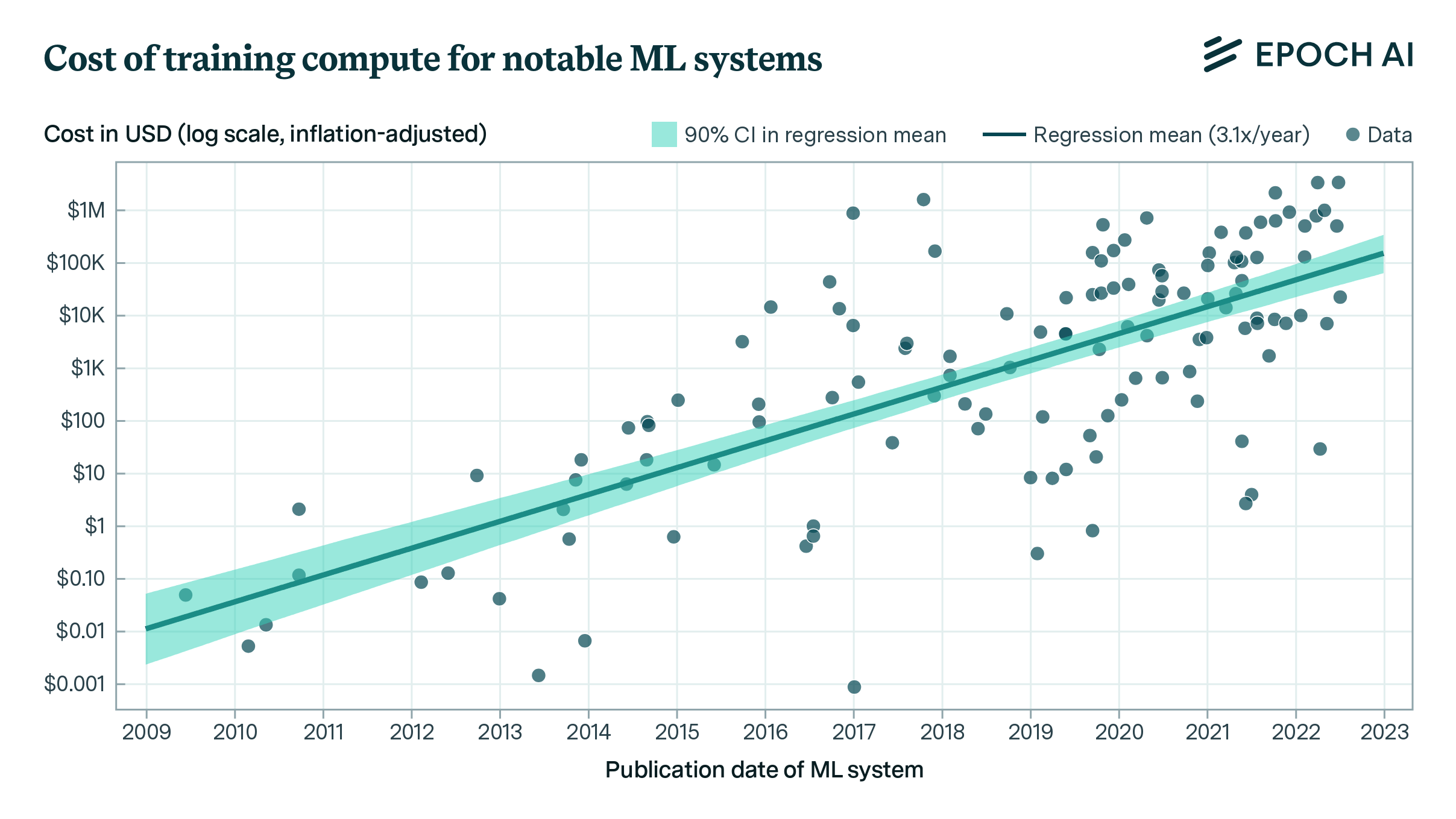
report
· 66 min read
Trends in the Dollar Training Cost of Machine Learning Systems
How much does it cost to train AI models? Looking at 124 ML systems from between 2009 and 2022, we find the cost has grown by approximately 0.5OOM/year.
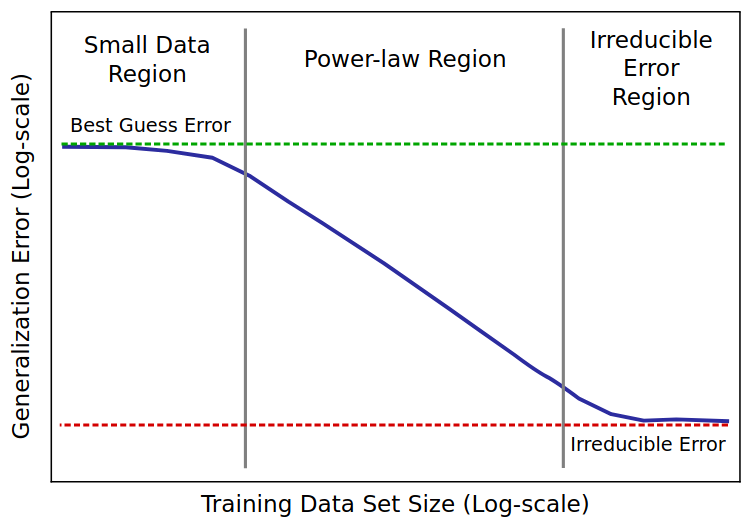
report
· 6 min read
Scaling Laws Literature Review
I have collected a database of scaling laws for different tasks and architectures, and reviewed dozens of papers in the scaling law literature.
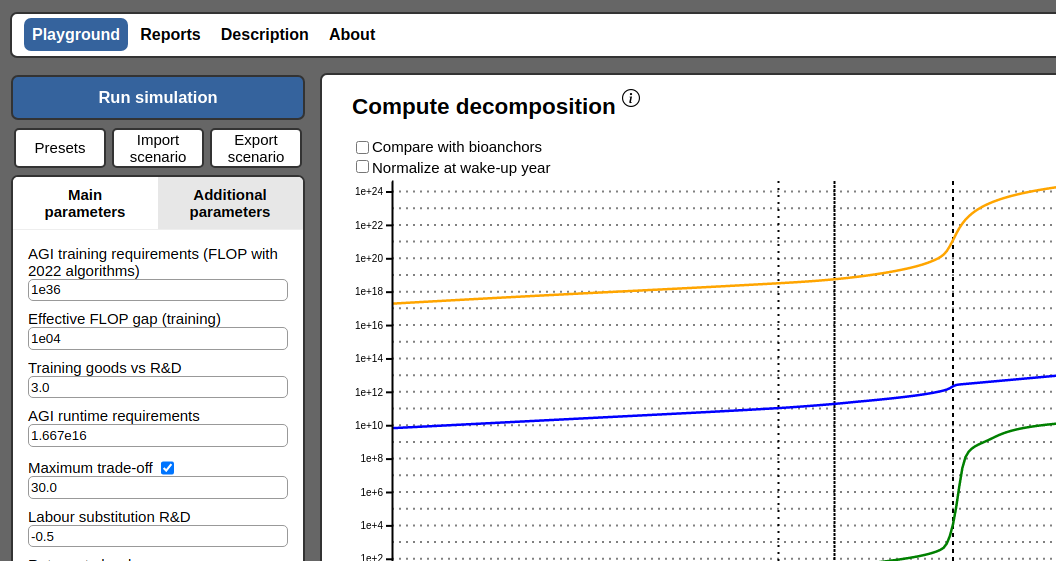
announcement
· 1 min read
An Interactive Model of AI Takeoff Speeds
We have developed an interactive website showcasing a new model of AI takeoff speeds.
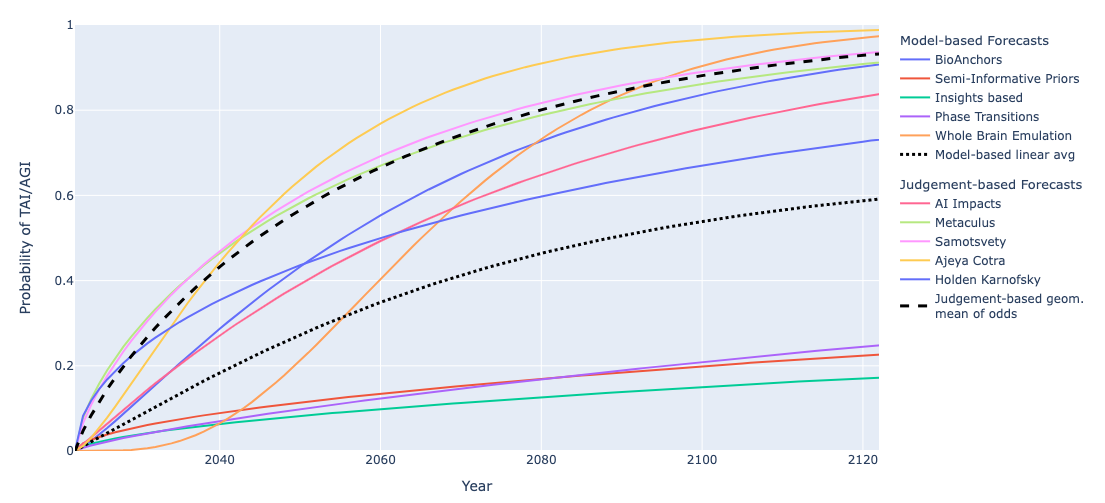
report
· 16 min read
Literature Review of Transformative Artificial Intelligence Timelines
We summarize and compare several models and forecasts predicting when transformative AI will be developed.
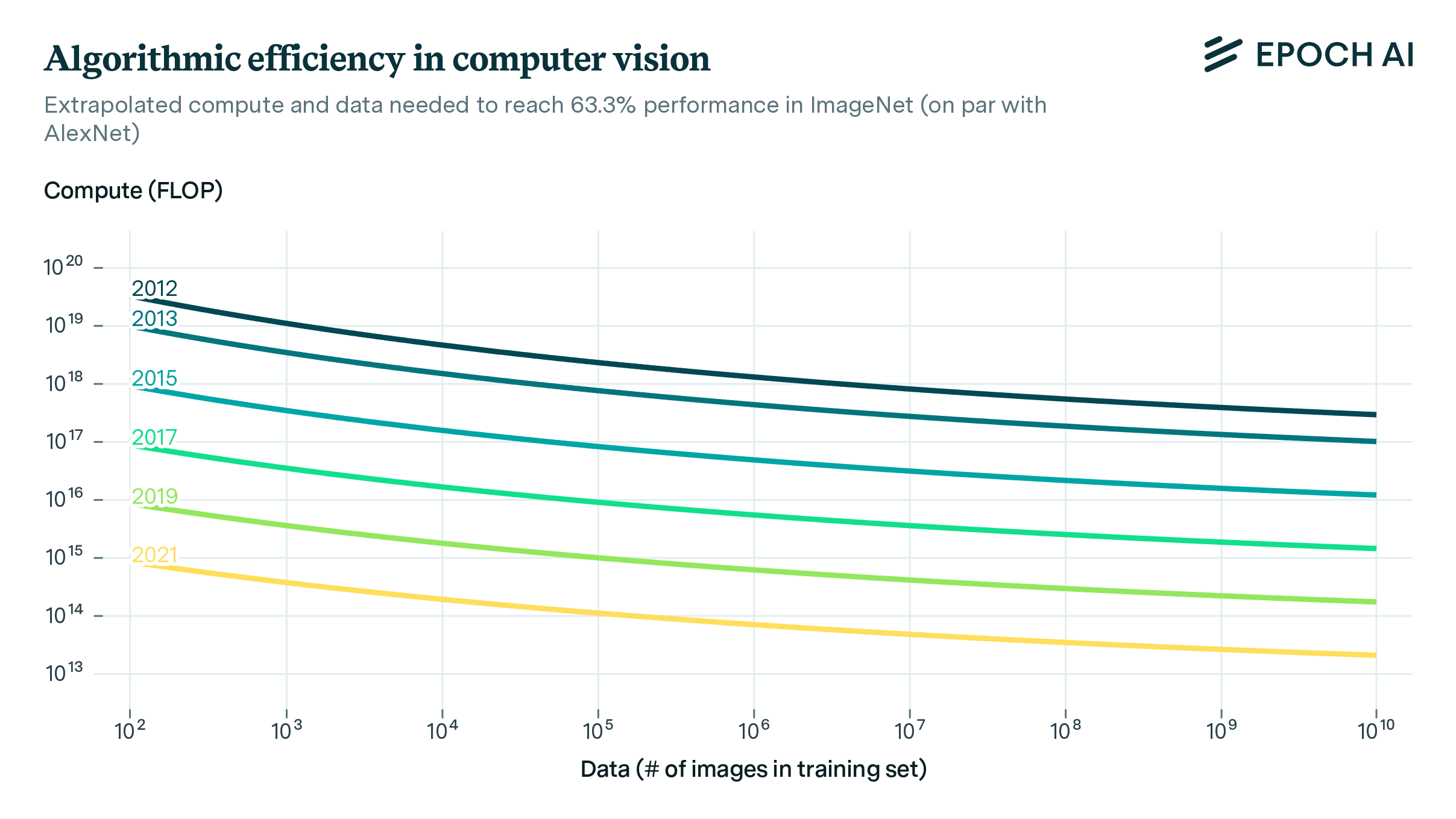
paper
· 2 min read
Revisiting Algorithmic Progress
Examining over 100 computer vision models, we find that every 9 months, better algorithms contribute the equivalent of a doubling of compute budgets.
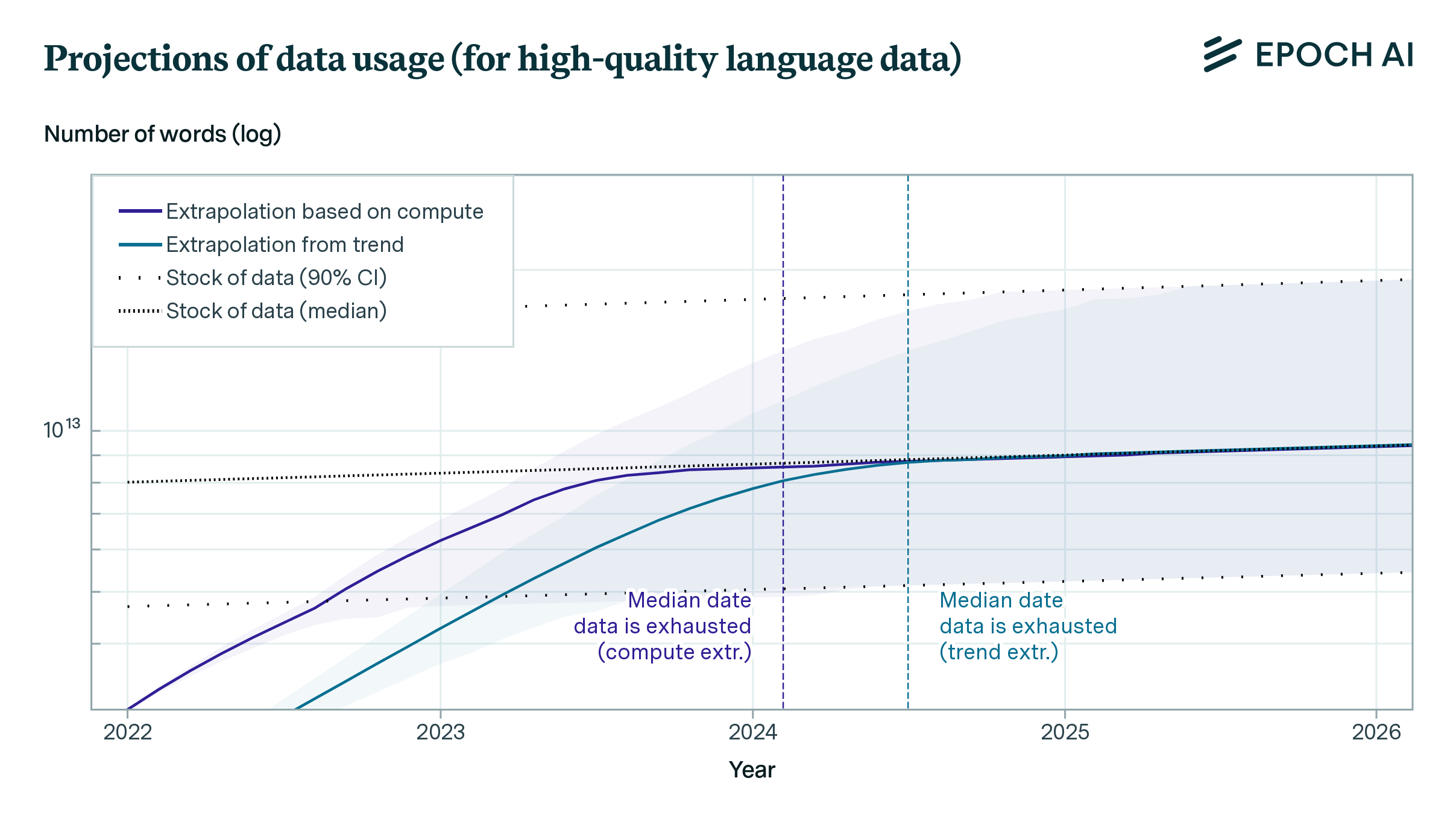
paper
· 3 min read
Will We Run Out of ML Data? Evidence From Projecting Dataset Size Trends
We project dataset growth in language and vision domains, estimating future limits to training by evaluating the availability of unlabeled data over time.
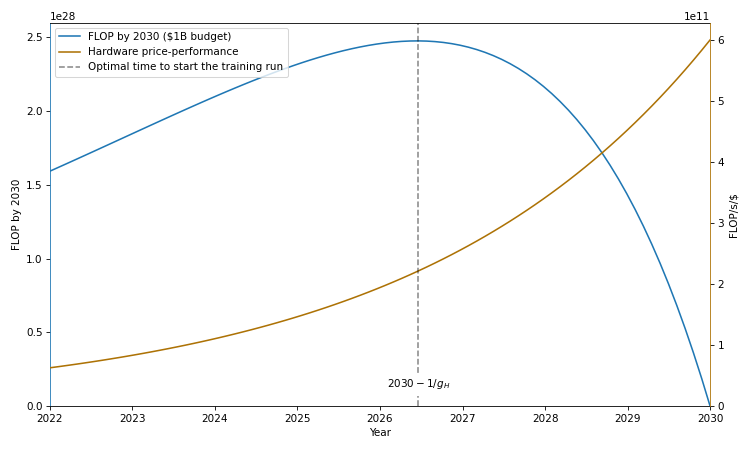
report
· 12 min read
The Longest Training Run
Training runs of large ML systems will likely last less than 14-15 months, as shorter runs starting later use better hardware and algorithms.
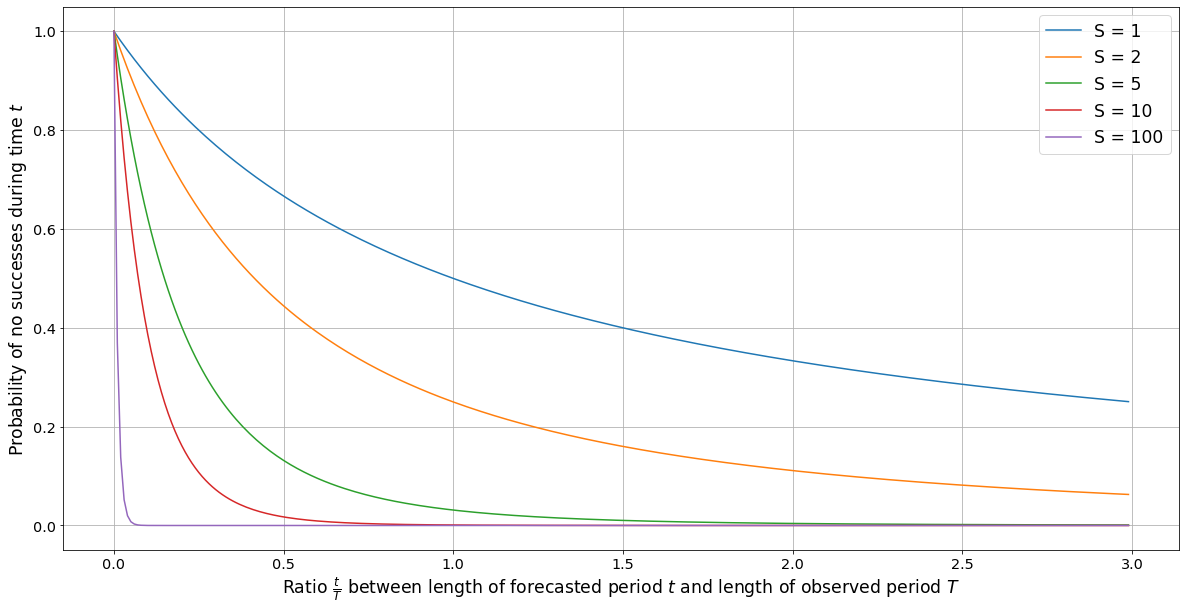
report
· 22 min read
A Time-Invariant Version of Laplace’s Rule
We discuss estimating event probabilities with past data, addressing issues with Laplace’s rule and proposing a modification to improve accuracy.
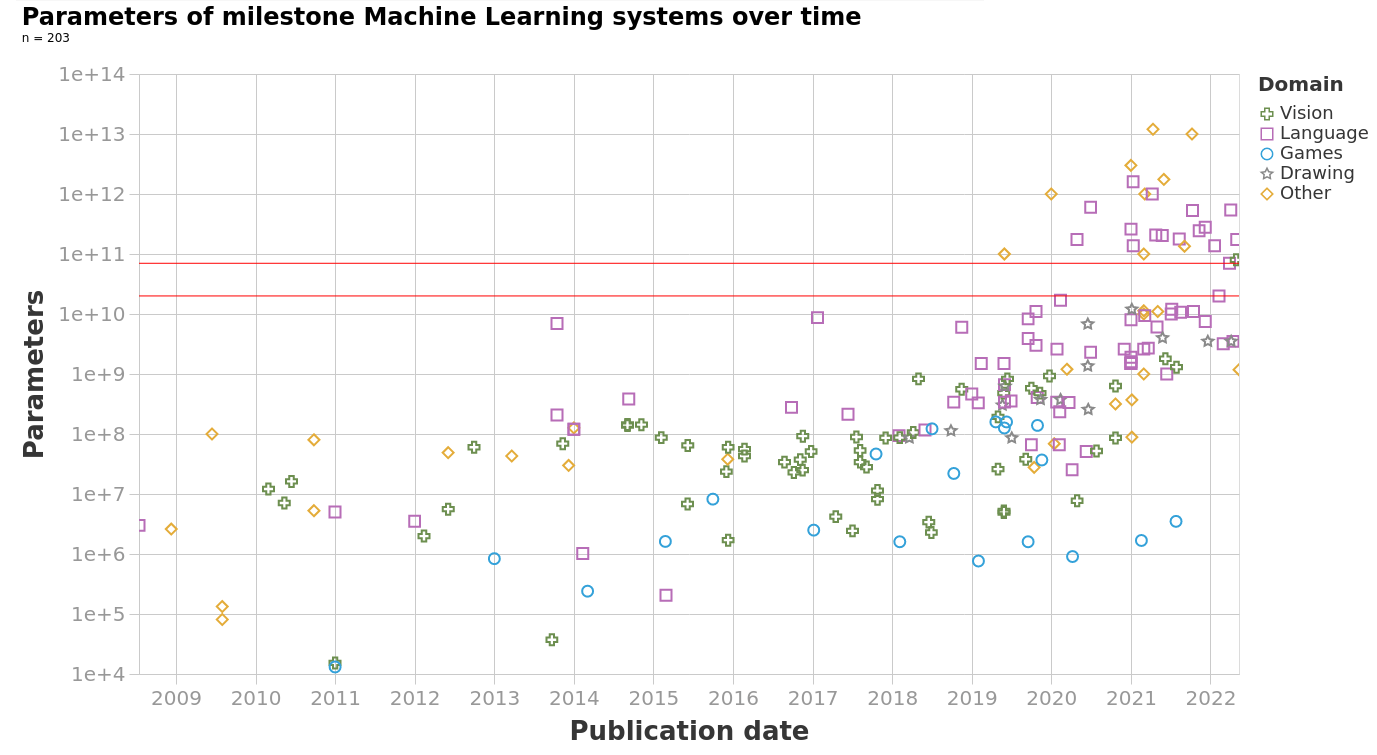
paper
· 2 min read
Machine Learning Model Sizes and the Parameter Gap
Since 2018, the size of ML models has been growing 10 times faster than before. Around 2020, model sizes saw a significant jump, increasing by 1OOM.
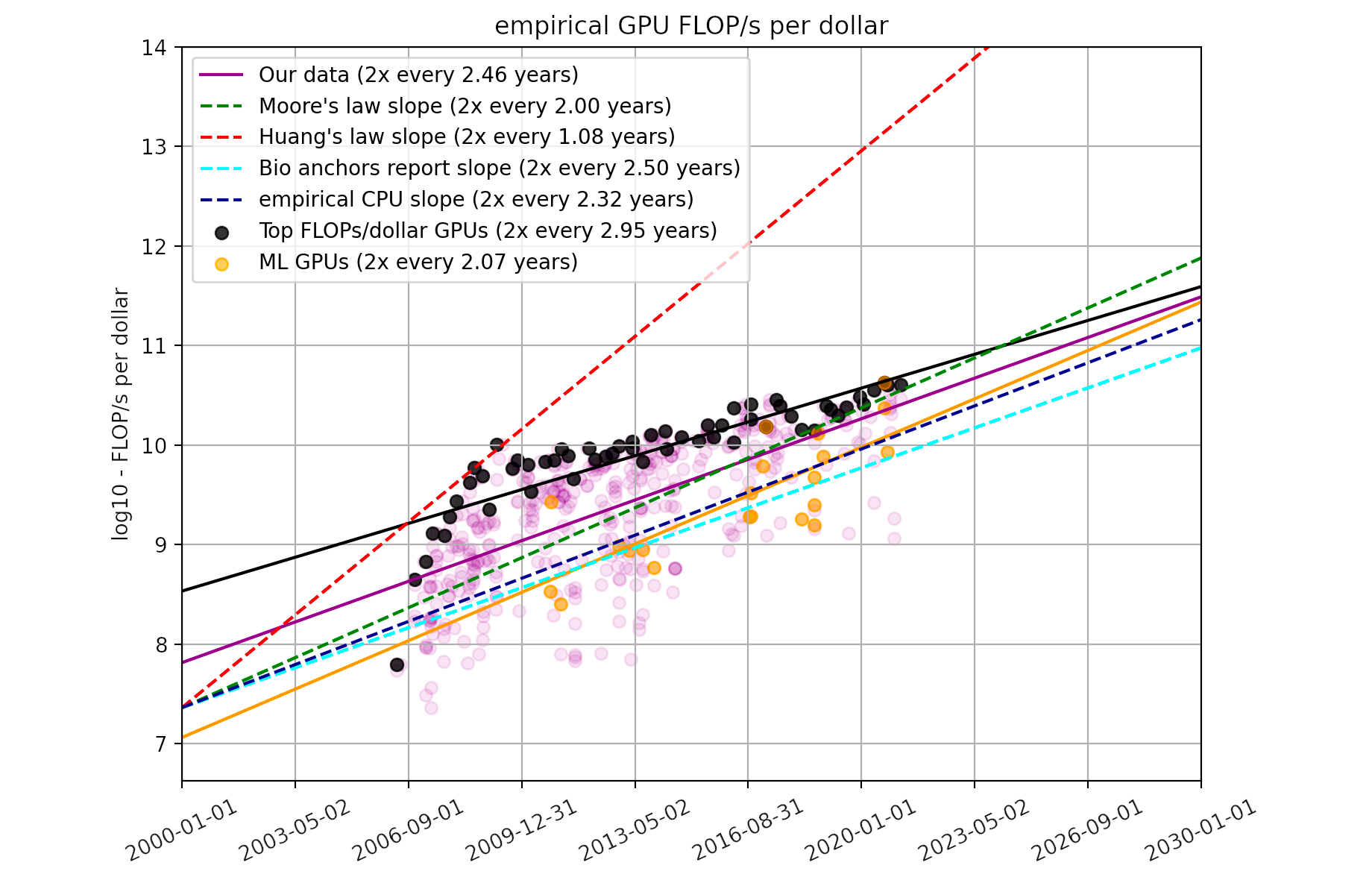
report
· 14 min read
Trends in GPU Price-Performance
Improvements in hardware are central to AI progress. Using data on 470 GPUs from 2006 to 2021, we find that FLOP/s per dollar doubles every ~2.5 years.
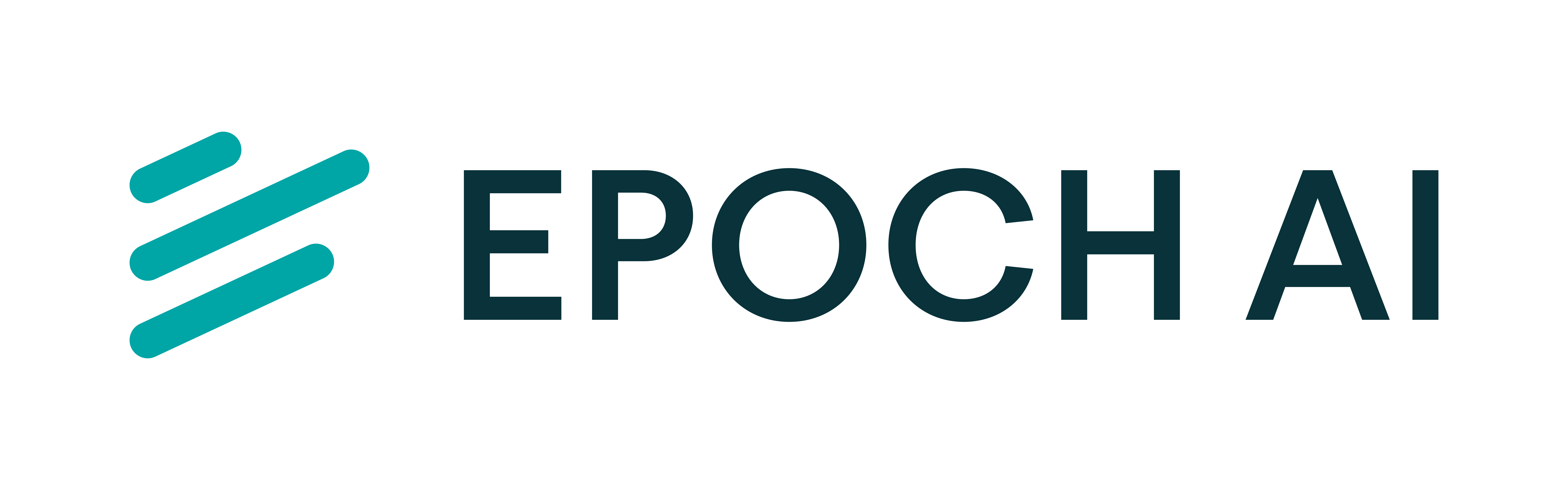
announcement
· 4 min read
Announcing Epoch AI: A Research Initiative Investigating the Road to Transformative AI
We are a new research initiative forecasting developments in AI. Come join us!
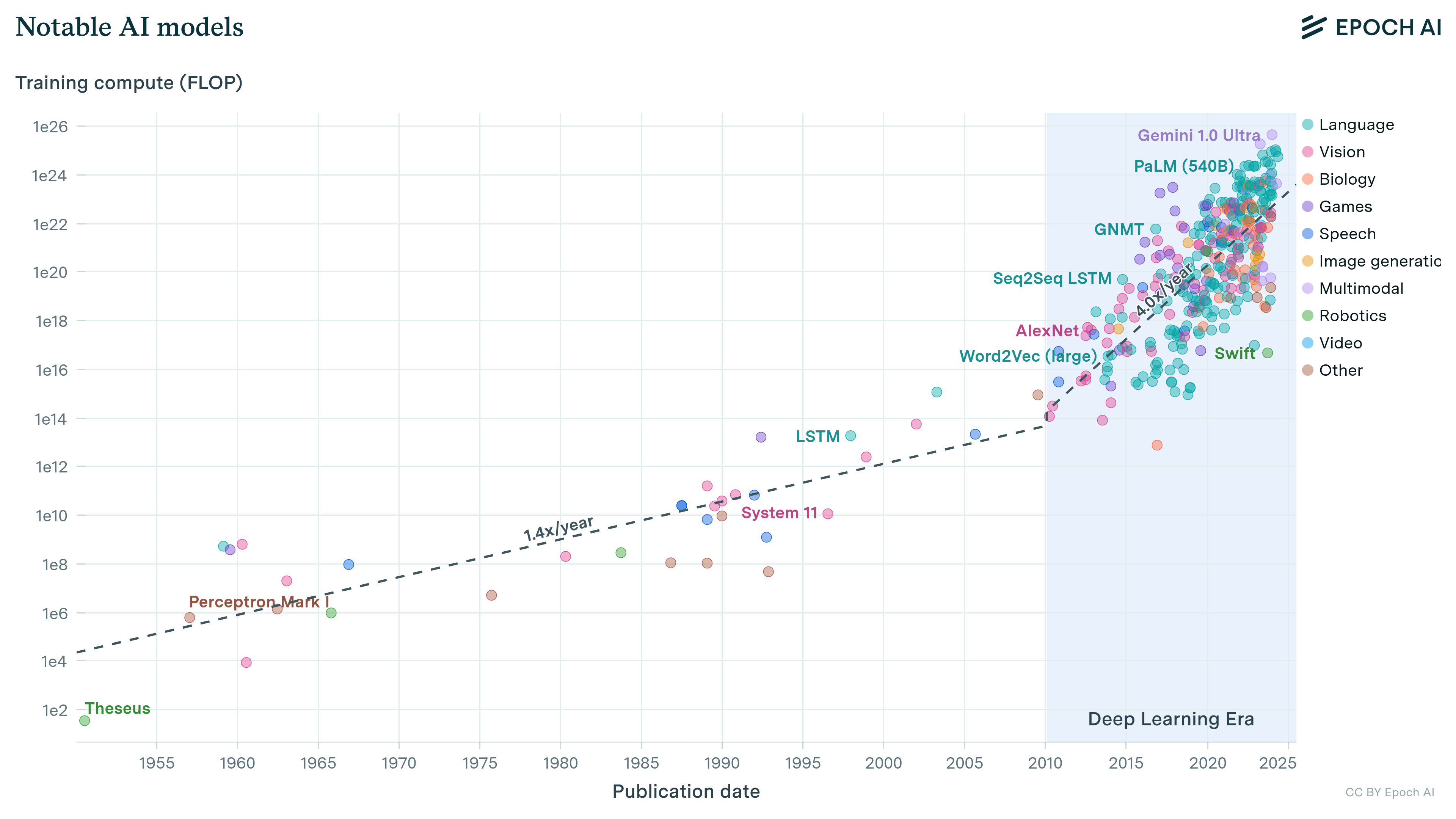
paper
· 7 min read
Compute Trends Across Three Eras of Machine Learning
We’ve compiled a comprehensive dataset of the training compute of AI models, providing key insights into AI development.
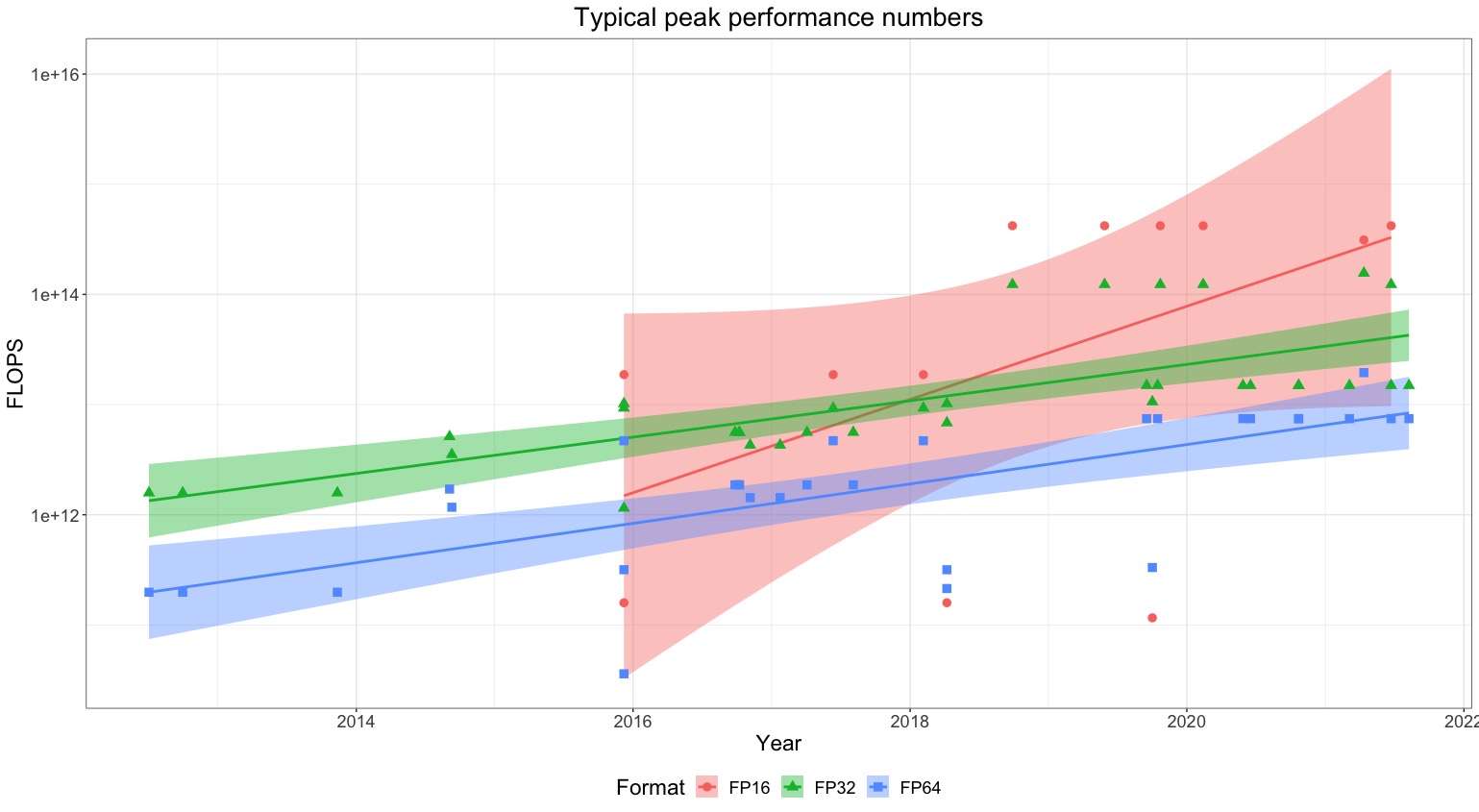
report
· 24 min read
Estimating Training Compute of Deep Learning Models
We describe two approaches for estimating the training compute of Deep Learning systems, by counting operations and looking at GPU time.
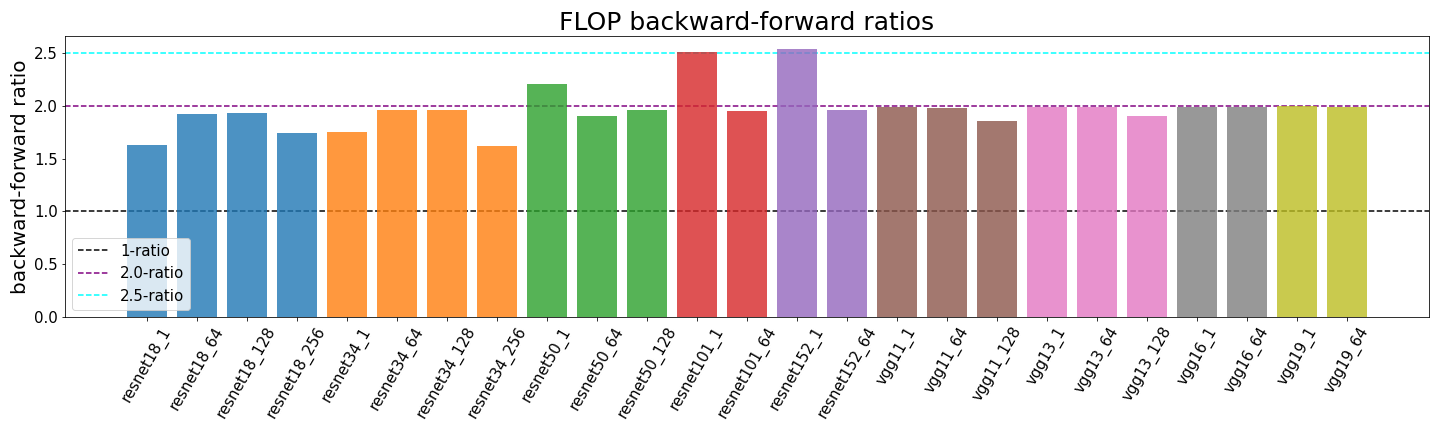
report
· 8 min read
What’s the Backward-Forward FLOP Ratio for Neural Networks?
Determining the backward-forward FLOP ratio for neural networks, to help calculate their total training compute.
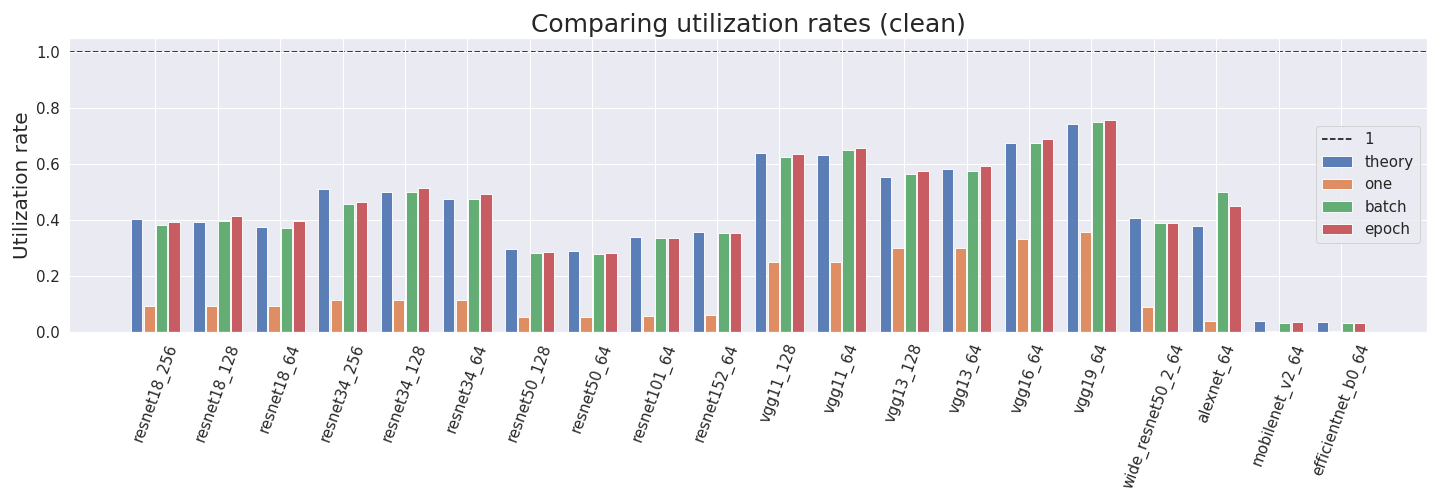
report
· 9 min read
How to Measure FLOP for Neural Networks Empirically?
Computing the utilization rate for multiple Neural Network architectures.