Introducing Epoch AI's AI Benchmarking Hub
We are launching the AI Benchmarking Hub: a platform presenting our evaluations of leading models on challenging benchmarks, with analysis of trends in AI capabilities.
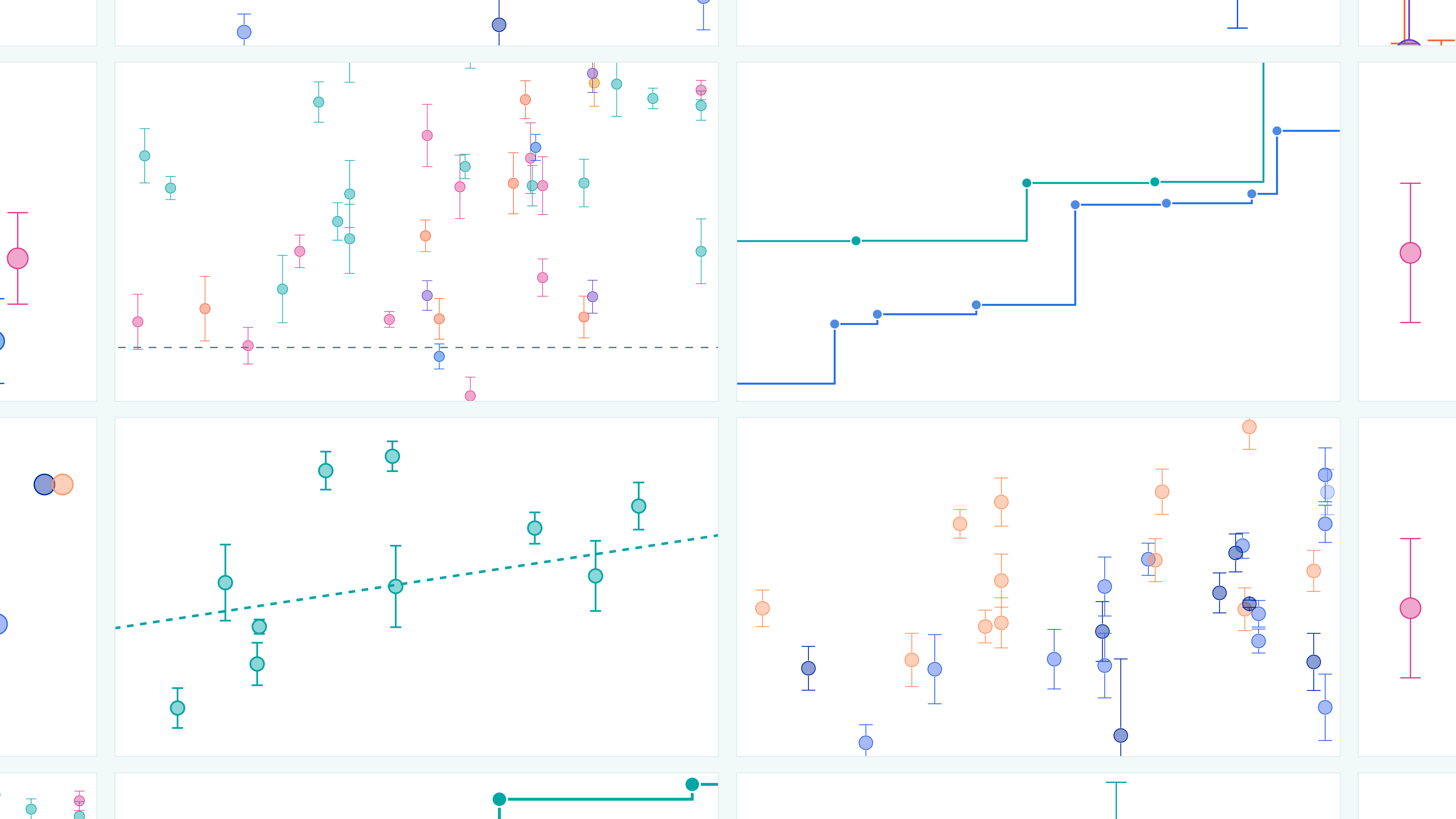
Published
Epoch AI is launching our AI Benchmarking Hub—a platform for comprehensively understanding AI capabilities.
By evaluating leading AI models ourselves and carefully analyzing the results, we aim to shed light on the main trends in AI capabilities. Our clear visuals and detailed findings can help researchers, developers, and decision-makers better understand what today’s AI systems can actually do—and where they’re headed.
Key Features of the AI Benchmarking Hub
Challenging benchmarks: Our goal is to track model performance on the hardest and most informative benchmarks. For this first release, the hub features results from two benchmarks:
- GPQA Diamond: This is a higher-quality, challenging subset of the GPQA benchmark, which tests models’ ability to answer PhD-level multiple choice questions about chemistry, physics, and biology.
- MATH Level 5: This is a subset of the hardest questions from the MATH benchmark, a dataset of high-school level competition math problems.
We plan to rapidly expand our suite of benchmarks to create a thorough picture of AI progress, by adding benchmarks such as FrontierMath, SWE-Bench-Verified, and SciCodeBench.
Independent evaluations: The AI community often relies on claims from AI labs about their models’ capabilities. Our AI Benchmarking Hub offers an independent perspective, helping stakeholders understand progress in the field through rigorous, standardized evaluations.
Supporting Deeper Analysis: Users can explore and visualize relationships between model performance and various characteristics of the models themselves. We also provide downloadable data and detailed metadata for all benchmark results, enabling users to conduct their own analyses. The hub makes it easy to understand how factors like training compute, model accessibility, and model developer relate to benchmark performance.
What’s Next?
More Benchmarks: We are adding more benchmarks to the hub, such as FrontierMath, SWE-Bench-Verified, and SciCodeBench. Our goal is to build a comprehensive suite of benchmarks that provides an accurate picture of the pace of AI advancement.
More Detailed Results: We will display even more information about benchmark results, such as model reasoning traces for individual questions. We want to raise the standards for how evaluations are conducted, leading by example.
Expanded Model Coverage: We’ll continue adding benchmark results for more models, including new leading models as they get released.
Scaling behaviour: We want to show in more detail how performance improves with scale, both the scale of pretraining compute and test-time resources.
We hope that the AI Benchmarking Hub will be a valuable resource for anyone interested in understanding and tracking AI progress. Stay tuned for regular updates as we continue to expand our benchmark suite and model coverage. Click here to start exploring!