Data on AI
Epoch AI collects key data on machine learning models from 1950 to the present to analyze historical and contemporary progress in AI. Our databases are a valuable resource for policymakers, researchers, and stakeholders to foster responsible AI development and deployment.
Explore our data
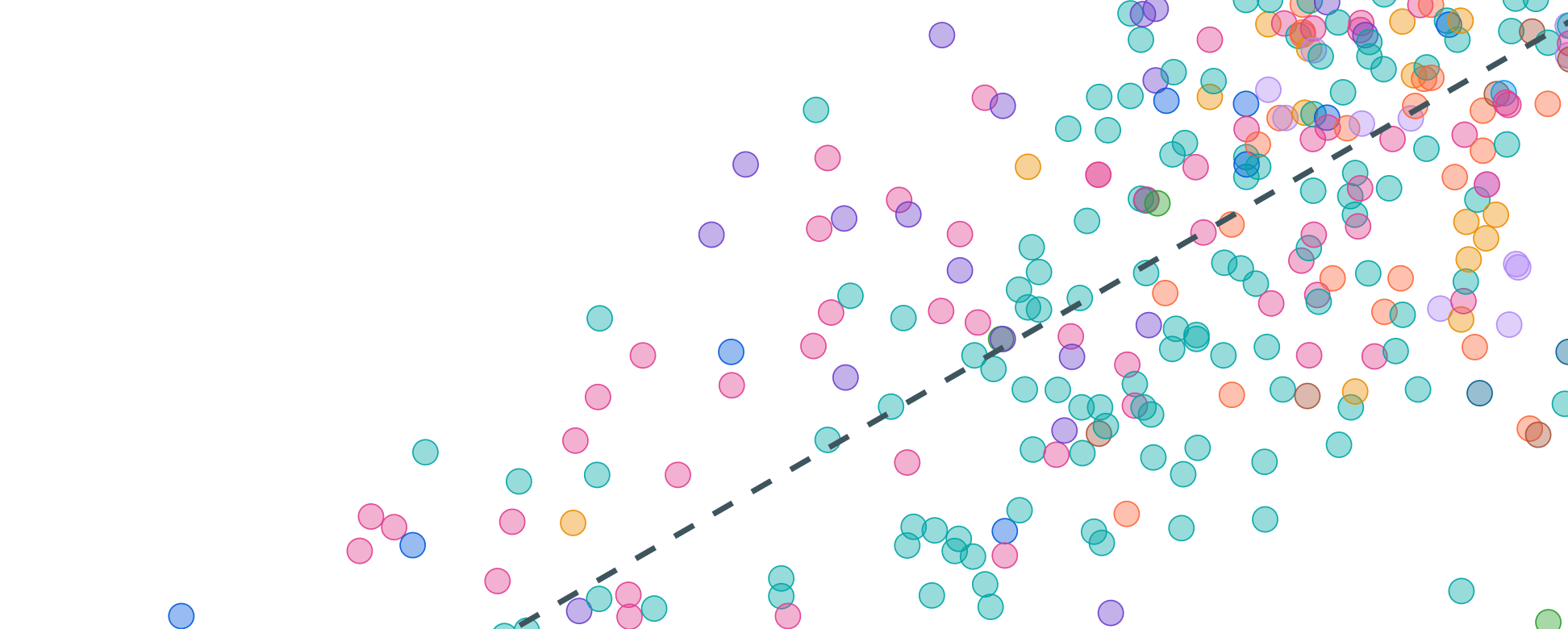
Notable AI Models
Our most comprehensive database, containing over 800 models that were state of the art, highly cited, or otherwise historically notable. It tracks key factors driving machine learning progress and includes over 400 training compute estimates.
Updated November 13, 2024
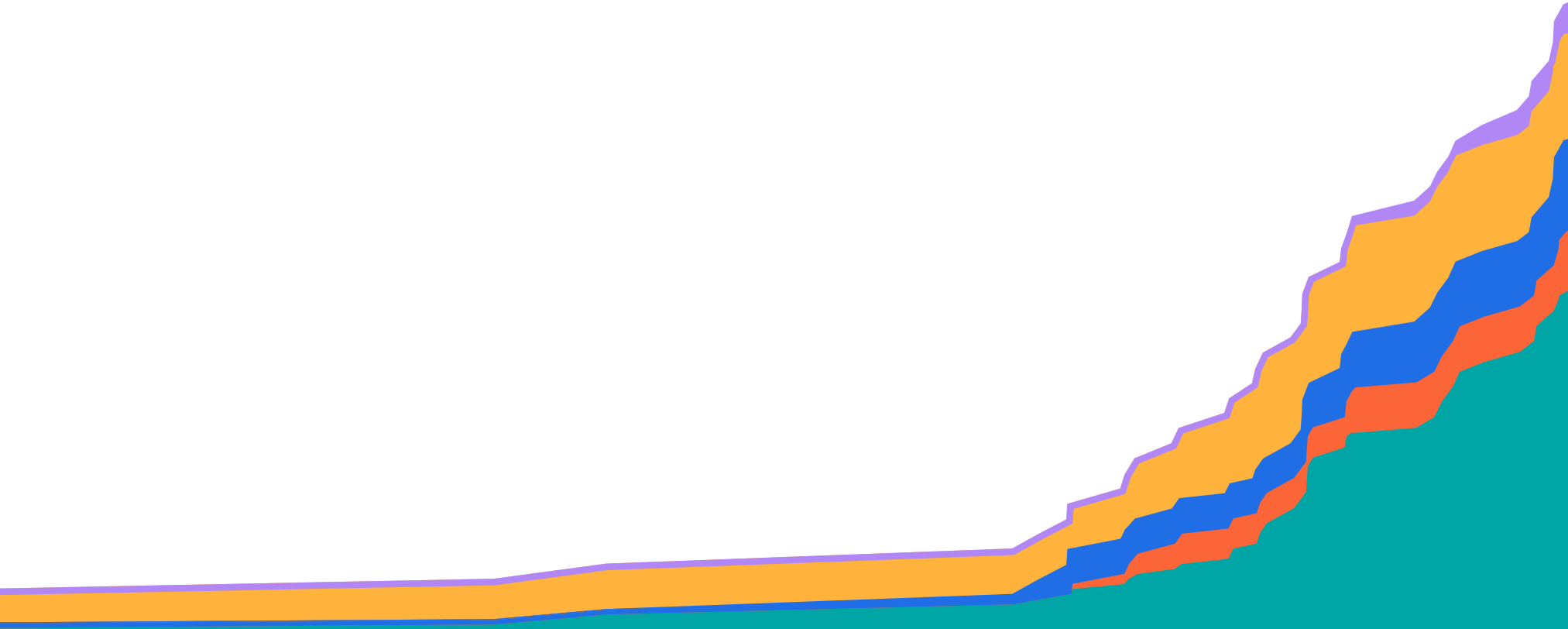
Large-Scale AI Models
Our Large-Scale AI Models database documents over 200 models trained with more than 1023 floating point operations, at the leading edge of scale and capabilities.
Updated November 13, 2024
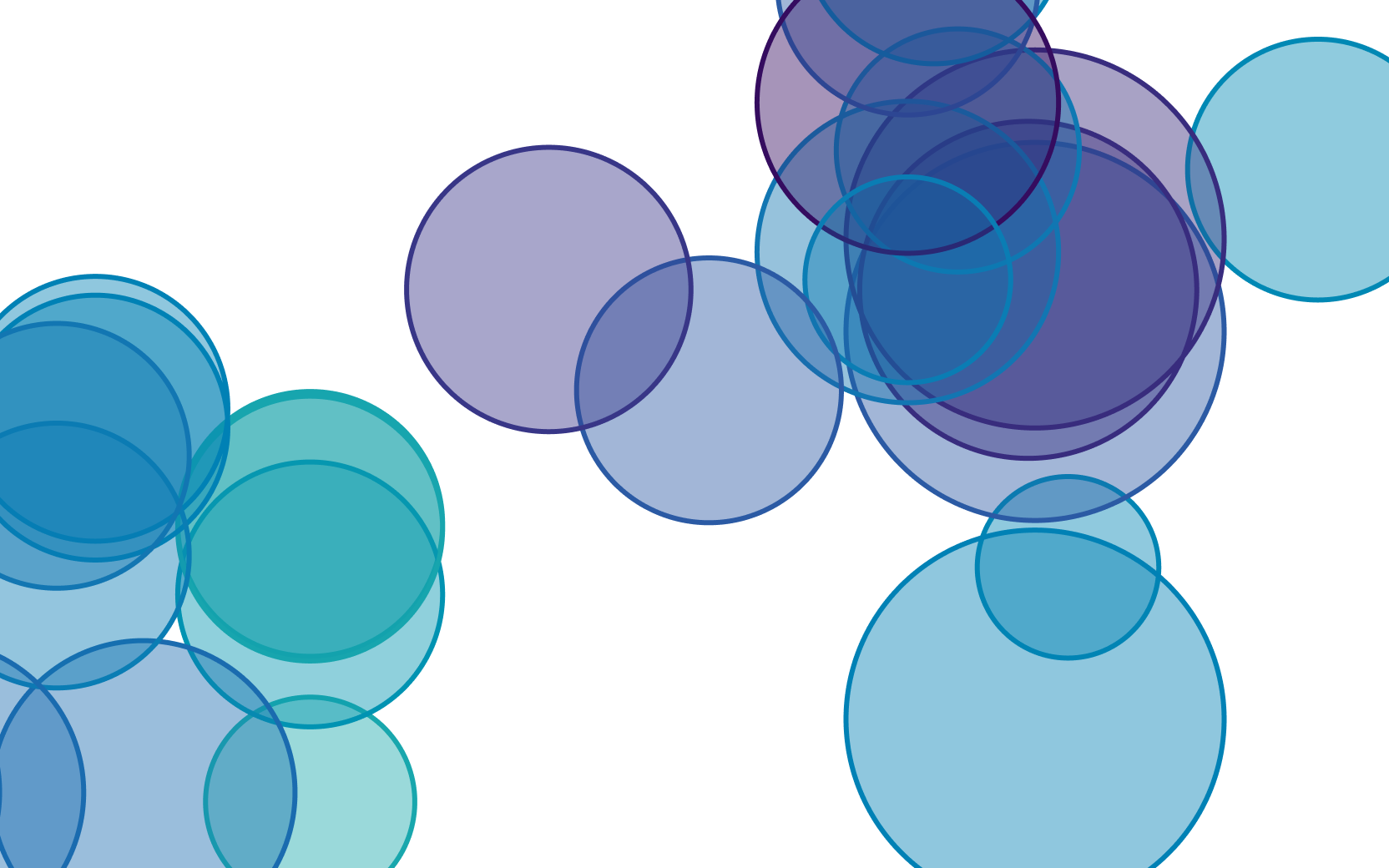
Machine Learning Hardware
We present key data on over 100 AI accelerators, such as graphics processing units (GPUs) and tensor processing units (TPUs), used for developing and deploying machine learning models in the deep learning era.
Updated November 13, 2024
Use our work
Epoch AI’s data is free to use, distribute, and reproduce provided the source and authors are credited under the Creative Commons Attribution license. Citations can be found on the respective pages for each database.
Download our data
Notable AI Models
CSV, Updated November 13, 2024
Large-Scale AI Models
CSV, Updated November 13, 2024
Machine Learning Hardware
CSV, Updated November 13, 2024
Our methodology
We identify and track contemporary and historic advances in AI, collating key details across several areas. This research includes who developed models, when, and for what tasks, how much compute was used for training, how many parameters models have, how much data was used for training, what hardware was used for training, and more.
Our research
Epoch AI is a multidisciplinary research institute investigating the trajectory and impact of artificial intelligence.
We publish datasets, data visualizations, research reports, and predictive models to analyze the forces shaping AI development. Our goal is to foster scientific dialogue and bring empirical rigor to predictions about the future of AI.