Announcing Epoch AI’s Updated Parameter, Compute and Data Trends Database
Our expanded database, which tracks the parameters, datasets, training compute, and other details of notable machine learning systems, now spans over 700 notable machine learning models.
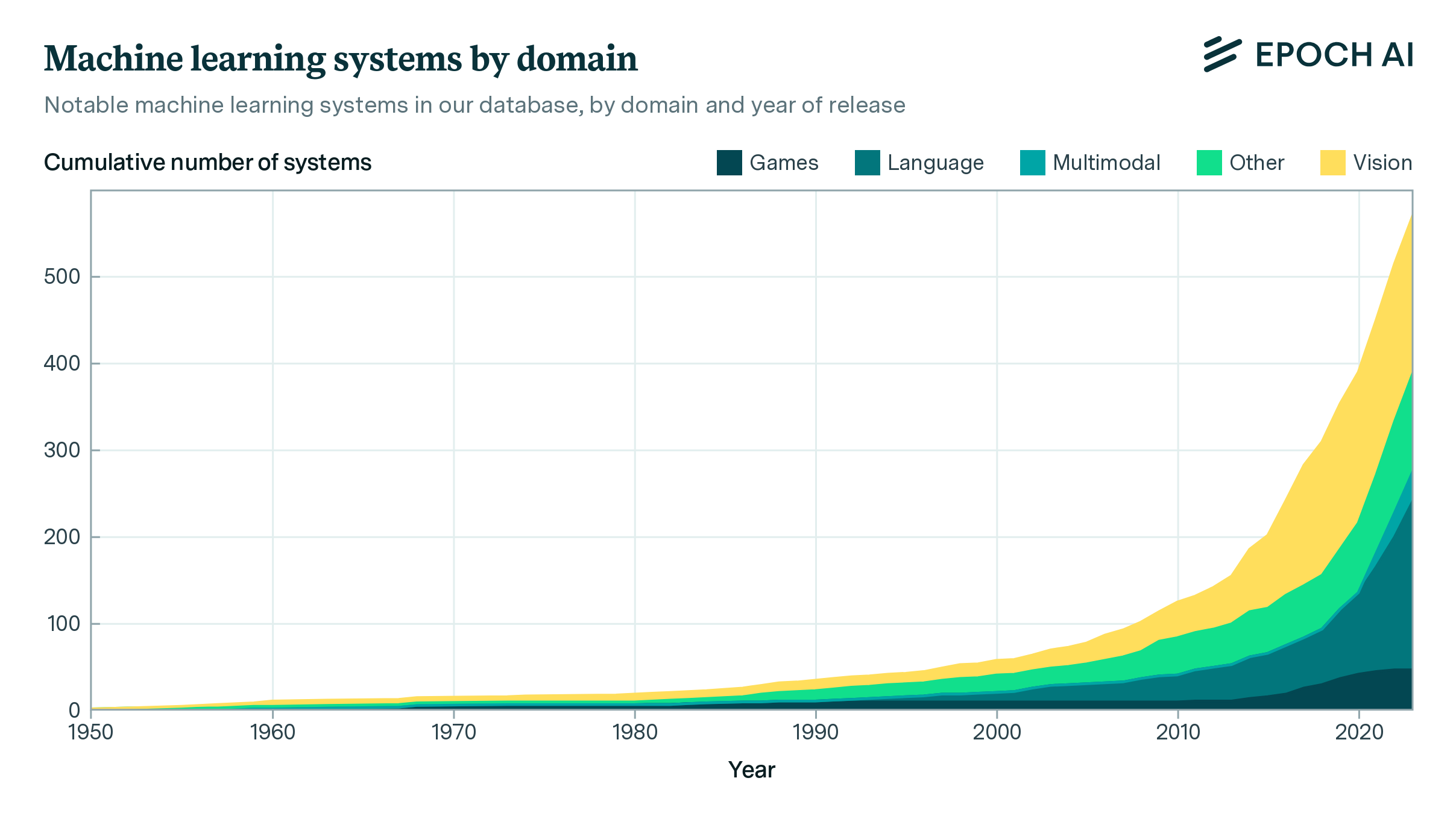
Published
Resources
Machine learning is advancing at breakneck speed, but what’s driving its progress? It is widely recognized that the performance of machine learning models is closely related to the amount of training data, compute, and number of parameters in the model. At Epoch AI, we’re investigating the key inputs that enable today’s AIs to reach new heights.
Our recently expanded Parameter, Compute and Data Trends database traces these details for hundreds of landmark ML systems and research papers. Our database allows everyone to understand:
- How models have swelled from mere dozens of parameters in early networks to over half a trillion in systems like Minerva today.
- How training compute has increased by nearly 8 orders of magnitude from 2012’s AlexNet to 2023’s GPT-4.
- How datasets have grown by billions of times, from 200 thousand words for early language models to the 1.9 trillion words used to train Flan-PaLM.
Building on a model and parameter dataset we first introduced in 2021, we’ve newly collected or edited data for over 400 systems, enriching the records with extra details. New entries include frontier AI models such as PaLM 2 and GPT-4, now in the dataset alongside historic breakthroughs such as convolutional networks, transformers, and LSTMs. In the past six months, we’ve added 240 new language models and 170 compute estimates. We will be maintaining this dataset, updating it with more historical information, and adding new significant releases.
Our data has been used in leading publications including The Economist, Time, and Our World in Data as well as research from Anthropic and the Brookings Institution, among others. We invite you to join them: check out the documentation, explore the interactive visualization, and access the data for your own research at https://epoch.ai/data/notable-ai-models?view=table#explore-the-data.