Trends in AI Supercomputers
AI supercomputers double in performance every 9 months, cost billions of dollars, and require as much power as mid-sized cities. Companies now own 80% of all AI supercomputers, while governments’ share has declined.
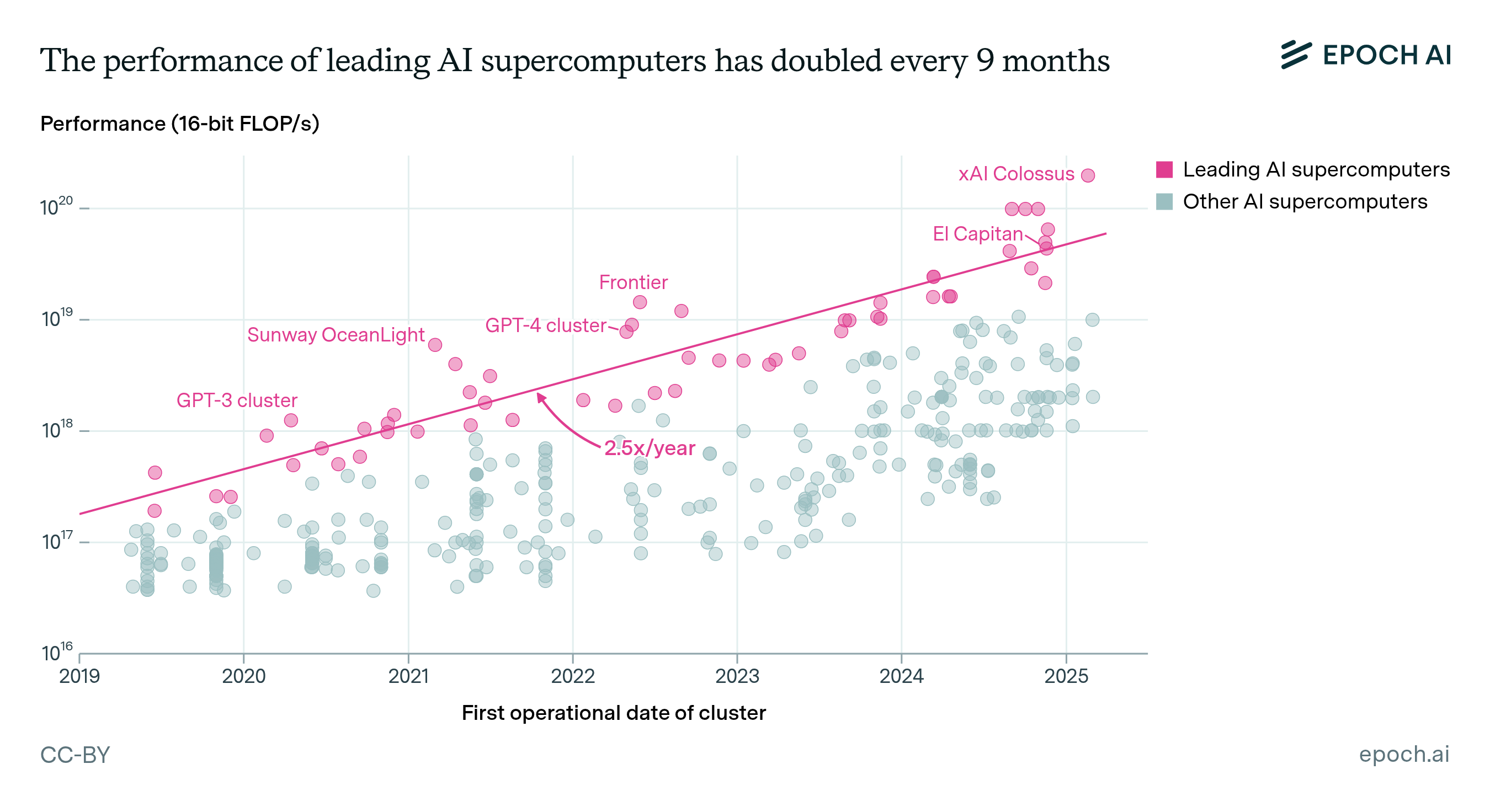
Published
Resources
Frontier AI development relies on powerful AI supercomputers. To train AI models with exponentially more compute, companies have developed massive systems like xAI’s Colossus, that contain up to 200,000 specialized AI chips, cost billions of dollars to build, and require hundreds of MW of power—equivalent to a medium-sized city.
However, public data on these systems is limited.
We curated a dataset of over 500 AI supercomputers (sometimes called GPU clusters or AI data centers) from 2019 to 2025 and analyzed key trends in performance, power needs, hardware cost, and ownership. We found:
- Computational performance grew 2.5x/year, driven by using more and better chips in the leading AI supercomputers.
- Power requirements and hardware costs doubled every year. If current trends continue, the largest AI supercomputer in 2030 would cost hundreds of billions of dollars and require 9 GW of power.
- The rapid growth in AI supercomputers coincided with a shift to private ownership. In our dataset, industry owned about 40% of computing power in 2019, but by 2025, this rose to 80%.
- The United States dominates AI supercomputers globally, owning about 75% of total computing power in our dataset. China is in second place at 15%.
We discuss these findings further below, or you can read our full research paper here.
Computational performance, energy, and cost trends
The computational performance of leading AI supercomputers has doubled every 9 months, driven by deploying more and better AI chips (Figure 1). Two key factors drove this growth: a yearly 1.6x increase in chip quantity and a yearly 1.6x improvement in performance per chip. While systems with more than 10,000 chips were rare in 2019, several companies deployed AI supercomputers more than ten times that size in 2024, such as xAI’s Colossus with 200,000 AI chips.
Figure 1: The performance of leading AI supercomputers (in FLOP/s, for 16-bit precision) has doubled every 9 months (a rate of 2.5x per year).
Power requirements and hardware costs of leading AI supercomputers have doubled every year. Hardware cost for leading AI supercomputers has increased by 1.9x every year, while power needs increased by 2.0x annually. As a consequence, the most performant AI supercomputer as of March 2025, xAI’s Colossus, had an estimated hardware cost of $7 billion (Figure 2) and required about 300 megawatts of power—as much as 250,000 households. Alongside the massive increase in power needs, AI supercomputers also became more energy efficient: computational performance per watt increased by 1.34x annually, which was almost entirely due to the adoption of more energy-efficient chips.
Figure 2: Cost of AI supercomputers over time, in 2025 USD.
If the observed trends continue, the leading AI supercomputer in June 2030 will need 2 million AI chips, cost $200 billion, and require 9 GW of power. Historical AI chip production growth and major capital commitments like the $500 billion Project Stargate suggest the first two requirements can likely be met. However, 9 GW of power is the equivalent of 9 nuclear reactors, a scale beyond any existing industrial facilities. To overcome power constraints, companies may increasingly use decentralized training approaches, which would allow them to distribute a training run across AI supercomputers in several locations.
Locations and public/private sector share of AI supercomputers
Companies now dominate AI supercomputers. As AI development has attracted billions in investment, companies have rapidly scaled their AI supercomputers to conduct larger training runs. This caused leading industry systems to grow by 2.7x annually, much faster than the 1.9x annual growth of public sector systems. In addition to faster performance growth, companies also rapidly increased the total number of AI supercomputers they deployed to serve a rapidly expanding user base. Consequently, industry’s share of global AI compute in our dataset surged from 40% in 2019 to 80% in 2025, as the public sector’s share fell below 20% (Figure 3).
Figure 3: Share of aggregate AI supercomputer performance owned by the private sector vs the public sector (governments and academia). Private-public means a partnership between the two sectors.
The United States hosts 75% of AI supercomputers, followed by China. The United States accounts for about three-quarters of global AI supercomputer performance in our dataset, with China in second place with 15% (Figure 4). Meanwhile, traditional supercomputing powers like the UK, Germany, and Japan now play marginal roles in AI supercomputers. This shift reflects the dominance of large, U.S.-based companies in AI development and computing. However, AI supercomputer location does not necessarily determine who uses the computational resources, given that many systems in our database are available remotely, such as via cloud services.
Figure 4: Share of AI supercomputer computational performance by country over time. Our dataset covers an estimated 10–20% of global aggregate AI supercomputer performance as of March 2025. While coverage varies across companies, sectors, and hardware types due to uneven public reporting, we believe the overall distribution remains broadly representative. Future country shares may change dramatically as exponential growth continues in both AI chip performance and production volume. We are visualizing all countries that held at least a 3% share at some point in time.
Our dataset
Our dataset and documentation have been released in Epoch AI’s Data on AI hub and will be maintained with regular updates.
You can read our paper here.