Who Is Leading in AI? An Analysis of Industry AI Research
Industry emerged as a driving force in AI, but which companies are steering the field? We compare leading AI companies on research impact, training runs, and contributions to algorithmic innovations.
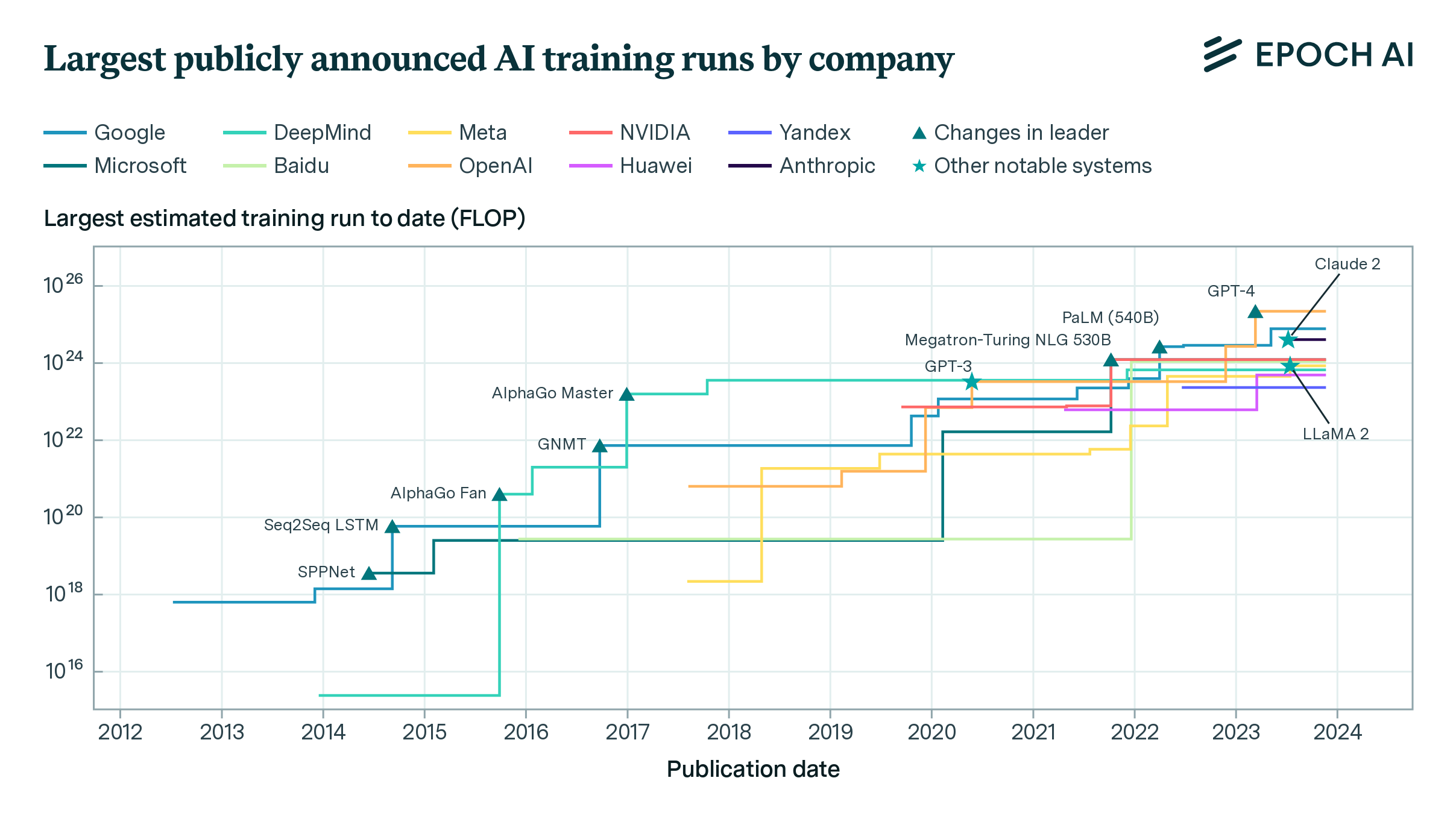
Published
Resources
The private sector’s pivotal role in AI research and development is marked by its substantial resource investments and significant influence, evidenced by higher citation rates for industry-involved research articles and dominance in compute-intensive training runs. Recent analyses, such as the 2023 Stanford AI Index, highlight the varying research impacts of institutions across countries, with US tech giants leading in terms of research impact. Our study is informed by three datasets—OpenAlex for AI publications, the PCD database for AI training compute data, and a new dataset on key algorithmic innovations in large language models. We offer a comprehensive comparison of leading companies in publications, citations, unique authors, training runs, and algorithmic innovation adoption. This multi-faceted approach provides a nuanced understanding of industry’s influence on AI development, contributing to policy discussions on key industry players.
You can read the full paper here.
Key results
Bibliometric output and impact. Our analysis shows that Google and Microsoft lead in total publications and citations over the past 13 years, reflecting their size and incumbency advantages. However, OpenAI, Meta, and DeepMind (now part of Google DeepMind) have higher citation rates per publication than other industry players. In contrast, Chinese firms such as Tencent, Baidu, and Huawei have lower citation impacts per author, with SenseTime as an exception, outperforming Microsoft but not DeepMind in citations-per-author.
Compute. Our findings reveal a trend among leading U.S. companies, including Google, OpenAI, and Meta, towards rapidly scaling up their training compute. Specifically, Google’s training compute grew approximately 10 million-fold over 11 years, while OpenAI and Meta each expanded by around one million-fold within six years. This growth far exceeds the general trend in ML systems, which saw a four thousand-fold increase over the same period.
From 2012 to 2023, the field of compute-intensive training runs in AI has seen significant shifts. Initially dominated by Google’s AI labs in the 2010s, OpenAI, founded in 2015, quickly matched and even surpassed Google DeepMind’s compute levels, especially with its GPT-4 model at an estimated 2e25 FLOP. OpenAI’s rapid progress was facilitated by a partnership with Microsoft in 2019. Anthropic, a newer player, also made an impact with Claude 2, nearing the compute scale of Google’s PaLM 2. Meta, however, has remained behind the leaders, with its closest gap being 6-fold lower than the frontier in May 2022.
Innovations. We examined the adoption of algorithmic innovations in the 10 publicly documented language models with the most training compute, including models like PaLM and LLaMA. These innovations are crucial in ML research, and their frequency of adoption offers insights into research impact. Our analysis shows that Google’s innovations, notably the Transformer and LayerNorm, are most widely adopted in recent foundational models. OpenAI’s contributions, including advancements in in-context learning and instruction tuning, are adopted about a third as frequently as Google’s, followed by Meta and DeepMind.
Policy implications
Keeping tabs on frontier AI labs. The leadership of Google, OpenAI and Meta emphasizes their importance when crafting AI policy. But new AI labs can catch up quickly, as was the case for OpenAI and especially Anthropic. It is therefore important to closely track the progress of emerging AI labs as well as current leaders.
Assessing national AI leadership. Chinese industry labs lag their US counterparts in all our metrics. These metrics may not capture all dimensions of AI development—for example, Chinese labs may intentionally put less priority on publication in academic venues. Nevertheless, it seems almost certain that Chinese labs are trailing US labs in the development of general-purpose foundation models, and have had less impact on academic AI research in general.
As governments increase oversight of AI companies, our paper provides key evidence about which companies are steering the field. We are excited about building on this work, especially to further our understanding of key algorithmic innovations.