2023 Impact Report
In 2023, Epoch published almost 20 reports on developments in AI, added hundreds of new models to our database, had a direct impact on government policies, raised over $7 million in funds, and more.
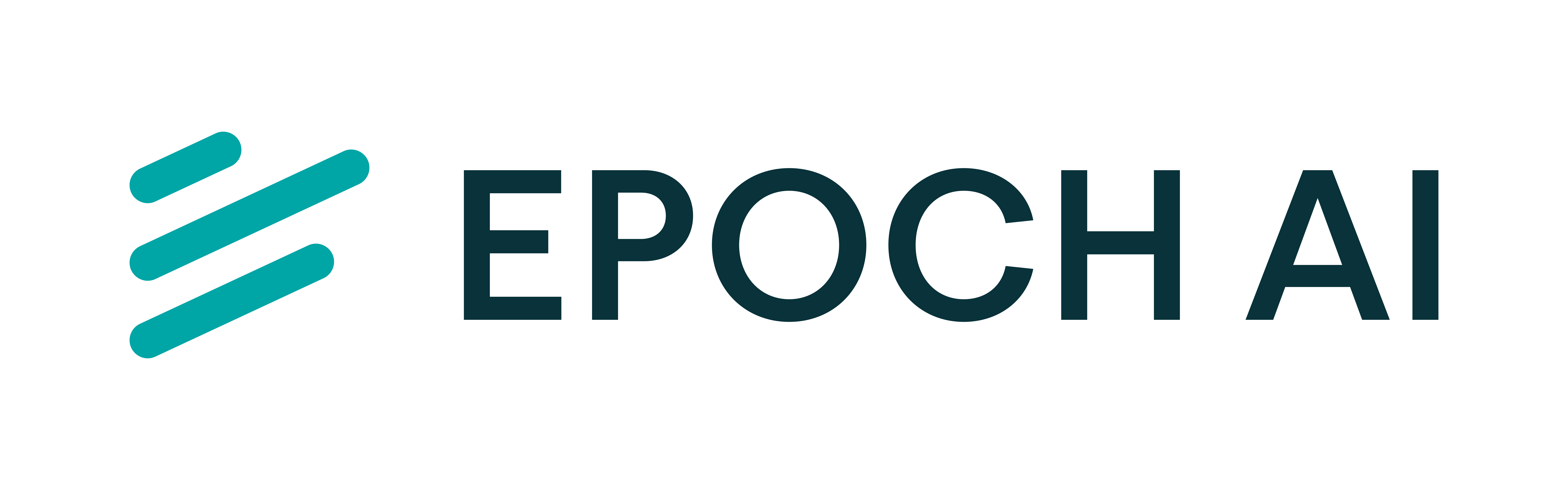
Published
About Epoch AI
Epoch AI is a multidisciplinary research institute investigating the trajectory of Artificial Intelligence (AI). We produce papers and reports on the drivers, trajectory and consequences of the development and deployment of AI.
In the past, we have produced cutting-edge AI forecasting work such as Compute Trends Across Three Eras of Machine Learning (Sevilla et al., 2022), Revisiting Algorithmic Progress (Besiroglu and Erdil, 2022) and Will We Run Out of ML Data? Evidence From Projecting Dataset Size Trends (Villalobos et al., 2022). We also maintain a database of notable ML models, widely regarded as the most comprehensive public database of its kind in existence.
Testimonials
Epoch does the most thoughtful and best-researched survey work in the industry. Several times I have thought I found errors in their results, only to discover when going through their notebooks that they had it right. They are my go-to resource for field-wide trends.
I feel like I wouldn't have nearly as good an understanding of the important trends in AI and their future implications without Epoch.
This year and future goals
In 2023, we continued our research on the trajectory of AI and its potential impacts. We produced almost twenty research reports, covering trends in ML hardware, scaling, and algorithmic improvements, as well as AI’s potential impact on automation and economic growth. We also updated and maintained our database of notable ML models, adding hundreds of new models.
We’ve also had many accomplishments this year outside of research. We successfully engaged with policymakers, media, and the AI industry to help share our ideas. We also raised over $7 million in funds, made multiple hires, updated our website to improve its style and our data visualizations, and ran two mentorship programs.
Next year, we intend to continue our research into the drivers of AI and on AI’s effect on economic growth. We will also work to strengthen the organization by diversifying our funding sources, developing our relationships with governments, and improving our headhunting capabilities.
Summary of key findings
ML trends
- We analyze trends in the performance of ML hardware and find:
- Lower-precision number formats on specialized hardware can improve performance in ML workloads by an order-of-magnitude compared to the traditional FP32 format. More.
- Computational performance in FLOP/s has doubled every 2.3 years for both general and ML-specific GPUs. Computational price performance in FLOP/$ has doubled every 2.1 years for ML GPUs and 2.5 years for general GPUs. Energy efficiency in FLOP/s/W has doubled every ~3 years. More.
- Memory capacity and bandwidth has doubled much more slowly, every ~4 years. More.
-
The recent US Executive Order on AI establishes reporting requirements for AI models. We analyze the current data on models trained on biological data, finding one protein language model exceeds the mandated threshold, and ambiguity around models fine-tuned on biology data or trained on mixed data. More.
-
We investigate the output from key industry AI labs, finding that Google, OpenAI, and Meta are leading AI industry research across citations, size of training runs, and key algorithmic innovations that underpin large language models. Leading Chinese companies such as Tencent and Baidu have a markedly lower impact on all of these metrics. More.
Algorithmic improvements
- Several techniques can improve the performance of trained large language models by an amount equivalent to increasing training compute by 1-2 orders of magnitude. These techniques incur little additional computational cost (<10% of pre-training compute, and often <1%). More.
-
It is also possible to improve the performance of trained models by an amount equivalent to increasing training compute by 1-2 orders of magnitude, at the cost of increasing inference compute by 1-3 OOM. More.
-
Model recycling via kickstarting or model augmentation likely only provides modest benefits in reducing foundation model training costs. More.
Economics of AI
- Leading theories of economic growth suggest that AI that could substitute for human workers in most tasks could lead to a ten-fold increase in economic growth in frontier economies. Key questions remain about the intensity of regulatory responses to AI, physical bottlenecks in production, the economic value of superhuman abilities, and the rate at which AI automation could occur. More.
- Predicting which occupational tasks will be automated by AI is an important but unsolved challenge. We’ve found substantial disagreement between the predictions made by different approaches. The field has significant room for improvement, such as in making realistic deployment assumptions and linking predictions to measurable AI progress. More.
Other topics
- We estimate the maximum energy efficiency of CMOS microprocessors, measured in FLOP per Joule of energy dissipated (FLOP/J). We estimate a roughly 50% chance that CMOS processors will hit an energy efficiency ceiling at ~6e13 to 1.5e15 FLOP/J (using 16-bit precision), which would be a ~200x improvement on the existing state-of-the-art. More.
- We use a novel, speculative methodology to estimate the training requirements of AI that can substitute for most economically useful tasks, and arrive at an estimate of between 1e27 and 1e41 effective FLOP (i.e. adjusting for algorithmic progress starting from 2024). More.
Overview of research projects
We produced 19 research outputs in 2023 after our previous impact report was written, including papers, reports, databases and dashboards. These cover many crucial topics for the future of AI, including trends in machine learning, algorithmic progress, the economics of AI and more.
Category | Type | Title | One sentence description |
---|---|---|---|
ML Trends | Report | Trends in the Dollar Training Cost of Machine Learning Systems | Estimates compute costs in dollars of major training runs between 2009 and 2022. |
ML Trends | Dashboard | Key trends and figures in machine learning | Features key numbers and data visualizations about AI, relevant Epoch AI reports, and other sources that showcase the change and growth in AI over time. |
ML Trends | Paper | Power Laws in Speedrunning and Machine Learning | Investigates how to model the advances of benchmarks over time. We find evidence in ML that 1) benchmarks haven’t saturated and 2) sudden improvements are uncommon but have precedent. |
Training requirements | Report | Direct approach | Estimates the amount of compute required to build AI that can reliably accomplish tasks of a certain length. |
ML Trends | Viewpoint | Please Report your Compute
(published in the Communications of the ACM) |
Argues for transparency around the training compute used to develop AI models. |
ML Trends | Viewpoint | A Compute-Based Framework for Thinking About the Future of AI | Argues that compute is the key indicator for anticipating future progress in AI. We discuss timelines of development under this light. |
ML Trends | Model | Direct approach interactive model | Combines extrapolations of the trends we have investigated to produce a naive estimate of when to expect transformative AI. |
Scaling laws | Report | How Predictable Is Language Model Benchmark Performance? | Studies trends in language model performance, showing that compute-focused extrapolations are a promising way to forecast AI capabilities. |
Algorithmic progress | Report | The Limited Benefit of Recycling Foundation Models | Estimates the benefits of recycling old foundation models on saving costs in future training runs, finding a limited benefit. |
Algorithmic progress | Report | Trading off compute in training and inference | Investigates the degree to which additional compute during inference can be spent to improve performance of trained models. |
Economics of AI | Paper | Explosive Growth from AI: A Review of the Arguments | Reviews theories of economic growth and empirical arguments regarding the potential of advanced AI substantially accelerating economic growth. |
ML Trends | Dataset | Updated Parameter, Compute, and Data Trends database | Expanded the database to over 800 ML systems, added performance benchmark data, and checked the accuracy of the existing data. |
ML Trends | Report | Trends in Machine Learning Hardware | Analyzes recent trends in ML hardware performance, focusing on metrics such as computational performance, memory, interconnect bandwidth, price-performance, and energy efficiency across different GPUs and accelerators. |
Economics of AI | Report | Challenges in predicting AI automation | A literature review summarizing and comparing existing approaches to predict automation of occupational tasks. |
ML Trends | Paper | Who is leading in AI? An analysis of industry AI research | Compares leading AI companies by research publications, citations, size of training runs, and contributions to key algorithmic innovations. |
Algorithmic progress | Paper | AI capabilities can be significantly improved without expensive retraining | In collaboration with Open Philanthropy and UC Berkeley, compiles a list of techniques that improve the performance of trained models, quantifying their costs and benefits. |
Hardware | Paper | Limits to the Energy Efficiency of CMOS Microprocessors
(accepted for publication at IEEE International Conference on Rebooting Computing) |
Estimates the limits of energy efficiency of CMOS microprocessors to yield bounds on amounts of computation that can be done at various energy budgets, e.g. 2023 global primary energy consumption levels. |
ML Trends | Report | Biological sequence models in the context of the new AI directives | Analyzes the recent White House Executive Order’s oversight of biological sequence models, providing context on models affected, ambiguities in the order, and trends in data usage and access. |
Overview of non-research activities
Besides our impressive research outputs, we are continuously investing into our organization.
Fundraising
We have raised 7 million USD to cover our expenses through September 2025 through a couple of grants from Open Philanthropy. We also received a notable $100,000 donation from Carl Shulman, and several minor donations from individuals.
We are actively putting efforts into diversifying our funding, such as applying for an NSF grant and initiating a sponsored collaboration with the Stanford AI Index. If you are interested in making a large donation, please contact us. We also appreciate individual contributions of any amount.
Hiring
We hired senior researcher David Owen, who has taken a prominent role in the organization through his research and management. We also hired data scientist Robi Rahman and multiple data contractors to scale up our existing data efforts.
Website
We have updated our website’s style, look and graphs, to better reflect the quality of our work. We plan to continue improving our visualizations to create quality graphs that help communicate our most important findings.
Mentorship Programs
We ran two mentorship programs, including a private mentorship program in March with students from MIT and Harvard, and a public mentorship program, in collaboration with the Forecasting Research Institute, aimed at women, non-binary people, and trans people of all genders.
Overview of impact
Media and scientific communication
Epoch AI continues to support better communication on AI. In particular, we have had some notable mentions of our work by media outlets, including TIME Magazine, The Economist, MIT Technology Review, and the New York Times. We also gave public talks about our work in Physics World, EAG London 2023, VPRO Tegenlicht and elsewhere.
Conferences and workshops
We frequently engage experts to receive feedback on our work. For instance, we participated in the 7th Annual Center for Human-Compatible AI Workshop, and the 2023 IEEE International Conference on Rebooting Computing.
We also have presented our work at the UVA Economics of AI seminar, MIT Economics of AI Tea, MIT Economics of AI seminar, AI Scaling workshop at MIT, the MIT FutureTech Lab, and elsewhere.
Mentions in industry, research and scientific publications
Epoch AI continues to be a relevant resource for developers and researchers at the cutting edge. We have had some notable mentions in scientific articles, industry communications, and books, including in François Fleuret’s The Little Book of Deep Learning, Google’s announcement of Bard and NVIDIA’s blog.
In total, our work has accumulated hundreds of citations in 2023 from academic and industry publications. Some papers that have received significant attention throughout the year are Compute Trends Across Three Eras of Machine Learning and Will We Run Out of ML Data? Evidence From Projecting Dataset Size Trends.
Engagement with policymakers
We have seen a surge of interest in AI policy in the past year. We have been able to support the ongoing conversation on managing AI technologies by providing up-to-date evidence on relevant topics.
In 2023, we supported the work of the UK Department for Science, Innovation and Technology and the Joint Research Centre of the European Commission through consultations and collaborations. In particular, the UK DSIT discusses our work on Frontier AI: capabilities and risks. We also submitted evidence to a House of Lords inquiry on language models.
Next year’s goals and funding needs
Research goals
Model of AI and growth
Throughout 2023, we have been actively developing a sophisticated model of AI and economic growth based on semi-endogenous growth theory, which will build upon the compute-centric model from Davidson (2023). This model takes an alternative modeling approach, incorporating more realistic dynamics of investment and several improvements over Davidson’s model.
We intend to release this model during the coming year, after a thorough process of review and testing. The release will consist of a whitepaper and an interactive model. We also plan on delivering several workshops among economists in government and academia to make the model widely accessible as a standard tool of analysis.
Drivers in AI
We plan to continue our research into the main drivers of AI, and possible bottlenecks the field might encounter in the future. This will encompass work on algorithms, overcoming data limitations, parallel training, the cost of training AI systems and more.
Data on machine learning
We plan to keep expanding our database of notable ML models, and in particular strive to comprehensively curate information on systems trained using large amounts of compute.
We also plan on developing a brand new database of innovations in ML, that will help support research into algorithmic progress.
Organizational goals
Stakeholder engagement
We foresee that interest in AI will continue surging in 2024, and that our input to policy will continue to be critical to informing decision-makers worldwide. To this end, we plan to develop our relationships with the US, UK and EU governments to provide evidence relevant for the regulation of AI.
Hiring
It is important to us to grow to accommodate new projects and to expand the expertise we have access to. We plan to hire 1-2 senior staff and 1-2 specialists, increasing our headcount to 14-16 FTE. We also want to improve our headhunting capabilities, and in particular our ability to recruit senior talent.
Project management
Over the last year we have taken significant steps towards improving the quality of our outputs, including a more thorough review process and more engagement with external experts. We want to keep improving our internal processes for project management and review to ensure the highest level of quality while keeping a high productivity. We aim to produce between 24 and 30 research outputs over the next year.
Diversifying our funding
We want to establish relationships with 1-2 additional funders to solidify the independence and continuity of the organization. This might include some commissioned work from stakeholders aligned with our core mission.
Website and brand
Continuing our process of rebranding, we want to strengthen our public image and the quality of our visualizations. We are in the process of hiring a full-time product and data visualization designer who will own this. Our goal is to have a recognizable style and produce graphs that help communicate our most important findings.