Challenges in Predicting AI Automation
Economists have proposed several different approaches to predicting AI automation of economically valuable tasks. There is vast disagreement between different approaches and no clear winner.
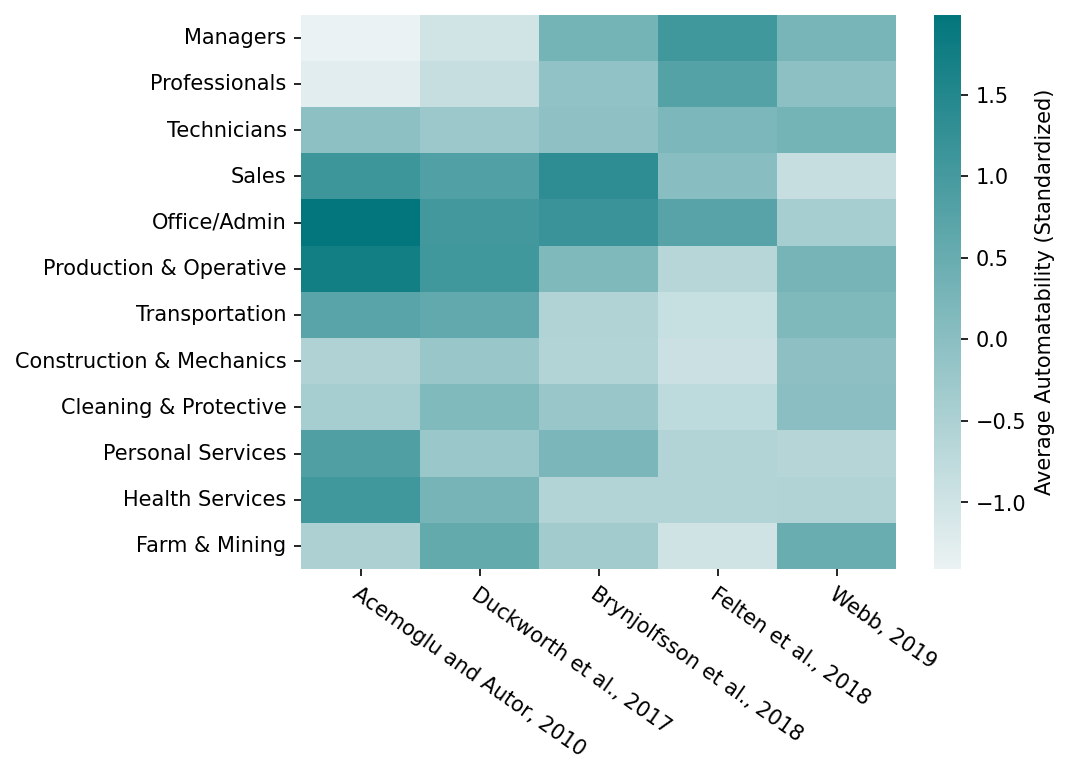
Published
Resources
Introduction
The automation of tasks by AI systems has the potential to generate tremendous economic value (Erdil and Besiroglu, 2023). The prospect of capturing this value can incentivize greater investments into developing AI capabilities. Accurately predicting when tasks are likely to be automated by AI could therefore help forecast the trajectory of AI investment and AI development. Understanding the impact of automation on the economy and labor force is also important for policymakers; governments may need to implement policies to help workers transition and ensure the benefits of automation are broadly shared.
This review examines the literature on predicting AI automation, focusing on the economics literature on AI-driven automation of occupational tasks. We also review the nascent literature on empirical validation of these predictions, examining whether we should put more trust in some predictions than others. We hope this review will help researchers engage with this important problem. We also hope that clarifying the challenges faced by existing predictions will surface promising directions for future work.
In the literature, there are three broad ways people have predicted automation:
- Task feature analysis to measure how susceptible tasks are to automation, typically informed by AI researchers’ opinions on AI capabilities, which are then linked to features of work tasks.
- Task-patent mapping, matching task descriptions to similar AI patents.
- Automation forecasting surveys directly asking AI experts when tasks or occupations will become automatable.
There are several challenges to existing methodologies for predicting automation. Most approaches focus on “in-principle-automatability” - scoring occupational tasks on how soon AI will have the technical capacity to automate them, while neglecting questions about costs and incentives, workflow reorganization, societal decisions, and other important factors. Interpretability is another important limitation. Many approaches do not distinguish between full and partial automation, making their results difficult to translate into well-defined predictions. Moreover, these methodologies, with the exception of forecasting surveys, do not provide concrete timelines - they instead provide ratings of relative automatability. Finally, some approaches rely on assessments of AI capabilities that have become outdated by subsequent progress, as discussed by Eloundou et al. (2023) and Felten et al. (2023). These limitations can be severe, and we discuss them further in Comparison of prediction methodologies.
Nevertheless, the field has made progress on the above challenges. There is even empirical evidence exploring how predictions compare to real world data. Several prediction methodologies for software and robotics automation have been partially validated by economy-wide occupational changes in hiring and wages. Focusing more narrowly on AI, there is early evidence that predictions correlate with firm-level changes in hiring. There are also AI-specific case studies showing productivity improvements from AI systems in domains such as customer support, translation, software engineering and management consulting. Taken together, these growing bodies of evidence may provide clues about how to predict automation from AI - and how to treat existing predictions.
Before reviewing prediction approaches in detail, we discuss the task-based framework used to model automation, which underpins them (How does automation happen?). Subsequently, we examine the purpose of automatability predictions, and the properties that we desire from prediction methodologies (What do we want from automation predictions?). We proceed to review the literature on automatability predictions, with a focus on their methodologies (An overview of automatability prediction methodologies). We then review the literature on empirical validation of these approaches, and examine the differences between them in a side-by-side comparison (Empirical evidence and comparison). Finally, we discuss open questions for future research, relating these to recent progress in both AI and the automation literature (Discussion).
How does automation happen?
In labor economics, it is common to study automation at the level of tasks associated with workers in given occupations or sectors, for example as described by Autor (2013). Task-level analyses can provide a more accurate and realistic model of automation than occupation-level analyses. For example, the work tasks of a secretary or software engineer have changed dramatically due to technology, but these occupations have not been automated in their entirety. The task-based approach builds upon the older “canonical model” that divided workers into low- and high-skilled groups without examining their individual tasks.
The concept of a task is defined very generally, for example in Autor (2013): “a unit of work activity that produces output”. Concrete examples offered are “moving an object, performing a calculation, communicating a piece of information, or resolving a discrepancy”. Much research on automatability has used the Occupational Information Network (O*NET) database of occupational information as a detailed source of occupations, tasks, and skills. The O*NET database describes work tasks at a range of granularities: a task is the most fine-grained description of work, often specific to a single occupation. Work activities are higher-level descriptions of more general work, often associated with many occupations.
There are broadly three ways of thinking about automation in a task-based framework:
- Full automation of tasks within jobs, i.e. technology being able to perform the task.
- Partial automation of tasks within jobs, i.e. improving workers’ productivity.
- Deskilling of tasks within jobs, i.e. reducing the skill requirement to perform a task.
Much of the literature on measuring automatability does not carefully distinguish between these three. This is in contrast to the literature on modeling automation’s effects, such as Acemoglu and Autor (2011), which considers these differences to be important. All of these can also see further technological progress, resulting in deepening automation: improvement of productivity on already-automated tasks as discussed in Acemoglu and Restrepo (2019). Relatedly, the practical cost of automation may vary with time: there is an important difference between a task that can be automated with large upfront and ongoing capital investment, and a task that can be automated with near-zero investment (Arntz et al., 2016).
An important feature in task-based analyses is that tasks can be adapted, destroyed or created due to technological and social change (Arntz et al., 2016; Acemoglu and Restrepo, 2019). This may happen naturally as a consequence of automation: when tasks can be automated cost-effectively, there are incentives to automate them. This often results in rearrangement of existing workflows around these tasks. Labor is reallocated to non-automated tasks, but with demands changed correspondingly: if automation increases productivity in a task, complementary non-automatable tasks will assume greater importance. This can be a significant challenge for recording data, modeling automation, and predicting automation trends. Nevertheless, this challenge is fundamental to a realistic view of automation.
What do we want from automation predictions?
Ideally, we would want to predict when particular occupational tasks will see a certain level of automation. We want to answer questions such as “When will AI improve productivity by X% on task T?” In practice, no existing methodology can yet answer such questions. Existing approaches instead tend to provide a general rating of tasks’ susceptibility to automation.
There are several properties we would want from automation predictions:
-
Interpretable predictions.
An automatability rating should be clearly related to the type and extent of automation, the timeframe or required AI capabilities, and the extent of task adaptation or workflow rearrangement needed to enable AI automation. This is important because these factors can substantially change the economic and labor market effects of automation. Existing approaches vary in which of these factors they model, and in what detail (if any) they are predicted.
-
Engagement with deployment practicalities: bottlenecks, incentives, and regulatory issues.
These can be significant drivers of real world automation progress. Measuring “in-principle-automatability” while ignoring these considerations, as some approaches do, can produce predictions that diverge vastly from real world automation. Predictions that neglect deployment can also be ill-defined and hard to falsify. The strongest signal a task can be automated is when it is successfully automated. Ideally, a prediction methodology should incorporate these details, or at least provide a clear way to model them separately.
-
Inputs have a strong theoretical and/or empirical justification for predicting automation.
Structured prediction methodologies use inputs such as ratings of task suitability for AI, task descriptions, skill ratings, patents, and AI capabilities benchmarks. Ideally, these should have the strongest possible justification for why they predict automation. For example, Eloundou et al. (2023) discusses how rapid changes in AI capabilities may challenge older ratings of task suitability for AI.
-
Predictions align with evidence on automation so far.
We are only now beginning to see empirical evidence of AI-driven automation, as opposed to automation from older technologies such as software or industrial robotics. In the labor economics literature, Acemoglu et al. (2020) offers evidence of firm-level hiring changes in response to AI exposure. There are also compelling case studies showing how AI automation can interact with occupational tasks in customer support, translation, software engineering and consulting. Predictions should be consistent with this evidence - or provide a clear reason why not.
An overview of automatability prediction methodologies
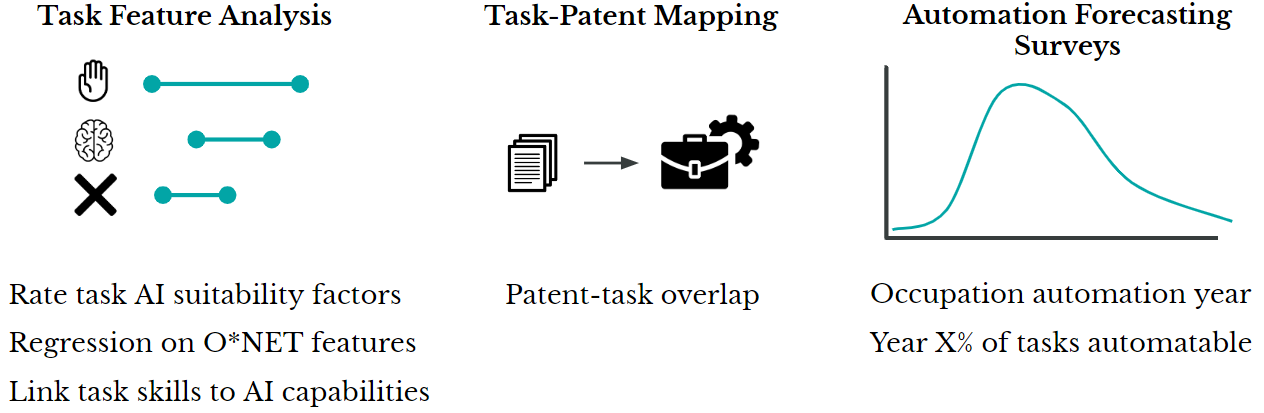
Figure 1: The academic literature on predicting task automatability falls into three categories: task feature analysis, task-patent mapping, and automation forecasting surveys. O*NET features, discussed under Task feature analysis, are from the O*NET database of occupational information, characterizing tasks in terms of required skills, abilities, and other details.
There are broadly three approaches for predicting task automatability. First, task feature analysis to measure tasks’ susceptibility to automation, typically informed by AI researchers’ opinions on the capabilities of present and near future AI. Second, as an alternative, task-patent mapping based on correlating keywords between task descriptions and recent innovations such as patents. Finally, there are automation forecasting surveys for when tasks or activities can be (or will be) automated. Only surveys include explicit forecasts of when automation will happen, while the other approaches provide relative measurements of automatability.
In this section, we review the literature on automatability prediction, focusing on methodologies - how the different methods operate. We briefly discuss broad differences in Overview of predictions, and provide example outputs in Appendix: example outputs from different methods, but they are not the focus of this review.
Methodology | Overview | Inputs | Reproducibility | |
Task feature analysis | Autor et al. (2003)
Pre-AI automation, adapted to O*NET variables by Acemoglu and Autor (2011). |
Classify tasks as routine / non-routine, cognitive / physical. | Transformed variables from Dictionary of Occupational Titles (predecessor of O*NET). | Categorisation published in paper. |
Frey and Osborne (2013) | Label occupation automatability with current AI. Extrapolate to all occupations by regression on 9 O*NET “bottlenecks” for AI. | AI researchers surveyed about occupation full automatability. | High-level categorisation published in paper. | |
Arntz et al. (2016) | Regress individual task automatability from inter-job variation in Frey and Osborne (2013). | Worker survey on tasks, Frey and Osborne occupation-level automatability scores. | Occupation-level ratings reported in paper. | |
Manyika et al. (2017), followed up later by Chui et al. (2023) | Rate tasks / activities / skills’ automatability. | Proprietary automatability ratings. | Proprietary data, not reported at occupation level. | |
Duckworth et al. (2019) | Rate task automatability. Regression on 120 O*NET features (skills, knowledge, abilities) to extrapolate. | Online survey of 156 “experts in machine learning, robotics and
intelligent systems”. |
Dataset and code available. | |
Felten et al. (2021), building on Felten et al. (2018) | Relate AI benchmarks to O*NET abilities. | EFF AI Progress Measurement benchmarks, crowdsourced ratings of benchmark-ability linkage. | Dataset available including benchmark-ability linkage, results at level of occupation, industries and US counties. | |
Brynjolfsson et al. (2018) | Rate activities on a 23-item rubric of suitability for ML (SML). | Crowdsourced survey. | Dataset and code available. | |
Eloundou et al. (2023) | Rate tasks according to whether they can be sped up >2x by ChatGPT or similar systems. | Ratings from GPT-experienced annotation workers, and ratings from GPT-4. | Rubric included in appendix, data not released. | |
Task-patent mapping | Webb (2019) | Identify task descriptions’ overlap with patents. Aggregate to occupations. | Patents (separate sets for AI, software, industrial robotics). | Dataset and code available. |
Automation forecasting surveys | Gruetzemacher et al. (2020) | Forecast fractions of tasks automatable. | 203 attendees at three ML conferences in 2018. No definitions for automatable or percentages. | Summary data available in paper. |
Stein-Perlman et al. (2022) | Forecast automatable-in-principle year for all tasks of a job. | 738 authors from NeurIPS or ICML papers in 2022. Focus on in-principle-automatability. | Dataset available. |
Table 1: Overview of the main prediction methodologies, including a brief description of how they work, the inputs they use for rating task automatability, and reproducibility.
Task feature analysis
Background
Attempts to measure task automatability in the economics literature arguably began with Autor et al. (2003). They broke down occupational tasks into routine and non-routine labor, routine meaning “a limited and well-defined set of cognitive and manual activities, those that can be accomplished by following explicit rules [as opposed to] problem-solving and complex communication activities”. They scored task routineness using pre-existing ratings in areas such as “Direction, Control and Planning”. Earlier work had examined the diffusion of computers and industrial robotics and their effects, and work from other disciplines had identified routineness as a key predictor of automation. Autor et al. (2003) applied this categorisation to tasks in a production function, developing a model in which automation replaced routine labor and complemented non-routine labor. This model’s predictions matched empirical evidence on labor demand.
Developments in AI later began to challenge the Autor et al. (2003) model of automation. Increasingly, tasks that had been rated as non-routine became more amenable to automation (Susskind, 2019). The first authors to create general metrics for AI automatability across the economy were Frey and Osborne (2013). They asked AI researchers whether 70 occupations could be fully automated in the near future, focusing on the technical capability to automate them rather than whether they would be automated. They then used these survey results to fit a regression to estimate automatability from nine manually identified O*NET skill variables related to AI bottlenecks. These were broadly in the areas of Perception and Manipulation, Creative Intelligence, and Social Intelligence. Their work contained a much-quoted finding that ~50% of total US employment was at high risk of automation (>70% on their metric). Several authors subsequently performed similar analyses for different countries’ labor markets, but without significant changes in methodology.
Task-focused analyses
Several researchers responded to Frey and Osborne (2013) by implementing task-focused analyses - arguing that automation usually affects tasks within occupations, rather than entire occupations (Arntz et al., 2016; Manyika et al., 2017; Brandes and Wattenhofer, 2016; Nedelkoska et al., 2018; Duckworth et al., 2019). Theseworks typically found that considering individual tasks significantly reduced the “high risk” share of employment, for example to 9% across OECD countries in Arntz et al. (2016). These methods continued to focus on researchers’ guesses of what could, in theory, be fully automated at the time of their surveys.
Of particular note, due to its transparency and replicability, Duckworth et al. (2019) expanded on Frey and Osborne (2013) by producing their own task-based formulation and open source dataset. They focused on tasks that were technically automatable at the time of investigation, and a more fine-grained level of detail, i.e. O*NET tasks. Their approach aggregated tasks into work activities, weighted by task importance and occupation requirements (skills, knowledge, and abilities). They then regressed automatability from occupation requirements for all O*NET work activities. This gave results more similar to Arntz et al. (2016) and Manyika et al. (2017) than Frey and Osborne (2013), i.e. few occupations entirely exposed to automation.
Brynjolfsson and Mitchell (2017) created a structured rubric to measure task automatability in terms of Suitability for Machine Learning (SML). This was potentially an improvement on preceding work by making assessments that are more legible and future-facing than regressing expert predictions via O*NET variables. Brynjolfsson et al. (2018) assessed O*NET work activities in this rubric using crowdsourcing, finding broadly similar proportions of exposed work to Arntz et al. (2016) and Duckworth et al. (2019).
Felten et al. (2018) developed an alternative metric for automatability based on AI benchmarks. They attempted to link AI benchmarks to abilities required by occupations, as categorized by O*NET. They focused on analysis at the occupation level, although O*NET also couples abilities to work activities, so the same dataset could be disaggregated. At first this was done in a backwards-looking way, with abilities linked to benchmarks by the authors with advice from computer science PhD students. Subsequently, this was expanded to include a crowdsourced survey for a forward-looking linkage in Felten et al. (2021), and inspired several similar approaches in subsequent work: Lassebie and Quintini (2022); Josten and Lordan (2020); Tolan et al. (2021).
Response to generative AI and LLMs
A handful of publications have been inspired by recent advances in generative AI, revisiting previous analyses and accounting for rapid progress in this area. Felten et al. (2023) revisited their automatability analyses, focusing on the tasks and occupations most exposed to language modeling and image generation. Chui et al. (2023) released a report focused on generative AI, broadly following the methodology of Manyika et al. (2017) - with estimates of roll-out updated to be significantly faster, based on recent advances.
Eloundou et al. (2023) devised a prediction methodology built specifically around large language models (LLMs). They categorized tasks for automation potential from GPT-like systems. This had three strengths: (i) assessing automation potential based on breakthrough AI capabilities demonstrated by LLMs such as GPT-4; (ii) defining a rubric focused on 2x task speed-up rather than full automation; (iii) rating O*NET tasks at the most fine-grained level, providing the most thorough automatability measures to date. Strikingly, the study used a combination of GPT-experienced human raters and GPT-4 itself to rate tasks - showing that there was high agreement between them.
Task-patent mapping
An alternative, data-driven approach to measuring automatability is to relate AI patents to occupations whose activities they may automate. This approach was pioneered by Webb (2019), who extracted verb-noun pairs from patents and related them to fine-grained O*NET tasks and occupation descriptions. This allowed him to measure technological exposure to AI – or to older technologies such as industrial robotics and software – depending on the selection of patents. Meindl et al. (2021) did similar work, and expanded the patent selection to cover a broad selection of “Fourth Industrial Revolution” topics. Similarly, Zarifhonarvar (2023) has recently explored a similar text mining approach for measuring occupational exposure to generative language models. Webb (2019)’s data release allowed for a broad comparison against metrics such as Felten et al. (2018) or Brynjolfsson et al. (2018) - discussed further in Empirical evidence and comparison.
Automation forecasting surveys
The main literature source giving explicit predictions measured in years is Stein-Perlman et al. (2022). This survey of AI researchers asked when all tasks in four example occupations (truck driver, surgeon, retail salesperson, AI researcher) would be technically automatable by AI. They also asked for examples of occupations that would be among the most difficult to automate. Although inter-respondent variation was large, median forecasts were that automatability may be achieved in 10 years for all tasks performed by truck drivers or retail salespersons. However, median forecasts also suggested that the final occupations to become automatable will not be automatable until much later (80 years). There were many different suggestions for the last occupation to become automatable. These tended to be occupations that (i) benefit from interpersonal physical interaction (nurse, therapist); (ii) involve high social status and charisma (politician, CEO); or (iii) require a lot of originality, creative thought and analytic rigor (philosopher, AI researcher).
Another source of forecasting surveys is Gruetzemacher et al. (2020), which elicited predictions on the overall fraction of automatable tasks versus year, surveying attendees at three machine learning conferences in 2018. While not focusing on individual tasks, this provides some insight into researchers’ overall beliefs. The surveys showed that researchers believed a large fraction of tasks are already automatable (~20%) and that this would increase dramatically in the next decade (~60%). Predictions had high variance, however, and predictions of the tasks automatable in 10 years ranged from 10% to 100%.
Overview of predictions
Comparison of existing methodologies’ predictions is fairly sparse in the literature, with a few exceptions such as Acemoglu et al. (2020). In this section we compare predictions, at the level of broad occupational categories and individual occupations. We show there is remarkably little agreement between the different predictions, although a common feature of all AI-focused methodologies is that they rate Managers and Professionals as more exposed to automation than traditional automatability measures.
Figure 2 compares automatability measures for twelve broad occupation categories, which have often been used for studying automation. Although aggregating to broad occupation categories elides over task details, these results provide an accessible snapshot of each methodology. Figure 2 only covers task-level methodologies with data releases. We provide comparison of example results from other methodologies in Appendix: example outputs from different methods. The routineness measure of Acemoglu and Autor (2011) is also included in Figure 2 to allow comparison with pre-AI automation.
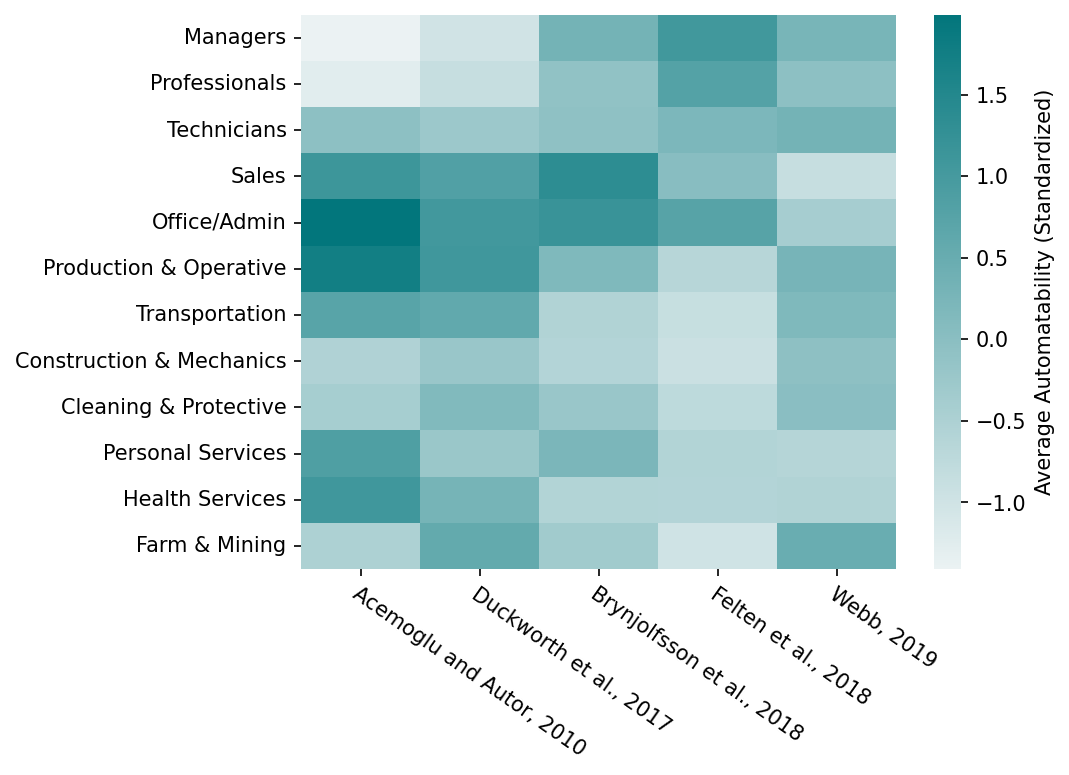
Figure 2: Average automatability measures for twelve broad occupation categories used in Acemoglu et al. (2020) and other sources. Higher scores indicate higher automatability. Occupations are ordered from highest to lowest median wage - broadly following traditional ratings of skill level. Measures have been standardized to employment-weighted z-scores at occupation level before aggregation to broad occupation categories. Data is taken from respective publications or the comparison in Acemoglu et al. (2020). In this figure the Acemoglu and Autor (2011) measure uses the sum of cognitive and physical net routineness scores, for simplicity.
Notably, most AI methodologies predict that higher-wage occupations such as Managers & Professionals have middling-to-high automatability. Duckworth et al. (2019) does not follow this pattern, and is generally closer to older pre-AI predictions - consistent with its broader scope and survey focused on currently-automatable tasks. Unfortunately, Eloundou et al. (2023) does not have a data release, preventing its inclusion in Figure 2. However, one should expect higher ratings for occupations involving more cognitive tasks, fewer manual tasks, and higher educational requirements - i.e. following the same pattern.
In the AI-focused methodologies, there is relatively little agreement between measures. Brynjolfsson et al. (2018) and Felten et al. (2018) are somewhat similar, showing a broad pattern where the occupations traditionally seen as high-skill are more automatable. However, the individual occupational categories do not agree closely. Webb (2019), conversely, predicts middling automatability across most occupations, with notable exceptions in Sales and Office/Admin (less automatable), and Farm & Mining (more automatable). This intuitively matches the method’s focus on patents before generative AI, typically involving “systematic relationships between inputs and decisions, such as sorting agricultural products” (Webb, 2019).
The low agreement between different automatability measures is explored in more detail in Figure 3. Figure 3 shows pairwise comparison of automatability measures, plotting their respective ratings for individual O*NET occupations. Duckworth et al. (2019) shows fairly close agreement with Acemoglu and Autor (2011), further supporting the interpretation that it is dominated by traditional pre-AI ideas of automatability. However, AI-focused measures have little correlation with each other: the strongest agreement is between Felten et al. (2018) and Brynjolfsson et al. (2018), where one measure can explain only 8% of the other’s variance. Other AI measures are wholly uncorrelated. This emphasizes their significantly different predictions, although without validation data it is unclear whether this implies some predictions are more accurate, or that different methods “capture different components of AI exposure” (Acemoglu et al., 2020).
Empirical evidence and comparison
It is difficult to test the various prediction methods because AI innovations are relatively new, and only recently became usable in many real world applications. We identify two strands of empirical evidence on AI automation: empirical studies of economic effects from automation, and case studies on AI in specific applications. We review key results from each, with the review of automation effects focusing on research that compares automatability measures with empirical evidence. Finally, in Comparison of prediction methodologies, we compare how the different methodologies line up with the desiderata of What do we want from automation predictions?. We show that many important challenges remain unresolved, but the field is showing promising signs of progress.
Studies of economic effects from automation are heavily confounded by other automating technologies, such as software or industrial robotics. This section does not discuss the rich literature investigating earlier automation (see Autor (2013) and others). We focus on empirical evidence around AI-driven automation: investigations that explicitly examine AI automatability measures, or case studies on AI technologies made possible by deep learning. Focusing on AI-driven automation, there is early evidence of firm-level hiring changes that are correlated with several automatability measures: Brynjolfsson et al. (2018), Felten et al. (2018), and Webb (2019). In contrast, case studies on AI have narrower scope, but offer fairly strong and detailed evidence for the automatability of tasks in customer support, translation, software engineering, and management consulting, albeit with greater external-validity concerns.
Studies of economic effects from AI automation
Several authors have analyzed occupation-level O*NET Degree of Automation data, which is collected from surveying workers and domain experts: Scholl and Hanson (2020), Otoiu (2022); Carlson (2023). Automatability metrics, such as Frey and Osborne (2013) and Brynjolfsson et al. (2018), were associated with increases in Degree of Automation. However, these metrics were no longer informative when combined with job characteristics such as “Pace Determined By Speed of Equipment”, which have straightforward interpretations in terms of traditional automation via e.g. industrial robotics. Concerningly, Scholl and Hanson (2020) found automation was flat or even decreasing over time after controlling for other factors. They suggested this may be due to shifting standards in what a worker considers automated.
Other work has investigated changes in hiring or wages and their association with AI automatability metrics (Acemoglu et al., 2020; Georgieff and Milanez, 2021; Felten et al., 2019) or more general technological automatability metrics (Meindl et al., 2021; Kogan, 2020). However, as with Degree of Automation, this is liable to significant confounding from automation via other technologies. This is consistent with the findings: broader automatability metrics (Arntz et al., 2016; Meindl et al., 2021; Kogan, 2020) are correlated with lower hiring in the economy at large, whereas more AI-focused metrics such as Felten et al. (2019) are not. Consequently, these works may be less useful for validating AI automatability measures.
Focusing more narrowly on AI automation, Acemoglu et al. (2020) examined job advertisement data, finding that companies exposed to automation increased hiring for AI roles and decreased hiring for automation-exposed roles. They demonstrated this finding held under three different automatability ratings: Webb (2019), Felten et al. (2018), and Brynjolfsson et al. (2018), although less robustly for Brynjolfsson et al. (2018). These results held after attempting to control for potential confounders, such as non-AI automation via non-AI software-focused patents using Webb (2019).
Case studies on AI in specific applications
There are a growing number of in-depth case studies examining AI automation. These could provide convincing evidence of AI improving productivity within representative tasks in the real world. Overall, these case studies suggest there are already measurable productivity gains from AI in certain occupations. They also offer evidence for all three of the automation types: deskilling of tasks, improvement of productivity through partial automation, and labor-replacing full automation.
Customer support and conversational assistants: Brynjolfsson et al. (2023) found that a GPT-based conversational assistant AI was able to improve customer support agents’ productivity by 14%. This appears to have been achieved by raising the standard of weaker, inexperienced agents’ work to match the best pre-existing workers - the latter group saw no significant benefit. This is a clear example of deskilling and consequent productivity improvement for most workers.
Software engineering: Peng et al. (2022) claimed that programmers using the Copilot programming assistant were able to implement a HTTP server in JavaScript 55% faster than a control group. This result appears to have come mostly from performance improvements for slower participants – consistent with deskilling. Notably, a previous study found that Copilot’s largest effects may be user satisfaction and perceived productivity rather than straightforward task speed Ziegler et al. (2022). A related case study, Tabachynk and Nikolov (2022), examined a similar ML-based code completion tool developed by Google for internal use. They found a 6% reduction in iteration time, lending further support to the idea that Copilot-like tools can improve programmer productivity. Moreover, these results suggest productivity gains from these tools may apply beyond lower-skilled software engineers.
Translation and question answering: Yilmaz et al. (2023) used Google’s introduction of AI translation in 2016-2017 as a natural experiment investigating its effect on online translators. They found that language pairs where the technology was introduced saw a fast drop in labor demand, with transaction value decreasing by about 30% relative to other language pairs. This difference was particularly focused on more routine translation tasks, whereas less routine “transcreation” tasks saw no decline in the same period. They also applied a similar methodology to ChatGPT and StackOverflow questions, finding a ~10% decrease in programming questions after ChatGPT’s release. The translation findings are suggestive of labor-replacing automation: the fall in demand for easier translation tasks was presumably due to the translation system being used to perform them. It is unknown whether end users did additional labor to proofread or correct these translations.
Management consulting: Dell’Acqua et al. (2023) performed an in-depth study on the use of GPT-4 on two categories of management consulting tasks: to “conceptualize and develop new products”, and “business problem-solving tasks using quantitative data, customer and company interviews”. Notably, the latter task was intentionally selected such that “AI couldn’t easily complete [the task] through simple copying and pasting of our instructions as a prompt”. For the “conceptualize and develop new products” tasks, AI assistance was highly beneficial. Completion speed increased by 25% and quality scores increased by over 40%. These effects were again larger for lower-skilled employees, consistent with a deskilling effect; however, higher-skilled employees also showed significant improvement. Strikingly, for the “business problem-solving” tasks, AI assistance reduced correct answer rates by 19%, leading the authors to categorize them as “outside the frontier” of GPT-4’s capabilities.
Comparison of prediction methodologies
Methodology | Interpretability | Deployment | Empirical or theoretical justification of inputs | Evidence so far | |||||
Partial automation | Timescale (future-facing) | AI capabilities up to date | O*NET features linkage (if any) | Fine grained task data | AI case studies | Hiring changes (AI-specific) | |||
Task feature analysis | Frey and Osborne (2013) | ✗
Excluded. |
✗
Present day automatable. |
✗ | ✗ | ✗
Occupation-level regression. |
✗ | ✗ | ✗ |
Felten et al. (2021) | ✓
Not explicitly defined. |
✓
Exposure to AI benchmarks. |
✗ | ?
2023 update for LLMs. |
?
Abilities-benchmarks via binary survey. |
✗ | ✓
All rated highly exposed. |
✓ | |
Duckworth et al. (2019) | ✓
Scale includes partial and full. |
✗
Present day automatable. |
✗ | ✗ | ?
Work Activity level regression. |
✗
Queried at task-level, analyses at activity-level. |
?
Only customer service is highly exposed. However, this metric also covers non-AI automatability, |
?
Not analyzed. |
|
Brynjolfsson et al. (2018) | ✓
Not explicitly defined. |
✓
Suitability for ML. |
?
Partial inclusion in rubric. |
✗ | - | ✗ | ✗
Generally rated less exposed. |
✓
Less robust than Felten et al. or Webb. |
|
Eloundou et al. (2023) | ✓✓
Defined: >2x task speed-up. |
?
Near future GPT-like systems. |
✓
Inclusion in rubric. |
✓ | - | ✓ | ✓
All rated highly exposed. |
?
Not analyzed. |
|
Task-patent mapping | Webb (2019) | ✓
Patents implicitly include this. |
✓
Partial, implicit in patents. |
✓
Implicitly linked to patents. |
✗
Could update with new patents. |
- | ✓ | ✓
Computer programmers, management analysts high; customer service middling. |
✓ |
Automation forecasting surveys | Gruetzemacher et al. (2020) | ✗
Not explicitly defined. |
✓✓
Fraction automated by year. |
✗
Ambiguous. |
? | - | ✗ | - | - |
Stein-Perlman et al. (2022) | ✗
Excluded. |
✓✓
Predict years for occupations. |
✗ | ✓ | - | ✗ | - | - |
Table 2: Comparison of prediction methodologies against the criteria of What do we want from automation predictions? We exclude Autor et al. (2003) as it is not AI-focused; methodologies such as Arntz et al. (2016), which are derived from Frey and Osborne (2013); and methods such as Manyika et al. (2017) and Chui et al. (2023), which provide fewer details on data collection.
We compare how the different methods line up against What do we want from automation predictions? in Table 2. No approach can yet fulfill all these ambitious desiderata, but there are signs of progress over time, with methodologies increasingly accounting for partial automation, deployment details, and fine grained task specifications. Below, we discuss salient differences between approaches for each desired property.
Interpretability: Most methodologies account for partial automation in some way, for example by using the same scale of automatability to measure from partial to full automation. Eloundou et al. (2023) provides the clearest definition here, in terms of tasks being sped up by 2x or greater. Most methodologies are forward-looking, with the exception of “nowcasting” approaches such as Frey and Osborne (2013) and Duckworth et al. (2019), but only forecasting surveys provide explicit timelines.
Deployment: Many methods ignore details of deployment, incentives, regulatory issues and similar. Brynjolfsson et al. (2018) partially accounts for these within its rubric, via questions such as whether it is “important that [task] outputs are perceived to come from a human”. More explicitly, the rubric of Eloundou et al. (2023) accounts for these details; and the measure of Webb (2019) implicitly accounts for them to some extent by using patents.
Inputs: Most approaches have been challenged by rapid progress in AI capabilities. Eloundou et al. (2023), due to its recency and focus on AI systems similar to an existing example (GPT), is a clear exception. Arguably, Felten et al. (2023) demonstrates that the methodology of Felten et al. (2021) could cope with changing AI capabilities if updated to focus on progress in language AI benchmarks. However, this is challenged by uncertainties about O*NET feature linkage: it is unclear how well binary surveys linking AI benchmarks to O*NET abilities can model the effect of AI progress. Several methodologies avoided using O*NET features, slightly bypassing this concern. Finally, Eloundou et al. (2023) and Webb (2019) are the only methods using fine grained tasks - O*NET tasks, as opposed to work activities.
Evidence so far: Eloundou et al. (2023) matches closely with all case studies. Arguably this is unsurprising, given the method’s recency and the case studies’ focus on AI applications amenable to GPT-like systems. More surprisingly, Felten et al. (2021) and Webb (2019) also align with case studies, despite being developed before recent AI advances in language modeling. Felten et al. (2021) and Webb (2019) also have the most robust correlation with AI-specific hiring changes, among analyzed methods.
Discussion
Forecasting automatability remains an open problem in many ways: in formulation, in methodological development, and in validation. Based on the comparison of existing prediction approaches in Comparison of prediction methodologies, we highlight three crucial but unresolved methodological questions: interpretable predictions, realistic deployment, and linking automation to AI capabilities.
Considering prediction interpretability, it is often unclear how automatability ratings correspond to automation type, extent and timescale. Eloundou et al. (2023) improved definitions around automation type and extent by specifying that tasks should be sped up at least 2x. Potentially, this could be combined with a rubric similar to Duckworth et al. (2019), explicitly categorizing whether tasks would be fully or partially automated - or quantifying the extent of speed-up.
The details of deployment - incentives, workflow rearrangement, societal preferences - can be significant drivers of real world automation. In earlier work, Manyika et al. (2017) developed proprietary models of technology roll-out based on historical precedents. Recent work has partially addressed deployment by including some details within task ratings, for example in Brynjolfsson et al. (2018) and Eloundou et al. (2023). Webb (2019) pursued a more data-driven approach using patents, which may be more closely linked to technology roll-out. There is significant scope for further work, building on these or otherwise.
Linking automation to AI capabilities underpins all of the approaches categorized as metrics in this review. Often, these focus on shorter term predictions, based on the capabilities of present day AI systems. Felten et al. (2021) and Tolan et al. (2021) have proposed forward-looking predictions by linking automation to AI capabilities in a way compatible with future progress. These approaches rate exposure to AI benchmarks, but there are significant open questions about how this exposure might predict automation. Recent advances in AI benchmarks and evaluations offer a promising starting point, which might eventually allow benchmark performance to be more directly related to automatability.
Having covered these open methodological questions, we add that it may be valuable for future work to provide an extended comparison of existing approaches’ predictions. Such a comparison might substantially expand on Figure 2 and Figure 3, comparing the factors that drive automatability in each method and examining how task automatability interacts with occupation-level and task-level characteristics.
In conclusion, there is a growing literature on forecasting task automation by AI, and recent advances in AI have simultaneously changed researchers’ predictions while emphasizing the importance of this question. This review has investigated the main forecasting approaches in the economics literature, separating the approaches into task feature analysis, task-patent mapping, and automation forecasting surveys. This review has also examined empirical evidence supporting these predictions: hiring changes in response to AI automation, and case studies examining AI assistance in several domains. There is some empirical evidence that prediction methodologies are consistent with AI automation so far, although many challenges remain unsolved, particularly around automation details and realistic deployment, and predicting beyond current AI systems. We are excited to see how future work will build on recent progress, and hope that this review may help researchers engage with this important problem.
Acknowledgments
We thank Misha Yagudin, Amelia Michael, Anson Ho, Jaime Sevilla and Josh You for their help with this work.
Appendix: example outputs from different methods
Table 3 lists examples of tasks or occupations with high or low automatability ratings across many different methods. These examples are more fine grained than the comparison at the level of broad occupations in Figure 2. For automation forecasting surveys, Table 3 instead reports a high-level summary of their overall predictions, as neither survey examines a large sample of occupations.
Methodology | More automatable | Less automatable | |
Task feature analysis | Autor et al. (2003)
Pre-AI automation, adapted to O*NET variables by Acemoglu and Autor (2011). |
Office clerks
Customer service clerks Precision, handicraft, craft printing and related trade workers |
Managers of small enterprises
Drivers and mobile plant operators Life science and health professionals |
Frey and Osborne (2013) | Telemarketers
Title Examiners, Abstractors, and Searchers Hand Sewers Mathematical Technician Insurance Underwriters |
Recreational therapists
First-line supervisors of mechanics, installers, and repairers Emergency management directors Mental health and substance abuse social workers |
|
Arntz et al. (2016) | Similar to Frey and Osborne | ||
Manyika et al. (2017), followed up later by Chui et al. (2023) | Sewing machine operators
Graders and sorters of agricultural products Travel agents |
Psychiatrists
Legislators Chief executives Statisticians |
|
Duckworth et al. (2019) | Examine physical characteristics of gemstones or precious metals.
Adjust fabrics or other materials during garment production. Evaluate quality of (wood) logs. Record shipping information. |
Teach humanities courses at the college level.
Teach online courses. Conduct scientific research of organizational behavior or processes. Develop promotional strategies for religious organizations. |
|
Felten et al. (2021)
Related work: Felten et al. (2023), Felten et al. (2018) |
Genetic counselors
Financial examiners Actuaries Purchasing agents, except wholesale, retail, and farm products |
Dancers
Fitness Trainers and Aerobics Instructors Helpers – painters etc Masons |
|
Brynjolfsson et al. (2018) | Concierges
Mechanical drafters Morticians Credit authorizers Brokerage clerks |
Massage therapists
Animal scientists Archaeologists Public address system and other announcers Plasterers and masons |
|
Eloundou et al. (2023) | Mathematicians
Blockchain engineers Accountants and auditors News Analysts, Reporters, and Journalists |
Physical skills were unexposed by definition, e.g. Agricultural Equipment Operators, Athletes, etc.
Less obviously, science and critical thinking skills were negatively correlated with exposure. Example low exposure non-physical occupations were not listed. |
|
Task-patent mapping | Webb (2019) | Clinical laboratory technicians
Chemical engineers Optometrists Power plant operators Dispatchers |
Animal caretakers, except farm
Food preparation workers Mail carriers for postal service Subject instructors, college Art/entertainment performers |
Automation forecasting surveys | Gruetzemacher et al. (2020) | Median forecasts:
|
|
Stein-Perlman et al. (2022) | Median forecasts of 50% cumulative probability of automatability:
|
Table 3: Example automatability ratings, by method. These vary between task-level, occupation-level, or explicit forecasts of timelines for specific occupations or task fractions.
Bibliography
Acemoglu, Daron, and David Autor. “Skills, tasks and technologies: Implications for employment and earnings.” Handbook of Labor Economics. Vol. 4. Elsevier, 2011. 1043-1171.
Acemoglu, Daron, and Pascual Restrepo. “Automation and new tasks: How technology displaces and reinstates labor.” Journal of Economic Perspectives 33.2 (2019): 3-30.
Acemoglu, Daron, et al. “AI and jobs: Evidence from online vacancies.” No. w28257. National Bureau of Economic Research, 2020.
Arntz, Melanie, Terry Gregory, and Ulrich Zierahn. “The risk of automation for jobs in OECD countries: A comparative analysis.” (2016).
Autor, David H., Frank Levy, and Richard J. Murnane. “The skill content of recent technological change: An empirical exploration.” The Quarterly Journal of Economics 118.4 (2003): 1279-1333.
Autor, David H. “The “task approach” to labor markets: an overview.” Journal for Labour Market Research 46.3 (2013): 185-199.
Brandes, Philipp, and Roger Wattenhofer. “Opening the Frey/Osborne black box: Which tasks of a job are susceptible to computerization?” arXiv preprint arXiv:1604.08823 (2016).
Brynjolfsson, Erik, and Tom Mitchell. “What can machine learning do? Workforce implications.” Science 358.6370 (2017): 1530-1534.
Brynjolfsson, Erik, Tom Mitchell, and Daniel Rock. “What can machines learn, and what does it mean for occupations and the economy?.” AEA Papers and Proceedings. Vol. 108. 2018.
Brynjolfsson, Erik, Danielle Li, and Lindsey R. Raymond. “Generative AI at work.” No. w31161. National Bureau of Economic Research, 2023.
Carlson, Eric. “Crafting the Future of Work: A Policy Framework for the Automation Age.” 2023.
Chui, Michael et al. “The Economic Potential of Generative AI.” McKinsey Global Institute, 2023.
Dell’Acqua, Fabrizio, Edward McFowland, Ethan R. Mollick, Hila Lifshitz-Assaf, Katherine Kellogg, Saran Rajendran, Lisa Krayer, François Candelon, and Karim R. Lakhani. “Navigating the Jagged Technological Frontier: Field Experimental Evidence of the Effects of AI on Knowledge Worker Productivity and Quality.” Harvard Business School Technology & Operations Mgt. Unit Working Paper 24-013 (2023).
Duckworth, Paul, Logan Graham, and Michael Osborne. “Inferring work task Automatability from AI expert evidence.” Proceedings of the 2019 AAAI/ACM Conference on AI, Ethics, and Society. 2019.
Eloundou, Tyna, et al. “GPTs are GPTs: An early look at the labor market impact potential of large language models.” arXiv preprint arXiv:2303.10130 (2023).
Erdil, Ege, and Tamay Besiroglu. “Explosive growth from AI automation: A review of the arguments.” arXiv preprint arXiv:2309.11690 (2023).
Felten, Edward W., Manav Raj, and Robert Seamans. “A method to link advances in artificial intelligence to occupational abilities.” AEA Papers and Proceedings. Vol. 108. 2018.
Felten, Edward, Manav Raj, and Robert Channing Seamans. “The effect of Artificial Intelligence on human labor: An ability-based approach.” Academy of Management Proceedings. Vol. 2019. No. 1. Briarcliff Manor, NY 10510: Academy of Management, 2019.
Felten, Ed, Manav Raj, and Robert Seamans. “How will Language Modelers like ChatGPT Affect Occupations and Industries?” arXiv preprint arXiv:2303.01157 (2023).
Felten, Edward W., Manav Raj, and Robert Seamans. “Occupational heterogeneity in exposure to generative AI.” Available at SSRN 4414065 (2023).
Felten, Edward, Manav Raj, and Robert Seamans. “Occupational, industry, and geographic exposure to artificial intelligence: A novel dataset and its potential uses.” Strategic Management Journal 42.12 (2021): 2195-2217.
Frey, Carl Benedikt, and Michael A. Osborne. “The future of employment: How susceptible are jobs to computerisation?” Technological Forecasting and Social Change 114 (2017): 254-280.
Georgieff, Alexandre, and Anna Milanez. “What happened to jobs at high risk of automation?” (2021).
Gruetzemacher, Ross, David Paradice, and Kang Bok Lee. “Forecasting extreme labor displacement: A survey of AI practitioners.” Technological Forecasting and Social Change 161 (2020): 120323.
Josten, Cecily, and Grace Lordan. “Robots at work: Automatable and non-automatable jobs.” Springer International Publishing, 2020.
Kogan, Leonid. “Technological change and occupations over the long run.” SSRN, 2020.
Lassébie, Julie, and Glenda Quintini. “What skills and abilities can automation technologies replicate and what does it mean for workers?: New evidence.” (2022).
Manyika, James, et al. “A future that works: AI, automation, employment, and productivity.” McKinsey Global Institute Research, Tech. Rep 60 (2017): 1-135.
Meindl, Benjamin, Morgan R. Frank, and Joana Mendonça. “Exposure of occupations to technologies of the fourth industrial revolution.” arXiv preprint arXiv:2110.13317 (2021).
Nedelkoska, Ljubica, Dario Diodato, and Frank Neffke. “Is our human capital general enough to withstand the current wave of technological change?” Center for International Development, Harvard University (2018).
Otoiu, Adrian, et al. “Analysing Labour-Based Estimates of Automation and Their Implications. A Comparative Approach from an Economic Competitiveness Perspective.” Journal of Competitiveness 14.3 (2022): 133-152.
Peng, Sida, et al. “The impact of AI on developer productivity: Evidence from GitHub CoPilot.” arXiv preprint arXiv:2302.06590 (2023).
Scholl, Keller, and Robin Hanson. “Testing the automation revolution hypothesis.” Economics Letters 193 (2020): 109287.
Stein-Perlman, Zach, Benjamin Weinstein-Raun, and Katja Grace, “2022 Expert Survey on Progress in AI.” AI Impacts, 3 Aug. 2022. https://aiimpacts.org/2022-expert-survey-on-progress-in-ai/.
Susskind, Daniel. “Re-thinking the capabilities of technology in economics.” Economics Bulletin 39.1 (2019): 280-288.
Tabachnyk, Maxim, and Stoyan Nikolov. “ML-enhanced code completion improves developer productivity.” (2022).
Tolan, Songül, et al. “Measuring the occupational impact of AI: tasks, cognitive abilities and AI benchmarks.” Journal of Artificial Intelligence Research 71 (2021): 191-236.
Webb, Michael. “The impact of artificial intelligence on the labor market.” Available at SSRN 3482150 (2019).
Yilmaz, Erdem Dogukan, Ivana Naumovska, and Vikas A. Aggarwal. “AI-Driven Labor Substitution: Evidence from Google Translate and ChatGPT.” Available at SSRN (2023).
Zarifhonarvar, Ali. “Economics of ChatGPT: A labor market view on the occupational impact of artificial intelligence.” Available at SSRN 4350925 (2023).
Ziegler, Albert, Eirini Kalliamvakou, X. Alice Li, Andrew Rice, Devon Rifkin, Shawn Simister, Ganesh Sittampalam, and Edward Aftandilian. “Productivity assessment of neural code completion.” In Proceedings of the 6th ACM SIGPLAN International Symposium on Machine Programming, pp. 21-29. 2022.