We’re investigating the trajectory of AI for the benefit of society
Epoch AI is a research institute investigating key trends and questions that will shape the trajectory and governance of AI
Discover our work
We are working on reports, papers, models, and visualizations to advance evidence-based discussions about AI.
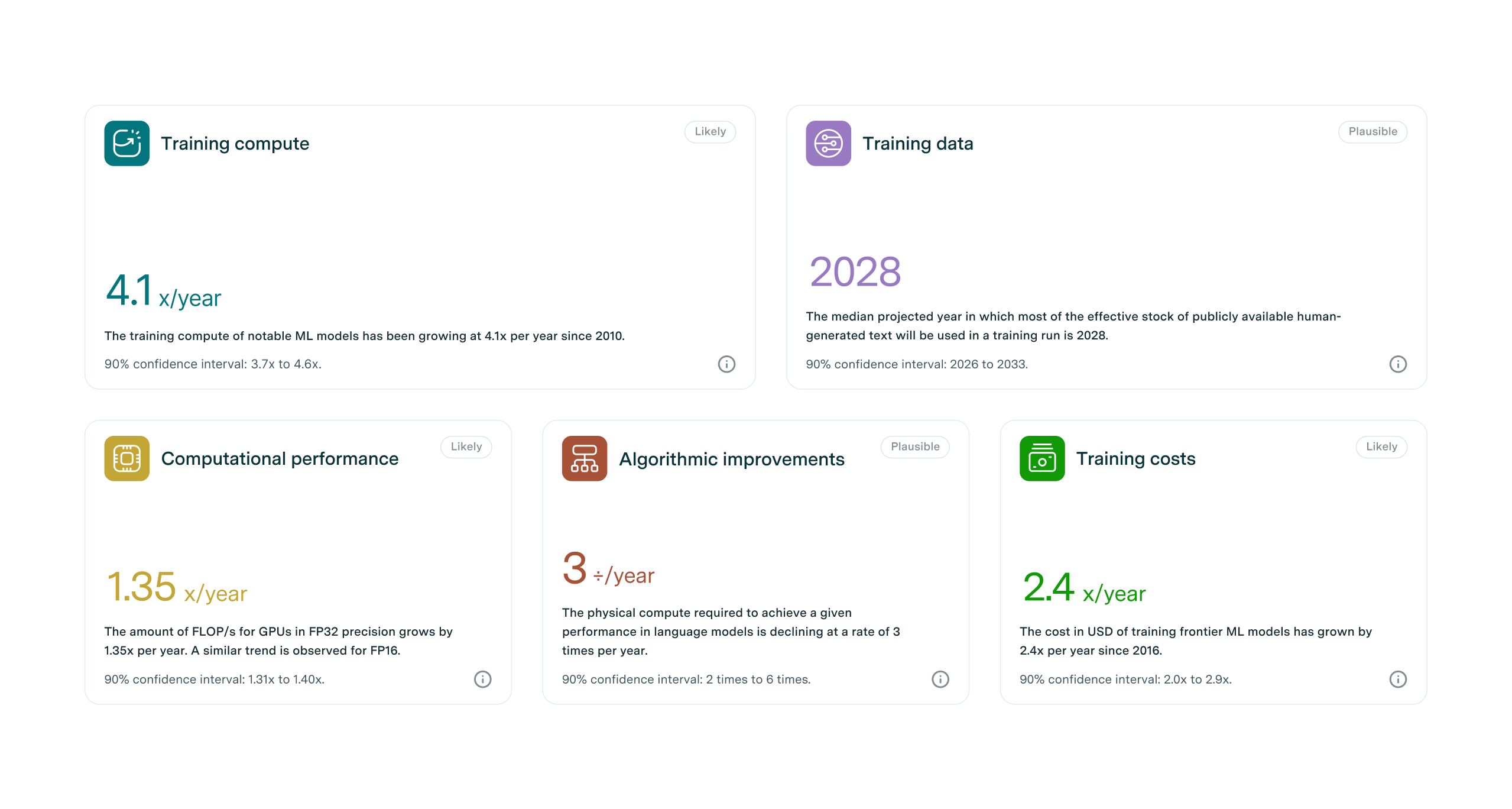
Machine Learning Trends
A collection of key data from our research on machine learning.
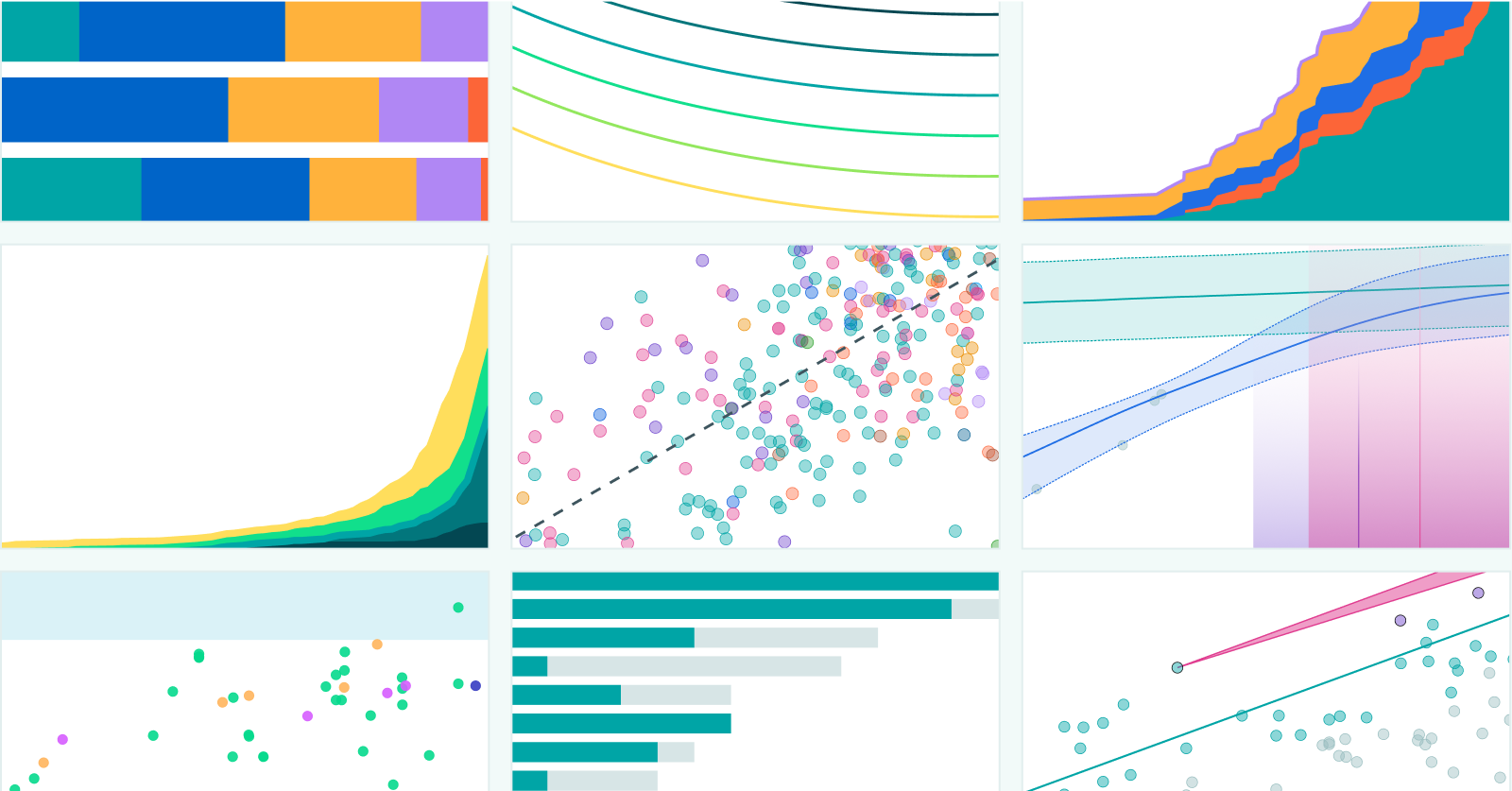
Data on the Trajectory of AI
Epoch AI collects key data on machine learning models from 1950 to the present to analyze historical and contemporary progress in AI.
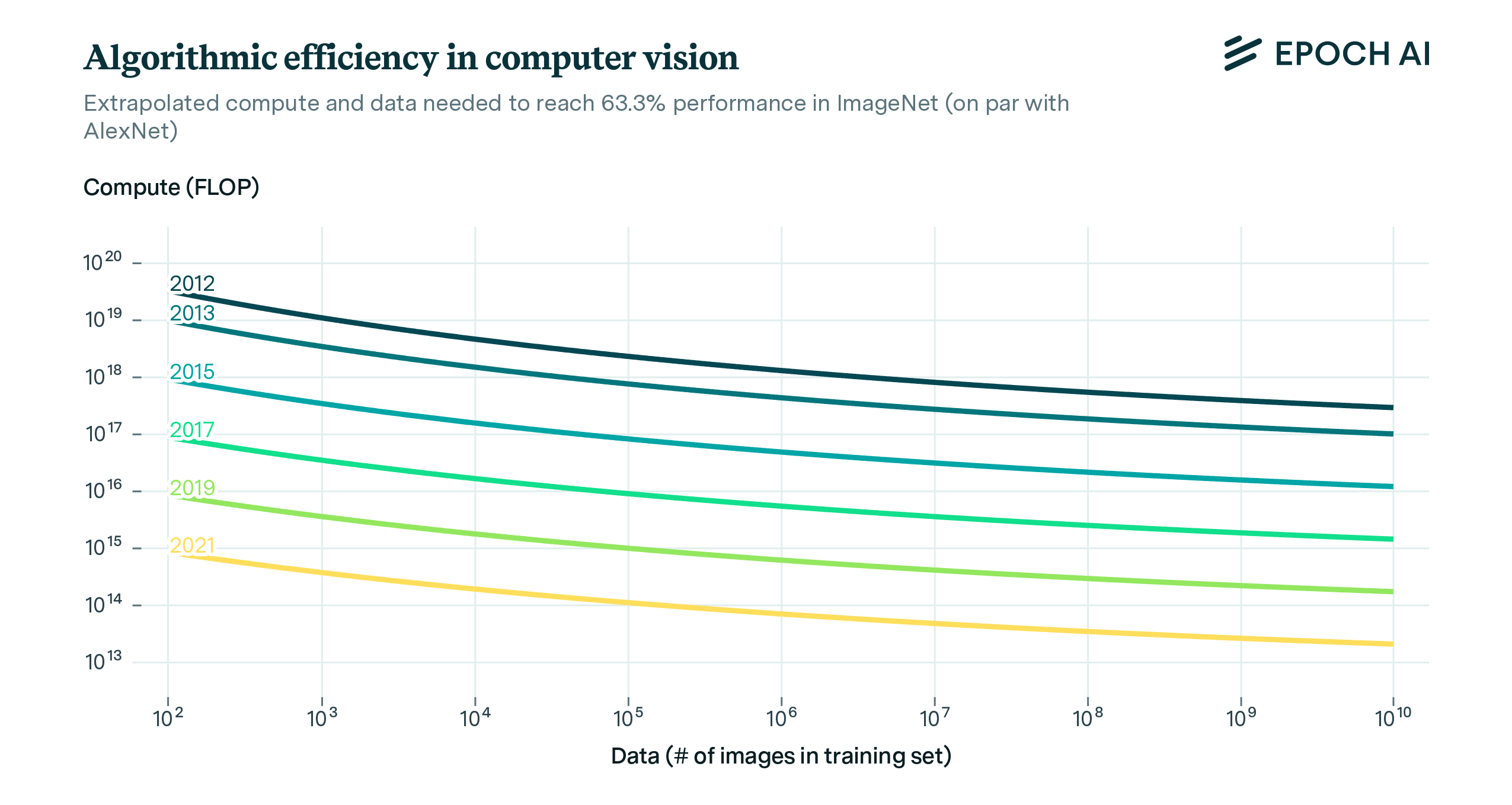
Revisiting Algorithmic Progress
Using a dataset of over a hundred computer vision models, we've quantified the rate of algorithmic improvements over the last decade.
Collaborate with us
We’re proud to work with select stakeholders on projects aligned with our mission. Our partners include:
Join us in understanding the path of AI
We're always looking for dedicated people from diverse backgrounds to collaborate on and contribute to our research.