The economic consequences of automating remote work
Published
Recent AI progress has shown great promise in automating cognitive tasks, like those in natural language processing and vision. By contrast, progress in general-purpose robotics has lagged. While we already have access to intelligent virtual assistants at our fingertips, robots capable of fully cleaning a standard suburban home still appear years away.
Given the relatively slow pace of progress in robotics, a large share of knowledge work might be automated before physical jobs are overtaken by AIs. This naturally prompts the question: what might be the economic impact if only remote work—defined as work that can be performed entirely from home using digital tools, a computer, and an internet connection—were to be automated?
To investigate this question, I conduct a three-part analysis. First, I use GPT-4o to classify the tasks involved in US occupations according to the O*NET database, finding that around 34% of job tasks can be performed remotely. This contrasts with previous findings from a study by Dingel & Neiman, which found that 37% of US occupations—not tasks—can be performed entirely remotely.
Second, I use data from the transition to remote work during the COVID-19 pandemic, along with data on the complementarity between skilled and non-skilled jobs, to argue that non-remote tasks are unlikely to become major bottlenecks in production even after remote tasks become fully automated. Put in economic terms, this suggests that the elasticity of substitution between remote and non-remote work is likely high.
After estimating both the fraction of tasks that can be performed remotely and the substitutability between remote and non-remote tasks, I calculate the theoretical economic gains from automating remote labor. Under all scenarios I consider, the magnitude of the effect is large, with the size of the economy doubling in the most conservative scenario, and growing by more than a factor of ten in the most optimistic scenario. These scenarios indicate that, even under conservative assumptions, the economic consequences of fully automating remote work would likely be huge.
What fraction of present economic work can be done remotely?
To estimate the fraction of economic work that can be done remotely, perhaps the best-known study is the highly cited work of Dingel & Neiman, “How Many Jobs Can be Done at Home?”. In this study—which was conducted in the context of the 2020 pandemic—they examine nearly 1000 occupations in the O*NET database, a large database of occupation data sponsored by the US Department of Labor for the purpose of better understanding the nature of modern work.
While O*NET contains a trove of data, it does not directly address the question of which jobs can be performed at home. Therefore, Dingel & Neiman rely on a set of proxy questions asked during surveys on job contexts to perform their own classification of remote and non-remote work. The questions include, for instance:
- Average respondent says they use email less than once per month (Q4)
- Average respondent says they deal with violent people at least once a week (Q14)
- Majority of respondents say they work outdoors every day (Q17 & Q18)
Dingel & Neiman conclude that 37 percent of jobs in the United States—which together account for roughly 46 percent of all US wages—can be performed entirely from home. By cross-referencing this data with the occupational data of other countries, they were able to show that it correlated well with self-reported data on how many people across various countries worked from home during the pandemic.
To validate and expand on this analysis, I decided to use GPT-4o to label all job tasks in the O*NET database based on whether they can be performed remotely (see here for my dataset, and here for my methodology). In the O*NET database, a job task is more fundamental than an occupation, as it comprises a specific work activity done within the context of a job, which is often shared between professions. This allows for a more fine-grained analysis of the role of remote work in the modern economy, since automation rarely results in entire occupations becoming obsolete all-at-once. Rather, automation is better described as continuously expanding the set of tasks that can be performed by a machine.
Each job task contains a qualitative description of what is involved in its execution, allowing GPT-4o to identify whether the task can be performed remotely or must be done in person. To provide a sanity check, I manually reviewed a random, small selection of tasks, and verified that the labelling seemed accurate.
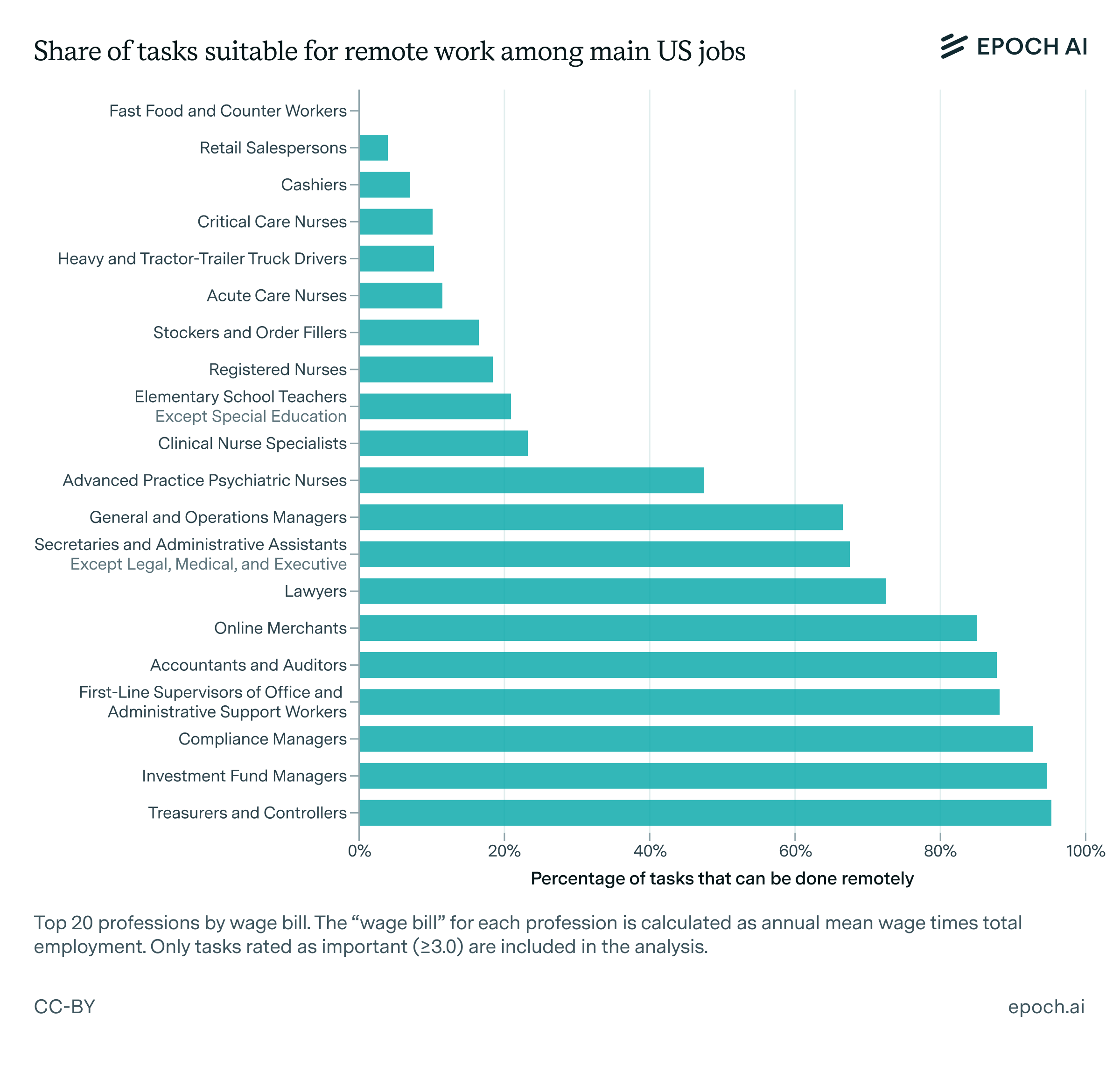
The results of this analysis surprised me, revealing a significant discrepancy compared to Dingel & Neiman’s results. While my classification shows that 34% of job tasks can be performed remotely, only about 13% of occupations have all of their top 5 most important tasks labeled as remote tasks. Even when focusing on only the top 3 most important tasks for each occupation, this figure increases to just 18%.
It is clear that these results are not fully consistent with Dingel & Neiman’s result that 37% of jobs can be performed at home, so what explains the discrepancy? One plausible hypothesis arises from the different purposes motivating the two data collection efforts. In Dingel & Neiman’s case, a major goal was to understand what fractions of jobs can be done at home during a pandemic, whereas in my case, the aim was to better predict which tasks in the economy may soon be automated by AI agents with computer control.
The pandemic disrupted the usual environment and temporarily changed our standards regarding what services can be adequately performed remotely. This shift in standards during the pandemic makes it difficult to compare Dingel & Neiman’s classification with mine. I return to this point when estimating the impact on GDP from automating remote work.
How much would the economy grow if remote work were automated?
Having investigated the fraction of tasks in the economy that could be performed remotely, I now turn to the question of what would happen if these tasks were to be automated. However, to answer this question, we will first need an estimate of the substitutability of remote and non-remote tasks. This is captured in the economics literature by a parameter called the elasticity of substitution, which I briefly explain below.
A brief primer on the elasticity of substitution
Elasticity of substitution (σ) is an economic measure that quantifies the degree to which one task or good can replace another in production or consumption. Understanding this concept is essential because it helps estimate how the share of automated tasks or sectors in the economy will evolve as automation progresses.
To see why the elasticity of substitution matters, let’s consider an example from economic history: agriculture. In many pre-industrial societies, agriculture accounted for the majority of GDP. However, as agricultural processes became increasingly automated, the share of agriculture in the economy shrank dramatically. Today, in the United States, agricultural production represents only about 1-2% of GDP.
This example illustrates a critical point: as automation transforms a sector, the importance of that sector within the broader economy can decline. This is because as productivity increases and output grows, additional gains in efficiency tend to contribute less and less to GDP. As a consequence, automation in these cases will have a muted effect on overall economic growth.
However, this pattern is not universal. Consider the case of textiles during the Industrial Revolution in Great Britain. The introduction of powered looms and spinning machines made textile production far more efficient, and instead of shrinking in importance, the textile industry grew to become a key part of Britain’s economy. In this case, automation led to a significant expansion of the sector’s share in the economy, meaning that automating textiles had an outsized, large impact on overall economic growth.
These contrasting examples – agriculture and textiles – highlight an important question: when remote work becomes increasingly automated, will it follow the pattern of agriculture, shrinking in economic importance as it becomes easier to automate? Or will it resemble textiles, growing in economic importance as automation increases? The answer depends on the elasticity of substitution (σ) between remote work and non-remote work.
More specifically, if σ < 1 (i.e. tasks are not easily substitutable in production), automating remote work will resemble the case of agriculture, with remote labor experiencing a declining share of GDP as it gets automated. Alternatively, if σ > 1 (i.e. tasks can relatively easily be substituted in production), then the share of GDP paid to information work will increase as it becomes automated.
The production function
We now turn to estimating an aggregate production function with a focus on the contribution of remote work to aggregate output. A production function is an economic relationship between inputs and outputs – in this case, a relationship between the number of remote workers, the number of non-remote workers, and overall economic output.
A common functional form employed in macroeconomics to model the productive capacity of the economy is the Constant Elasticity of Substitution (CES) production function. This function takes the following form:
\[ Y = F(R, N) = A[\alpha R^\rho + (1-\alpha) N^\rho]^{\frac{1}{\rho}} \]
Here, Y is the level of production, R is the number of remote workers, N is the number of non-remote workers. \(\alpha\) is a share parameter, and \(\rho\) is the substitution parameter. The parameter A is a scale parameter, often representing “technology”, a productivity multiplier.
As per its name, a CES production function makes the assumption that the elasticity of substitution between factors of production is constant, and does not change with different inputs. The elasticity of substitution is related to the substitution parameter, \(\rho\), specifically via the following relationship:
\[ \sigma = 1/(1 - \rho) \]
With this function, we can calculate the theoretical effect of automating remote work on total production. The idea here is that, if AIs can effectively substitute for humans in remote work and become widely deployed throughout the economy, we can model this transformation as a dramatic increase in the remote work input \(R\). Moreover, economic output will grow even further if some percentage of displaced human remote workers successfully transition to non-remote tasks as AI fills remote positions.
With these modeling assumptions, we can estimate the economic impact of automating remote work by determining the parameters of this production function and then calculating how much total output Y increases when remote work input R is multiplied by a large factor.
Unfortunately, fitting the parameters of a CES production function can be difficult. Traditionally, this is done by fitting the function to cross-sectional data or good long-term time series data, which we lack here. To mitigate this issue, I will discuss two alternative methods that may be used to obtain credible estimates:
- Using the pandemic as a natural experiment to estimate a value for the elasticity of substitution
- Using proxy data to estimate the elasticity of substitution. I examine data for skilled labor vs. unskilled labor, and discuss a pessimistic lower bound.
Using the pandemic to estimate the elasticity of substitution
The COVID-19 pandemic offered a unique natural experiment to understand the effects of automating remote work. During the pandemic, a large fraction of the workforce suddenly switched from performing their tasks in-person to performing their tasks remotely.
If the elasticity of substitution between remote work and non-remote work is low—meaning these two types of tasks function as economic complements—we would expect a severe decline in GDP during the pandemic. This is because complementary tasks cannot easily substitute for one another, so the inability to perform in-person work would create significant bottlenecks and hence disrupt economic activity.
What actually happened during the pandemic surprised many economists. Despite the dramatic shift to remote work and the fact that only a small percentage of the workforce had previously worked from home, GDP declined by much less than many had anticipated. From Q4 2019 to Q2 2020, U.S. GDP fell from approximately $20.9 trillion annualized to $19.1 trillion, representing a contraction of about 9.2%. While this decline was severe by typical standards — compared to most recessions — it was smaller than many, perhaps most, economists had predicted.
In the plot below, we can see the size of the decline in real GDP during 2020 was relatively modest when considering that roughly a third of US workers shifted to remote work.1
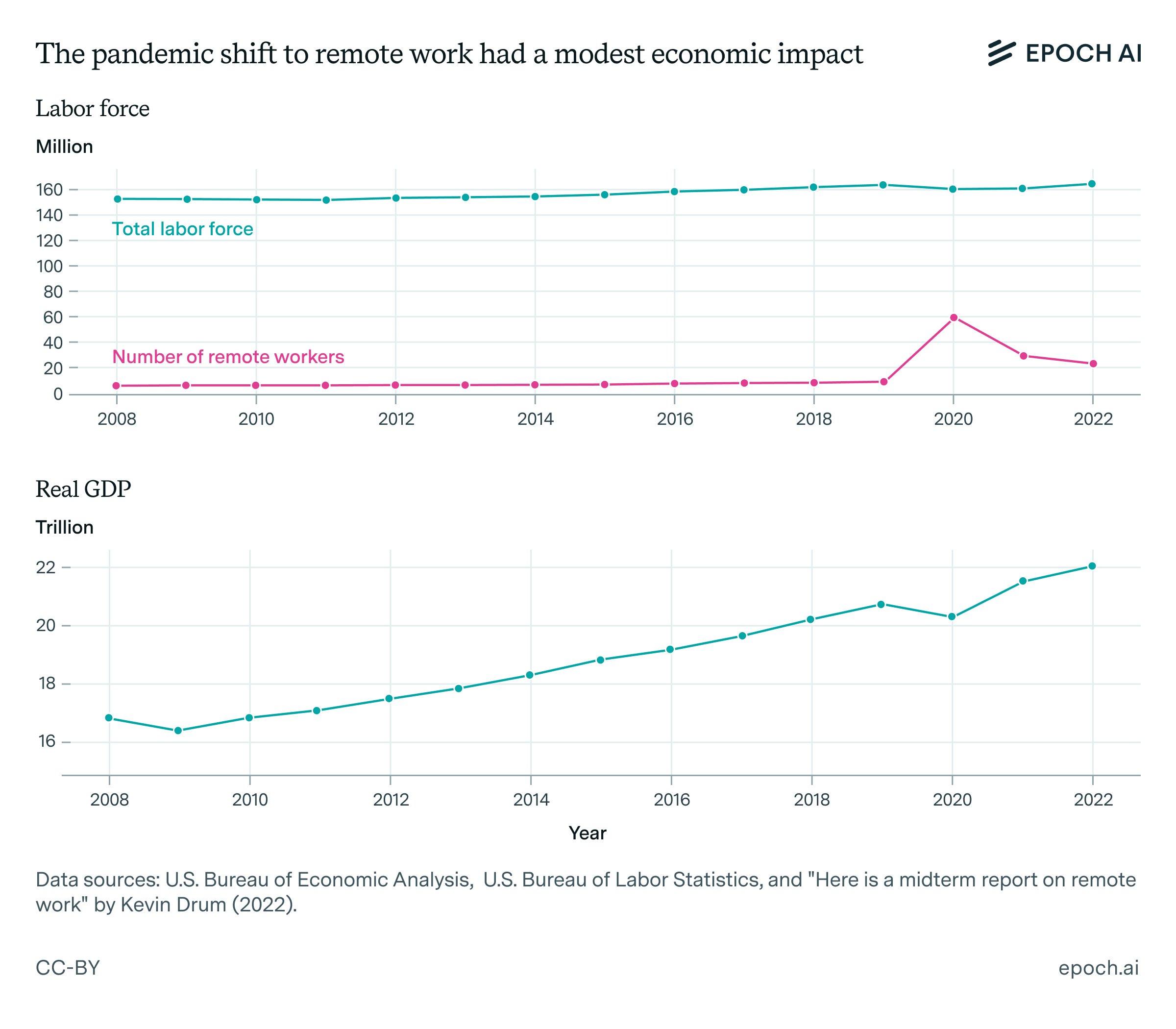
In fact, the size of the decline in real GDP was so small that this decline may have largely simply been attributable to the fact that millions of people exited the labor force entirely at the same time other workers shifted to working remotely. If no one had exited the labor force at all, and instead all that had occurred was that roughly a third of workers shifted to working remotely, the decline in GDP would likely have been significantly smaller, suggesting that the impact of tens of millions of people suddenly deciding to work remotely might have had almost no impact on GDP.
The economy’s ability to adapt smoothly to such an environment without a huge economic contraction means that many in-person tasks may have been much more substitutable with remote tasks than many previously thought. This outcome suggests that the elasticity of substitution between remote work and non-remote work may be much higher than many economists initially assumed. More precisely, this data suggests a large share of tasks in the economy (perhaps more than a third) have a high degree of substitutability with remote tasks, with σ >> 1 for those tasks.
This observation is particularly surprising when considered in the context of how economists have traditionally explained urbanization in the modern world. According to a widely accepted economic theory, people are drawn to major cities because urban jobs are generally more productive and offer higher wages compared to jobs in less densely populated areas. This theory suggests that the productivity advantage of urban jobs comes from the opportunity for workers to interact with many others face-to-face, creating synergies and collaborative benefits that are difficult to replicate in rural or less connected environments.
Based on this theory, one would expect that the abrupt shift to remote work during the pandemic would have caused a dramatic decline in productivity, as workers lost the benefits of in-person interactions. However, the data did not reflect this expectation. Despite the widespread move to remote work, productivity did not decline as sharply as this orthodox theory would predict.
However, it is worth keeping in mind that this interpretation of the Covid era data is not uniformly accepted, and there is an alternative story that could better explain the data. Namely, it’s plausible that the productivity loss from switching to remote work was low simply because the share of tasks that can be done remotely is very high, rather than because the elasticity of substitution between remote and non-remote tasks is high. In this story, during normal economic times, a large share of people work in-person mainly because there is a small productivity boost to working in-person, even though their tasks can inherently be done remotely.
However, even if this alternative interpretation of the data is correct, it shouldn’t change the conclusion that the effect of automating remote work would be very large. This is because the alternative story involves a large share of remote tasks, which simultaneously means that a lot of work could potentially be replaced by digital AI workers. Since these two different interpretations produce the same conclusion, the distinction between them shouldn’t matter much for the purpose of my analysis.
A separate problem with my analysis is that GDP estimates during the Covid era are controversial. As mentioned in a previous section, when people switched to remote work during the pandemic, their outputs changed subtly. For example, doctors began using telehealth, rather than talking to patients inside of a clinic. This may have resulted in lower quality care, and this quality difference may not have been well-captured by the official GDP statistics. Similar effects were seen throughout the entire economy. For this reason, it is worth considering alternative ways to measure σ.
Estimating the elasticity of substitution via proxy
Another way to estimate the elasticity of substitution between remote work and non-remote work is to look at potential proxy variables. This includes published estimates for the elasticity of substitution between different pairs of factors of production.
A plausible proxy is the elasticity of substitution between skilled labor and unskilled labor. This proxy seems appropriate because remote work, by its nature, involves using one’s brain, rather than one’s muscles. Naturally, one can assume that jobs that require college education are particularly likely to be jobs that can be performed remotely. This dichotomy is often captured in the economic literature, at least approximately, by the distinction between skilled and unskilled labor.
One recent meta-analysis reviewed 682 estimates for this parameter across 72 studies. They found that most estimates tended to range from 1 to 3. However, they also found publication bias towards lower estimates, suggesting a true value closer to 4. If a value of 4 is correct, this result aligns relatively well with the analysis in the previous section.
Nonetheless, many people have the intuition that remote work and non-remote work should have large complementarities. For this reason, it is worth considering a conservative lower bound on the elasticity of substitution. Perhaps the most pessimistic proxy I can think of is the case of food vs. non-food goods in the economy. Food is difficult to substitute with other economic goods because human beings need it to survive. If a person were starving, providing them with abundant non-food goods—such as housing, transportation, or refrigeration—would do little to address their immediate need for calories.
However, even in the case of food, the complementarity is not infinite. As food gets cheaper relative to other goods, people do not simply continue to buy the minimum amount of food needed to meet their caloric requirements; instead, they tend to allocate more income toward purchasing higher-quality or more desirable foods. Studies often calibrate their elasticity of substitution between food and non-food goods to be around 0.5. In my view, a value of 0.5 serves as a pessimistic lower bound for elasticity of substitution between remote work and non-remote work.
Putting it all together
My guesses for the elasticity of substitution vary widely, from a pessimistic lower bound of 0.5, to values far greater than 1, as suggested by the data during the Covid era. Since these estimates span a wide range, it is convenient to consider a range of possibilities for the value of this key parameter. Below, I have plotted two scenarios (taken from this notebook), representing different ends of this spectrum. In the pessimistic case, the elasticity of substitution is 0.5, and in the optimistic case, the elasticity of substitution is 10. In both scenarios, I assume the share of work that can be done remotely is initially 34%—in line with my data. I also assume that approximately 50% of displaced human remote workers switch to non-remote tasks after being displaced by digital AI workers.
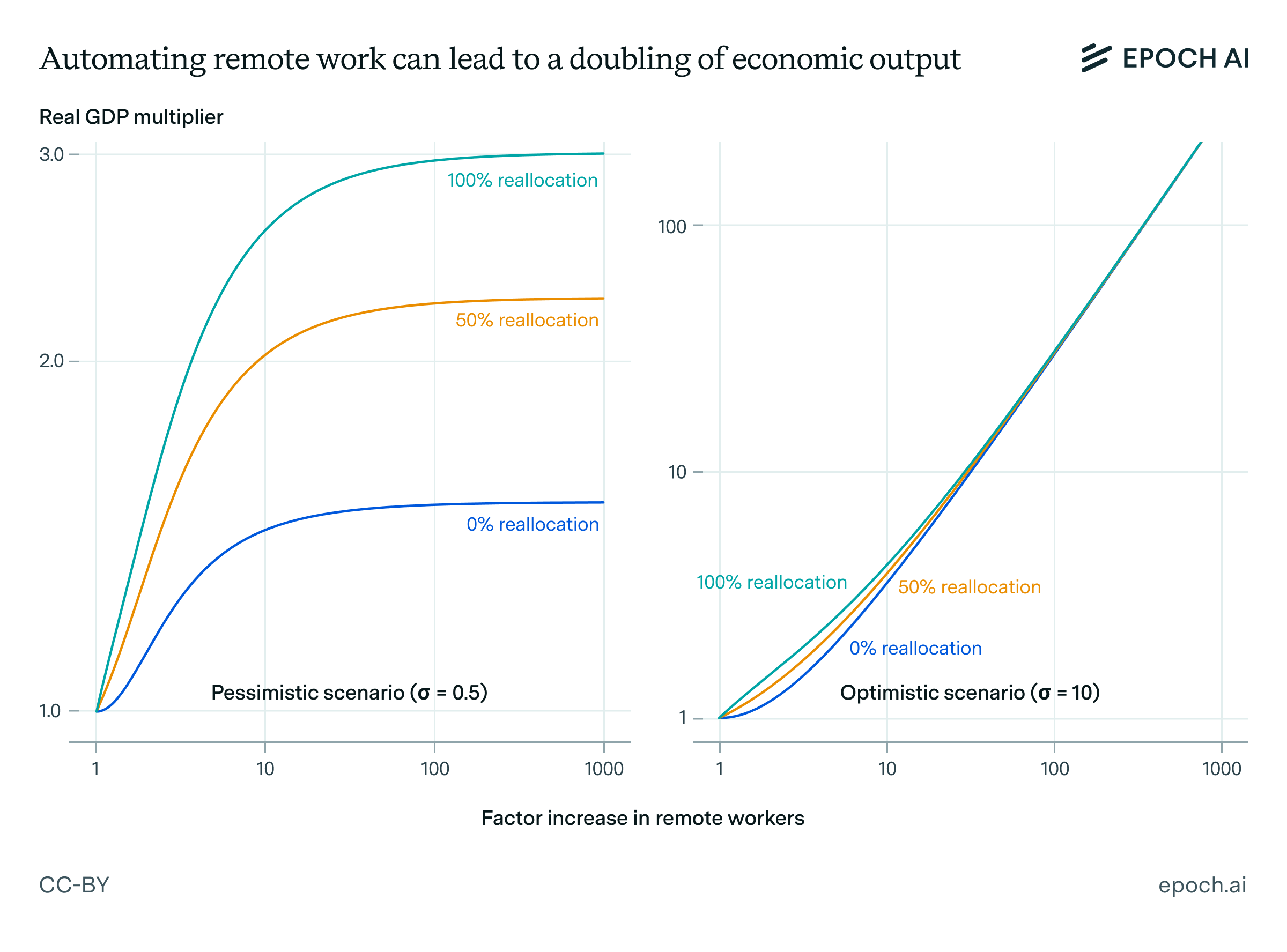
This plot demonstrates that, even under quite pessimistic assumptions, U.S. GDP could still double if remote labor became automated. This growth could be achieved by increasing the number of remote workers in the economy by a factor of 100-1000, which could occur if tens of billions of digital workers were hosted in data centers.2 If such an economic expansion occurs over the course of one decade, this would correspond to an average annual economic growth rate exceeding 7%. This rate could also be pushed higher if it were supplemented with progress in robotics and non-AI sources of economic growth.
However, in my opinion, this pessimistic scenario is too conservative. A value for the elasticity of substitution below 1 seems to contradict both the economic data during Covid, and the proxy estimate for the elasticity of substitution between skilled and unskilled labor. Given this background evidence, I think the effect from fully automating remote work will likely be much larger than a doubling of U.S. GDP.
If the result is closer to the most optimistic scenario, then real GDP would grow by a factor of more than 10 if the number of remote workers were scaled by a factor of 100-1000. In a scenario in which real GDP expands by “merely” a factor of 10 over the course of a decade, then economic growth rates would exceed 25% per year – way beyond anything experienced so far in U.S. history.
Yet even the optimistic scenario rests on some conservative assumptions. In particular, the optimistic scenario relies on an assumption of constant returns to scale, as modeled by the CES production function. Under this assumption, a proportional increase in all inputs leads to an equivalent proportional increase in output. However, historical evidence suggests that the global economy does not exhibit constant returns to scale. Instead, the economy appears to exhibit increasing returns to scale, where increases in inputs, such as population size, yield technological progress, which enables more efficient use of resources.
One way these increasing returns to scale could manifest is through AI systems automating the research and development processes for AI itself. However, this mechanism should not be viewed too narrowly. Increasing returns to scale for the overall economy can also arise from AI automating other forms of scientific discovery and technological innovation, contributing to broader increases in efficiency across various industries. Incorporating these possibilities into a comprehensive model of economic growth presents an important avenue for future research.
Rather than focusing on the exact bounds, the right conclusion to draw here is probably that these effects on economic growth will be enormous. I consider it highly likely that U.S. GDP would more than double as a consequence of remote work becoming fully automated. Indeed, a 10-fold expansion in the size of the U.S. economy seems slightly more likely than not to me. Such a dramatic economic expansion, taking place in the near or medium-term future, would be well beyond mainstream economic forecasts, and would surely be a transformative event in human history.
Notes
-
This data comes from the Bureau of Labor Statistics and Kevin Drum. In the Google Colab notebook for this newsletter issue, I employ this data to fit the parameters of the CES production function as outlined in the previous section. The result I get is an estimate of σ = 12.9. The exact value of σ = 12.9 should be taken with a huge grain of salt, as this model does not appear very robust, and it only takes into account data in a single country since 2008. Rather than thinking about this particular number, it is more important to understand the more basic point that as remote work became dramatically more popular in 2020, the United States only experienced a very modest decline in real GDP. Even if this analysis is wrong in some way, the main takeaway is that the data is broadly consistent with a value for σ much greater than 1, suggesting a high elasticity of substitution. ↩
-
At an inference cost of, say, 10^14 FLOP/s per worker, creating ten billion digital workers that run continuously would require a compute stock capable of performing 10^24 FLOP/s. This would be the equivalent of roughly 250 million H100s performing work in parallel at maximum efficiency. While this is an enormous amount of compute by today’s standards, current projections of chip capacity support the notion that such a scenario could be achieved in the early 2030s. ↩